Exploring Decision Support Systems in Healthcare
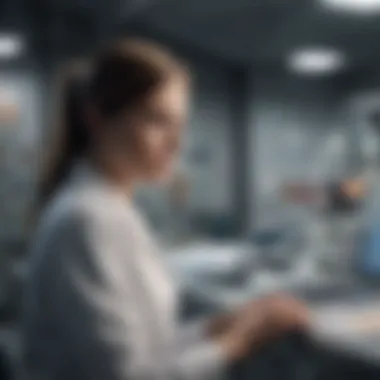
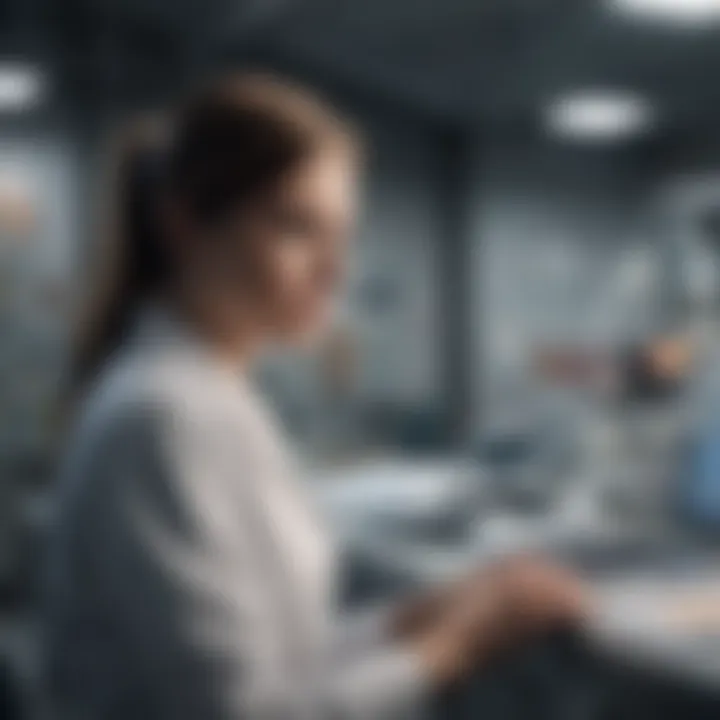
Intro
Decision Support Systems (DSS) have emerged as a critical component in the landscape of healthcare. These systems leverage various technologies to support clinical decision-making, directly influencing patient care. By facilitating access to data and guiding healthcare professionals in making informed choices, DSS can improve the quality of healthcare delivery. As the healthcare industry faces increasing complexity, the integration of DSS offers significant advantages. Through proper implementation, these systems not only streamline processes but also enhance patient outcomes and safety.
Research Highlights
Key Findings
Research in the field of Decision Support Systems has brought to light several important aspects. Studies have demonstrated that DSS can help reduce diagnostic errors. For instance, systems that integrate artificial intelligence can analyze vast amounts of data, identifying patterns that clinicians might miss. Additionally, DSS can leverage clinical guidelines to support treatment decisions. The primary findings suggest that:
- Effective DSS leads to improved consistency in clinical decision-making.
- Systems utilizing real-time data experience higher accuracy in recommendations.
- Integration of DSS in workflows enhances overall organizational efficiency.
Implications and Applications
The advantages of implementing Decision Support Systems in healthcare are manifold. Here are a few key implications:
- Enhanced Patient Outcomes: DSS can lead to better treatment plans and increased compliance with clinical guidelines, ultimately resulting in improved health outcomes.
- Informed Decision-Making: Access to precise and relevant data enables healthcare providers to make timely decisions, which is crucial in scenarios requiring immediate intervention.
- Operational Efficiency: By minimizing the time spent on information retrieval, DSS can optimize the workflow of healthcare facilities, allowing professionals to focus on patient care.
According to various studies, healthcare facilities using Decision Support Systems report a substantial increase in the accuracy of diagnoses and treatment recommendations.
Methodology Overview
Research Design
The exploration of Decision Support Systems in healthcare involves diverse methodologies. A mixed-methods approach is often employed, combining quantitative and qualitative analysis. This includes case studies and surveys to gather data on DSS effectiveness.
Experimental Procedures
Research typically examines the following components:
- System Implementation: Evaluating how DSS are integrated into existing healthcare settings.
- Functionality Assessment: Analyzing how these systems interact with other clinical tools and databases.
- Outcome Measurement: Studying the impact of DSS on patient outcomes through metrics such as treatment effectiveness and patient satisfaction.
Through these procedures, it is possible to gauge the overall influence of DSS on healthcare and inform future developments in this essential area.
Prelims to Decision Support Systems
Decision Support Systems (DSS) play a critical role in modern healthcare, shaping how decisions are made by practitioners. The significance of these systems lies in their ability to analyze complex data and assist in making informed choices regarding patient care. By acting as a bridge between vast amounts of healthcare data and the healthcare provider's judgment, DSS enhance the clinical decision-making process. This section provides a closer look at defining DSS and their importance in healthcare.
Defining Decision Support Systems
Decision Support Systems refer to a variety of tools and technologies designed to help healthcare professionals make explicit decisions based on data analysis. A DSS can take many forms, from simple algorithms that guide treatment to complex software that aggregates patient history, lab results, and current guidelines. The core function of a DSS is to facilitate understanding and proper use of information, reducing uncertainties in clinical decision-making.
These systems analyze data from various sources such as electronic health records (EHRs), diagnostic tests, and patient demographics. By processing this information, DSS present actionable insights tailored to specific clinical scenarios.
Importance in Healthcare
The importance of Decision Support Systems in healthcare cannot be overstated. With the increasing complexity of medical data and rapid advancements in treatment protocols, clinicians face significant challenges. DSS help overcome several issues:
- Improved Efficiency: DSS reduce the time spent on data interpretation and streamline workflows, allowing healthcare providers to devote more time to patient care.
- Enhanced Accuracy: By cross-referencing patient data with comprehensive databases, DSS minimize errors that can arise from human judgment alone. This is particularly vital in urgent care settings where every decision counts.
- Personalized Medicine: DSS enable tailored treatment plans based on individual patient data and preferences, enhancing the relevance of care provided.
"Decision Support Systems are vital for navigating the complexities of modern healthcare and ensuring optimal patient outcomes."
As healthcare evolves, the necessity of integrating robust Decision Support Systems becomes increasingly evident. They stand as essential tools that empower healthcare professionals, reduce the potential for error, and ultimately lead to better patient care and improved health outcomes.
Components of Decision Support Systems
The effectiveness of Decision Support Systems (DSS) resides in their core components, each contributing to the overall capability and usability of these systems in healthcare. Understanding these components—data management, inference engine, and user interface—sheds light on how DSS facilitate informed decision-making processes. Each component plays a distinct role and, together, they form a cohesive unit that enhances operational efficiency and outcomes in medical environments.
Data Management
Data management is the foundation of any Decision Support System. It involves the systematic collection, storage, and retrieval of healthcare data. These data can come from multiple sources, including electronic health records, laboratory results, and patient monitoring systems. Therefore, the accuracy and timeliness of data are crucial. If the data is flawed or outdated, the resulting analysis and recommendations will also be unreliable.
In this context, proper data management allows healthcare providers to draw insights that lead to improved patient care. For instance, an organized data management system can help identify trends in patient conditions over time. This not only aids in patient monitoring but also supports preventive measures. Ultimately, effective data management ensures that the right information is available at the right time, which is essential for making informed clinical decisions.
Inference Engine
The inference engine serves as the analytical core of a Decision Support System. It processes the data provided by data management and applies predefined rules or algorithms to generate insights. This component evaluates various scenarios, weighing different factors, and produces recommendations based on its analysis.
A robust inference engine can enhance predictive capabilities, helping clinicians identify potential health risks before they escalate. For example, a well-designed inference engine may analyze patient data to predict the likelihood of readmission, allowing healthcare providers to implement intervention strategies proactively.
The significance of an inference engine is that it translates raw data into actionable knowledge, bridging the gap between mere information and practical application in clinical settings.
User Interface
User interface (UI) design is a critical element that influences the effectiveness of a Decision Support System. A well-structured UI allows healthcare professionals to interact with the DSS intuitively and efficiently. It should present complex data in an easily digestible format, minimizing cognitive load.
An effective UI also takes user feedback into account, allowing customization and adaptability. This enhances user satisfaction and ensures that the system meets the specific needs of different healthcare providers. In practice, a user-friendly interface can minimize training time, facilitating quicker adoption of the system.
Moreover, the UI should enable seamless integration of the DSS with existing workflows. Healthcare professionals are often pressed for time; therefore, a system that blends seamlessly into their routines increases the likelihood of its usage.
Types of Decision Support Systems
Understanding the different types of Decision Support Systems (DSS) is crucial for grasping their role in healthcare. Each type has its specific functionality and aimed outcomes, serving unique needs of healthcare professionals and organizations. By distinguishing these types, one can appreciate the tailored approaches these systems offer to tackle various clinical challenges. This differentiation also helps in recognizing how each type contributes to enhanced patient care and informed decision-making.
Clinical Decision Support Systems (CDSS)
Clinical Decision Support Systems (CDSS) are among the most prominent types of DSS in healthcare settings. These systems provide healthcare professionals with knowledge and specific patient information intelligently filtered and presented at appropriate times to enhance patient care.
CDSS utilize patient data alongside clinical guidelines and medical knowledge, enabling practitioners to make evidence-based decisions. Key functionalities often include:
- Alerts and reminders: Automatic notifications for potential issues such as drug interactions or allergies.
- Clinical guidelines: Recommendations based on established protocols, assisting in implementing best practices.
- Diagnostic support: Tools that help clinicians interpret patient data and diagnostic results effectively.
The integration of CDSS into daily clinical practice can lead to several benefits, including improved accuracy in diagnosis, timely interventions, and ultimately better patient outcomes. The importance of CDSS cannot be understated, as they bridge the gap between vast medical knowledge and the practical application needed at the point of care.
Executive Information Systems
Executive Information Systems (EIS) serve a markedly different purpose compared to CDSS. These systems are designed for high-level decision-makers in healthcare organizations, providing relevant data to inform strategic planning and management decisions. EIS consolidate patient information, financial data, and operational statistics into a single dashboard view, which can be critical for making informed organizational choices.
Key elements of EIS include:
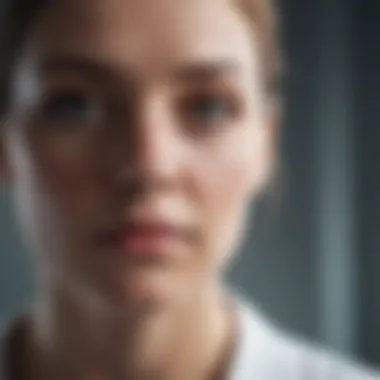
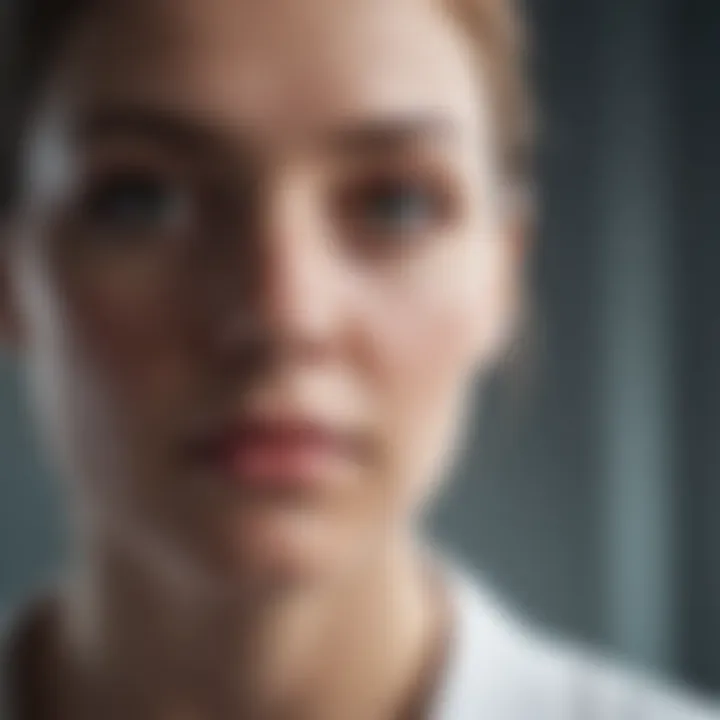
- Data Visualization Tools: Graphs, charts, and dashboards that present complex data in an easily digestible format.
- Performance Metrics: Overview of key performance indicators relevant to the healthcare organization’s objectives.
- Scenario Analysis: Tools to simulate various scenarios to understand potential outcomes of strategic decisions.
By utilizing EIS, healthcare executives can monitor trends, optimize resources, and strategize for future improvements. The distinct roles these systems play highlight the diverse applications of DSS, significantly benefiting both patient care and organizational efficiency.
In summary, understanding the types of Decision Support Systems is essential for appreciation their impact on decision-making processes within healthcare. By addressing both clinical and executive needs, DSS elevate the standard of care and operational excellence.
Example of a Clinical Decision Support System
In healthcare, clinical decision support systems (CDSS) play a crucial role in assisting medical professionals. Their main objective is to provide timely and relevant information that aids in clinical decision-making. These systems harness a variety of data sources, allowing practitioners to make informed choices tailored to each patient's unique conditions. A well-implemented CDSS can significantly enhance the quality of care provided and help in managing healthcare resources more effectively.
Interpreting Diagnostic Data
Interpreting diagnostic data is a key function of clinical decision support systems. A CDSS integrates patient data such as lab results, imaging studies, and clinical history, then uses it to present clear insights to physicians. This capability aids in identifying crucial patterns and potential health issues faster than traditional methods.
The system can also reduce errors in diagnosis, as it can compare the incoming data against established clinical guidelines. For instance, a CDSS might alert a clinician to abnormal lab results that align with a specific disease pattern. This highlight can prompt further investigation, potentially leading to timely intervention.
Moreover, by utilizing advanced algorithms, CDSS can analyze massive datasets, providing predictive insights that help anticipate future health complications. This feature is especially valuable for managing chronic diseases, where early detection can prevent severe outcomes.
Treatment Recommendations
The treatment recommendations generated by a clinical decision support system contribute significantly to improving patient outcomes. Based on the interpreted diagnostic data, these recommendations guide healthcare providers in choosing the most appropriate course of action.
For example, in cases of pneumonia, a CDSS can recommend specific antibiotics based on the identified bacterial strain and the patient's medical history. This targeted approach not only enhances treatment effectiveness but also minimizes the risk of antibiotic resistance, which is a growing concern in modern medicine.
Furthermore, CDSS can also consider patient preferences and clinical guidelines simultaneously. This ensures that recommended treatments align with the latest evidence-based practices while catering to the individual's values. It not only aids clinicians but also empowers patients in their treatment journey.
"Clinical decision support systems are redefining the baseline for patient care quality and operational efficiency in healthcare settings."
By encompassing these processes, clinical decision support systems provide a comprehensive strategy for healthcare providers, enhancing decision-making at every stage of patient care.
Technological Frameworks in DSS
Technological frameworks are the backbone of Decision Support Systems (DSS) in healthcare. These frameworks integrate sophisticated technology to facilitate efficient decision-making processes. By utilizing advanced software and computational technologies, DSS can analyze vast amounts of data quickly and effectively, providing critical insights to healthcare professionals. Such systems enhance not only the speed but also the reliability of decision-making in clinical environments.
The integration of technology in DSS comes with numerous benefits. For one, it supports improved clinical outcomes through better-informed decisions. Furthermore, these frameworks enable clinicians to access real-time data, allowing for prompt responses to patient needs. With the increasing complexity of healthcare data, a robust technological framework is indispensable for navigating this landscape.
Machine Learning Applications
Machine learning plays a significant role in the evolution of DSS. By leveraging algorithms that learn from data, healthcare providers can predict patient outcomes more accurately. Machine learning applications in DSS can identify patterns in patient data, which is essential for personalized treatment plans. For instance, algorithms can analyze historical patient information to flag potential health issues before they escalate.
The use of machine learning in DSS enhances diagnostic accuracy and treatment effectiveness. This can lead to reduced hospital stays and improved patient satisfaction rates. Moreover, as systems learn from new data continuously, their predictive capabilities improve over time, which makes them an invaluable asset in clinical settings.
Predictive Analytics
Predictive analytics is another critical component of technological frameworks in DSS. This process involves using statistical techniques and algorithms to analyze historical and current data. The goal is to forecast future events based on identified trends and patterns. In healthcare, predictive analytics can be a game-changer for patient care.
By implementing predictive analytics, healthcare systems can foresee potential outbreaks of diseases, understand patient risk factors, and allocate resources more efficiently. For example, hospitals can predict patient admission rates during flu season, allowing them to prepare staff and resources accordingly.
"Predictive analytics could transform how healthcare providers anticipate the needs of their patients, ultimately reshaping patient care delivery."
Key aspects of predictive analytics include:
- Risk Assessment: Identifying patients who may need preventive care.
- Resource Management: Ensuring the availability of necessary supplies and staff.
- Treatment Optimization: Customizing treatment plans based on predictive models for better health outcomes.
The fusion of machine learning and predictive analytics within DSS forms a powerful technological framework that genuinely enhances healthcare delivery. These frameworks not only improve operational efficiency but also elevate the standard of patient care. As technology advances, the potential for more integrated and sophisticated decision support systems will continue to grow, ultimately leading to better healthcare outcomes.
Impact on Healthcare Outcomes
The integration of Decision Support Systems (DSS) in healthcare has significant implications for healthcare outcomes. These systems are designed to enhance the process of decision-making for clinicians and other healthcare professionals. They provide clinical knowledge and patient-specific information to support care decisions. As the demands on the healthcare system continue to grow, it becomes crucial to understand how DSS not only helps in operational efficiency but also markedly influences patient outcomes.
Enhanced Patient Safety
One of the primary benefits of Decision Support Systems is their role in enhancing patient safety. By offering guidelines and evidence-based recommendations, DSS helps healthcare providers avoid mistakes during diagnosis and treatment. For instance, these systems can alert clinicians to potential drug interactions, allergies, or inappropriate dosages.
According to a study published by the Agency for Healthcare Research and Quality, implementing Clinical Decision Support Systems led to a notable decrease in adverse drug events by about 50%. Enhancements in patient safety not only contribute to improved health outcomes but also foster greater trust between patients and healthcare providers.
"DSS fundamentally shifts the paradigm of healthcare from reactive measures to preventive strategies, significantly cutting down risks associated with patient care."
Reduction of Medical Errors
Medical errors are a significant concern within healthcare systems, with studies estimating that they contribute to thousands of deaths each year. The effective utilization of Decision Support Systems is promising in reducing such errors through systematic reviews of clinical data. These systems enable clinicians to access critical information swiftly, thus minimizing the likelihood of misdiagnosis or incorrect treatment plans.
Some benefits include:
- Real-time Alerts: DSS can provide timely warnings for clinicians regarding potential safety issues, thus allowing for immediate intervention.
- Data Standardization: Structured data input reduces variability and errors associated with manual data entry.
- Improved Communication: DSS fosters better communication among healthcare teams, ensuring that everyone is aligned on patient care informed by accurate data analysis.
The evidence suggests that hospitals employing robust DSS frameworks have seen a marked decrease in the incidence of medical errors. This not only streamlines workflow but also enhances overall patient care quality.
Case Studies of Decision Support Systems
Case studies play a crucial role in understanding the real-world applications and effectiveness of Decision Support Systems (DSS) in healthcare. By examining specific instances where these systems have been implemented, we can draw important conclusions about their functionality and impacts. Case studies help to illuminate best practices, reveal challenges faced during implementation, and provide evidence of the benefits DSS can offer.
In exploring case studies of DSS, it is essential to focus on key elements such as:
- Real-life examples that show successful outcomes
- Specific benefits achieved through the use of DSS
- Considerations regarding the integration of DSS into existing healthcare environments
- Lessons learned from each case study that can aid future implementations
By delving into these aspects, this section provides valuable insights into how DSS can transform patient care and decision-making processes.
Case Study One: Predictive Risk Modeling
Predictive risk modeling systems stand out as one significant example of DSS in healthcare. These systems are designed to evaluate vast amounts of clinical data to predict potential adverse outcomes, such as hospital readmissions or complications following procedures. Through sophisticated algorithms and statistical methods, predictive risk models can identify patients who may require additional monitoring or intervention.
A notable case involves the use of predictive modeling at a major hospital network. The institution implemented a system that analyzed patient data, including demographics, medical history, and prior hospitalizations. The model successfully identified high-risk patients, enabling clinicians to tailor interventions more effectively. As a result, the hospital noted a substantial reduction in readmission rates by nearly 20% within one year of implementation.
Benefits from such systems include:
- Improved patient stratification for preventive care
- Enhanced allocation of resources in high-risk situations
- Greater clinician confidence in decision-making through data support
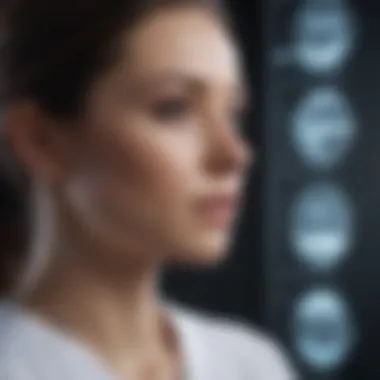
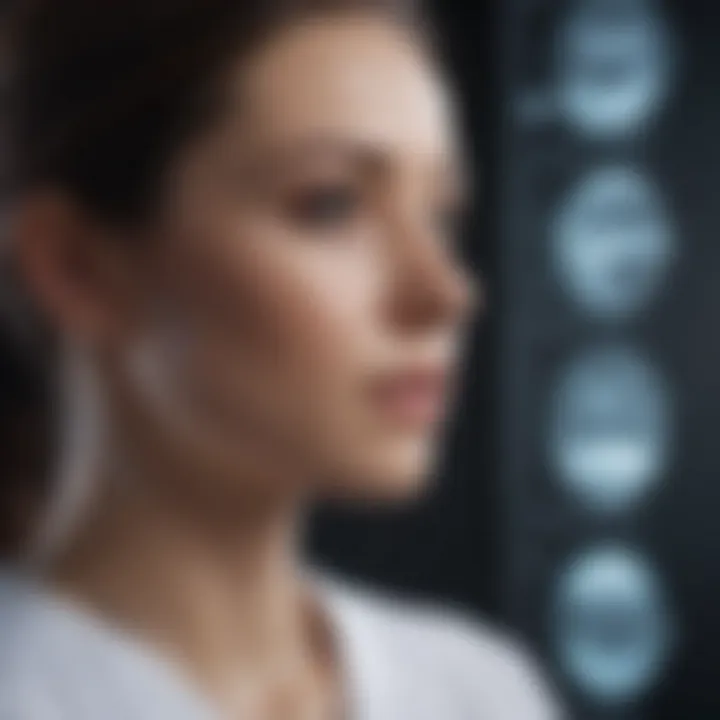
The case underscores the growing importance of data-driven approaches in identifying risks, fostering proactive measures in patient care.
Case Study Two: Personalized Treatment Plans
Personalized treatment plans are another critical application of DSS in healthcare. These systems utilize patient-specific data—such as genetic information, lifestyle factors, and clinical histories—to recommend tailored treatment options. By focusing on individualized care, these systems aim to optimize outcomes based on the unique profiles of patients.
An illustrative case can be drawn from a leading cancer treatment center that adopted a personalized treatment planning DSS. The center integrated genomic data analysis to guide oncologists in selecting therapies that matched the genetic makeup of each patient’s tumor. This precision medicine approach showed remarkable results, with a noted increase in treatment success and a corresponding decrease in adverse drug reactions.
Key aspects of this case include:
- Real-time integration of patient data into treatment planning
- Enhanced communication between patients and healthcare providers regarding treatment options
- Continuous feedback mechanism to adapt treatments as necessary
Overall, the findings from these case studies illustrate the transformative potential of Decision Support Systems in healthcare settings. They not only enhance clinical decision-making but also contribute significantly to improved patient outcomes.
Challenges in Implementing DSS
Implementing Decision Support Systems (DSS) in healthcare is complex and can present numerous challenges. Understanding these challenges is crucial for successful integration and utilization of these systems. They not only affect the performance of DSS but also influence the overall effectiveness of healthcare delivery. Identifying and addressing these challenges can lead to improved patient outcomes and enhanced operational efficiency.
Data Quality Issues
Data quality is a significant concern when deploying DSS in healthcare settings. High-quality data is essential for accurate decision-making. Without reliable data, systems can mislead healthcare professionals, negatively impacting patient care. Issues such as incomplete data, inaccurate entries, or outdated information can severely undermine the system's capabilities.
To overcome data quality issues, healthcare facilities can adopt several strategies:
- Data Validation: Utilizing automated checks to ensure that incoming data meets predefined criteria. This helps to maintain accuracy and completeness.
- Regular Audits: Conducting audits of the data on a periodic basis to identify and rectify errors promptly.
- Staff Training: Ensuring that healthcare personnel understand the importance of data quality and are trained to input and manage data effectively.
These strategies contribute to enhancing data integrity, which is fundamental for the DSS to function optimally.
Integration with Existing Systems
Integrating DSS with existing healthcare systems is another critical challenge. Many healthcare facilities rely on multiple disconnected systems. This siloed approach can limit the sharing of information, leading to inefficiencies and potential errors in patient care. A well-integrated system can provide a comprehensive view of patient history, aiding in improved decision-making.
To achieve smooth integration, several considerations must be taken into account:
- Compatibility: Evaluating whether the new DSS can communicate effectively with existing Electronic Health Records (EHR) and other healthcare technologies.
- Interoperability Standards: Using recognized interoperability frameworks can facilitate seamless communication between systems.
- User Adoption: Engaging staff in the integration process to alleviate concerns and promote acceptance of the new system.
Success in integrating DSS with current systems can streamline workflows, minimize disruptions, and enhance overall healthcare delivery.
"The success of a Decision Support System heavily relies on the quality of data it utilizes and the ability to integrate with existing workflows."
Future Trends in Decision Support Systems
The landscape of healthcare is continuously evolving, and the future trends in Decision Support Systems (DSS) reflect the need for more effective tools to enhance decision-making processes. Understanding these trends is essential. It not only provides insights into the direction of healthcare technology but also highlights innovative strategies to improve patient care. Various elements such as advances in artificial intelligence and the integration of telemedicine are at the forefront of these trends, leading to more personalized and efficient healthcare solutions.
Advancements in Artificial Intelligence
Artificial Intelligence (AI) is reshaping the functionality of DSS. The incorporation of AI technologies allows systems to analyze large datasets rapidly and accurately. Machines can learn from previous inputs to enhance their predictive capabilities. This results in more reliable recommendations for healthcare professionals. Furthermore, AI algorithms can identify patterns that human analysts might overlook.
These advancements in AI improve clinical decision-making in various ways:
- Predictive Analytics: AI models can predict patient outcomes based on historical data. This provides clinicians with foresight when managing care plans.
- Natural Language Processing: AI can process unstructured data such as medical notes. This helps in extracting valuable insights which can enhance decision support functionalities.
- Personalization: AI aids in tailoring treatment recommendations specific to a patient's unique health history and demographics.
The integration of AI into DSS is not without challenges, such as data privacy issues and the need for continuous monitoring of algorithms. However, the potential benefits strongly support its implementation in modern healthcare settings.
Telemedicine and DSS
Telemedicine is rapidly gaining traction as an essential component of healthcare delivery. Integrating DSS with telemedicine platforms presents a new paradigm in how care is provided. This integration allows healthcare providers to make informed decisions even when they are not physically present with the patient.
Some key benefits of this trend include:
- Improved Accessibility: DSS integrated with telemedicine can provide remote patients with recommendations and resources without needing in-person visits.
- Real-time data: Through telemedicine, clinicians can access real-time data from patients, allowing for quick decision-making during remote consultations.
- Efficiency: Combining DSS with telemedicine can streamline workflows, allowing healthcare professionals to focus on delivering care instead of manual data retrieval and analysis.
In summary, the future trends in Decision Support Systems signify a dynamic shift towards more technologically advanced solutions. Both advancements in Artificial Intelligence and the growth of telemedicine will shape how healthcare providers approach patient care in the coming years.
Regulations and Standards for DSS in Healthcare
In the complex and evolving landscape of healthcare, regulations and standards for Decision Support Systems (DSS) are crucial for ensuring their effective implementation and utilization. As these systems play an increasing role in clinical decisions, understanding the regulatory framework becomes essential for healthcare providers, developers, and policymakers. This section will cover two primary aspects: compliance with healthcare regulations and data privacy considerations.
Compliance with Healthcare Regulations
Compliance with healthcare regulations is not merely a requirement; it serves to enhance the safety and efficacy of Decision Support Systems within clinical environments. Various bodies establish regulations aimed at safeguarding patient information and ensuring the quality of care delivered. In the United States, the Health Insurance Portability and Accountability Act (HIPAA) sets strict guidelines on how patient data must be managed. Compliance with such regulations necessitates rigorous data security protocols and continuous auditing of data handling practices.
Healthcare organizations must stay updated on any changes in regulations to avoid potential penalties or legal ramifications. This attention to compliance also builds trust among patients, as they are more likely to engage with systems that prioritize their privacy and well-being.
For DSS to be effective, they must conform to clinical guidelines and best practices outlined by relevant authorities, such as the National Institute for Health and Care Excellence (NICE). Incorporating these standards helps bridge the gap between clinical decision-making and regulatory requirements, fostering a culture of accountability in healthcare delivery.
Data Privacy Considerations
Data privacy consideration is a cornerstone of successful Decision Support Systems. As these systems often interact with sensitive patient information, the potential for data breaches creates considerable risk. Ensuring data privacy not only adheres to legal standards but also reflects ethical obligations to protect patient confidentiality.
An effective approach to data privacy involves employing strong encryption methods, access controls, and audit trails. Healthcare providers must establish protocols that determine who can access data and under what circumstances. This becomes particularly important in the context of integrating DSS into broader healthcare systems, where vulnerabilities may arise from system interoperability.
Moreover, educating healthcare professionals about data privacy is essential. Awareness drives responsible behavior in handling patient information and using DSS. Organizations can implement regular training modules to keep staff updated on best practices.
In summary, regulations and standards for DSS in healthcare ensure that these systems contribute positively to patient care while safeguarding privacy and security. This commitment to compliance and privacy not only fosters confidence among patients but also enhances the overall quality of healthcare services provided.
User Training for Effective DSS Utilization
User training for effective Decision Support Systems (DSS) utilization is crucial in maximizing the benefits these systems bring to the healthcare field. As healthcare evolves, the integration of advanced technology such as DSS becomes more prevalent. However, its success largely depends on the competency of the users. The knowledge and skill of clinicians and administrators in utilizing these systems directly correlate with the accuracy of the decisions made.
Poorly trained users can lead to misinterpretations and, ultimately, adverse patient outcomes. Therefore, comprehensive training programs focusing on the practical application of DSS can help bridge this gap. Users must be familiar with the system's interface and capabilities to leverage its full potential. Some benefits of effective user training include:
- Improved Decision-Making: When users understand the DSS well, they can make informed decisions quickly.
- Increased Efficiency: Well-trained personnel can navigate the system swiftly, minimizing delays in patient care.
- Enhanced Patient Safety: Thorough knowledge of the system helps to prevent errors and lapses that could jeopardize patient safety.
In implementing training, one must consider various aspects, such as the scheduling, content of the modules, and ongoing support post-training.
Training Modules for Clinicians
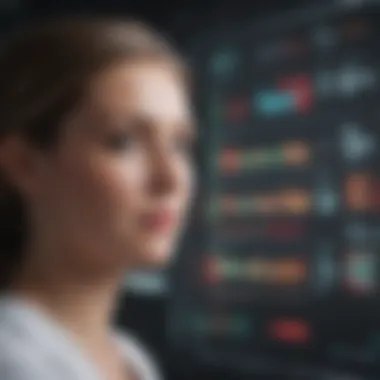
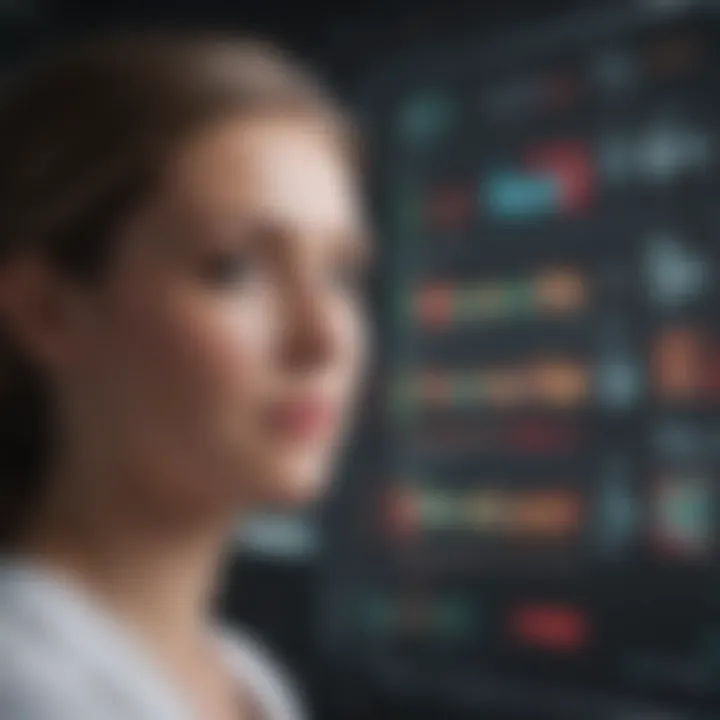
Training modules for clinicians should be designed with a clear focus on clinical relevance. These modules must address both theoretical knowledge and practical skills necessary for effective DSS usage. Key elements include:
- System Overview: This should cover the general functionality of the DSS, including its components and data input methods.
- Clinical Scenarios: Modules should incorporate realistic clinical scenarios that emphasize the decision support facets relevant to daily practices.
- Hands-On Training: Providing clinicians the opportunity to practice using the DSS in a controlled environment ensures comfort when employing it in actual patient care settings.
- Assessment and Feedback: Evaluate the training with assessments, and offer feedback. This allows continuous improvement and identifies areas needing reinforcement.
Such training modules can significantly enhance user understanding and decrease the resistance to adopting these systems.
Continuous Education on System Updates
Continuous education on system updates is another pivotal aspect of user training for DSS. The rapid pace of technology means that updates and new features are common. Keeping clinicians informed enables them to adapt quickly and effectively. It is vital not only to train on initial system deployment but also to instill a culture of lifelong learning. This can be done through:
- Regular Workshops: Hosting periodic workshops can address updates and new functionalities in the DSS.
- Online Resources: Providing easy access to online tutorials and knowledge bases can help clinicians stay informed about changes.
- Feedback Sessions: Encourage users to provide input on system functionality before and after updates. This ensures that clinician needs are met and fosters engagement.
Continuous education fosters an environment where clinicians feel confident in utilizing the most current tools available, resulting in better patient outcomes and system usability.
In summary, user training is not a one-time event but rather an ongoing process that is essential for harnessing the full potential of Decision Support Systems in healthcare.
Patient Involvement in Decision Support Systems
Patient involvement in Decision Support Systems (DSS) is a critical aspect that enhances the overall effectiveness of healthcare delivery. When patients engage with DSS, it empowers them to take an active role in their own treatment processes. This involvement leads to better-informed decisions, resulting in improved health outcomes and patient satisfaction. The integration of patient perspectives into DSS can also lead to more tailored treatment plans that align with individual preferences and values.
Effective patient involvement in DSS requires thoughtful consideration of various elements, such as accessibility of information and shared decision-making. Patients should be able to access relevant health data and understand it easily. This may involve creating user-friendly interfaces where patients can view their medical history, treatment options, and potential outcomes. The goal is to make information more digestible, allowing patients to contribute meaningfully to discussions regarding their care.
Moreover, the benefits of patient involvement go beyond personal decision-making. Engaging patients in the development and implementation of DSS can lead to systems that are more responsive to their needs. When patients provide feedback on their experiences, it can influence the design and functionality of these systems, enhancing usability. Ultimately, this collaborative approach fosters a sense of ownership among patients over their health journey.
Engagement in Treatment Decisions
Engagement in treatment decisions is central to the functionality of DSS. It reflects a shift from a paternalistic model of healthcare to a more participatory approach. Patients who are engaged in the decision-making process tend to be more satisfied with their care. They feel valued and understood, which can enhance compliance with treatment plans. Engaging patients also encourages them to express their preferences and concerns, ensuring that their values are considered in the decision-making process.
To facilitate engagement, healthcare providers can use various strategies. One effective method is shared decision-making, which involves discussions between patients and providers about the possible options, outcomes, and trade-offs of treatments. It requires both parties to share information openly, creating an environment where patients can ask questions and voice their feelings. This method not only improves the patient’s understanding of their treatment options but also increases their commitment to the chosen plan.
Feedback Mechanisms in DSS
Feedback mechanisms are essential for continuous improvement in DSS. These systems must include features that allow patients to provide insights into their experiences with the DSS. Such feedback can highlight the areas needing enhancement, whether in the system's interface, the relevance of information, or the integration of patient-centered features. Understanding how patients use DSS can inform future developments, leading to more effective systems.
Additionally, feedback can take various forms, such as surveys, user reviews, or direct input during consultations. It is vital to create a culture where patients feel comfortable sharing their perspectives. This openness ensures that real-world experiences inform system updates, enhancing the relevance and efficiency of DSS over time.
Evaluating the Effectiveness of DSS
Evaluating the effectiveness of Decision Support Systems (DSS) is crucial for ensuring that these tools actually improve healthcare outcomes. A systematic evaluation helps healthcare providers understand the impact of DSS on clinical practices, patient safety, and overall operational efficiency. It is important to determine if the DSS delivers the expected benefits and to identify areas for improvement.
The evaluation process involves looking at multiple aspects. Firstly, it assesses whether the system meets the initial goals set by the organization. This involves reviewing the functionality of the system in real-world scenarios and comparing it to the intended outcomes. In addition, examining user satisfaction presents insights into the usability of the system. More satisfied users are likely to utilize the system more effectively.
Furthermore, the effectiveness of DSS cannot be only measured through user satisfaction. Evaluations must also consider tangible impacts on patient health and safety. This includes monitoring if the DSS leads to fewer errors in diagnosis and treatment decisions. Therefore, a thorough evaluation addresses both the technical and clinical dimensions of the system's performance.
Key Performance Indicators
Key Performance Indicators (KPIs) are vital in measuring the success of Decision Support Systems in healthcare. These metrics provide quantitative data that can illustrate the system's effectiveness. Common KPIs include:
- User Adoption Rate: Measures how many healthcare providers are using the system.
- Time Savings: Assesses the reduction in time spent on decision-making processes due to the use of DSS.
- Accuracy of Recommendations: Evaluates how frequently the system's suggestions align with actual best practices or outcomes.
- Impact on Clinical Outcomes: This can encompass metrics like reduced readmission rates, length of hospital stays, and overall patient satisfaction.
Each of these indicators provides valuable feedback on the DSS's performance. By systematically tracking these KPIs, healthcare organizations can gauge the overall utility of DSS and adjust accordingly for maximum effectiveness.
Patient Outcome Metrics
Patient Outcome Metrics are integral to understanding the impact of Decision Support Systems on healthcare delivery. Evaluating these metrics allows stakeholders to quantify improvements in patient health and safety. Key metrics include:
- Mortality Rates: Changes in patient survival rates post-implementation of a DSS.
- Re-admission Rates: Frequency of patients returning to the hospital due to unresolved health issues, which should ideally decrease.
- Patient Satisfaction Surveys: Direct feedback from patients regarding their experiences and perceived quality of care.
- Error Rates: Tracking medication errors or misdiagnoses can highlight improvements driven by the DSS.
Evaluating these metrics should not merely be a statistical exercise. It requires a comprehensive approach, considering socio-economic factors and healthcare disparities that might influence outcomes. Understanding how DSS contributes to overall patient health not only validates the system's value but also promotes continuous improvement in healthcare practices.
Evaluating DSS is essential not just to validate their effectiveness but also to refine their functionality for better patient outcomes.
Cost Implications of Decision Support Systems
In today’s healthcare landscape, understanding the cost implications of Decision Support Systems (DSS) is crucial for both providers and administrators. The financial aspects of adopting and implementing these systems can significantly affect their adoption. It is important to analyze both initial costs and long-term benefits to better grasp the overall financial impact on healthcare organizations.
Initial Investment and Operational Costs
The initial investment in a Decision Support System can be substantial. This investment encompasses various aspects such as software licensing, hardware procurement, and infrastructure setup. Depending on the sophistication of the DSS, costs can range from thousands to millions of dollars. Additionally, integrating the system with existing healthcare databases and electronic health record systems can incur extra costs.
Operational costs also need careful consideration. Ongoing expenses for maintaining and updating the DSS can affect budgets. These expenses might involve:
- Software Updates: Regular updates are necessary to keep the system functioning optimally and to incorporate new medical guidelines.
- Technical Support: Ongoing technical support is required to troubleshoot issues and maintain operational effectiveness.
- Training: Personnel training is often required to ensure that healthcare staff can use the system effectively. This training generates additional costs that healthcare providers must factor in.
"DSS cost analysis should incorporate both immediate and future financial implications to ensure long-term sustainability in healthcare operations."
Long-term Financial Benefits
Despite the initial financial burden, the long-term financial benefits of implementing Decision Support Systems can be substantial. These benefits often justify the initial investment. Here are some key advantages:
- Reduced Errors: By minimizing medical errors through accurate decision-making support, organizations can avoid costly malpractice claims and improve patient safety.
- Increased Efficiency: DSS enables healthcare providers to make faster decisions. Improved efficiency can lead to shorter patient wait times and optimized resource allocation, ultimately saving money.
- Enhanced Patient Outcomes: Improved decision-making leads to better patient outcomes. This can result in higher patient satisfaction and, consequently, increased patient retention which positively influences revenue.
- Lower Operational Costs: Over time, automated processes facilitated by DSS can sufficiently lower labor and operational costs associated with administrative tasks.
In summary, while the cost implications of Decision Support Systems present challenges, the long-term financial gains often outweigh initial expenditures. Understanding these dynamics can help healthcare organizations make informed decisions about adopting and financing DSS.
Ending
The conclusion of this article encapsulates the vital nature of Decision Support Systems (DSS) in the current healthcare landscape. These systems serve as a bridge between data and informed decision-making, ultimately improving patient outcomes and enhancing operational efficiency within healthcare settings. The summary of key points presented in this article reveals that DSS are not merely tools, but integral components of healthcare practices that can transform workflows.
An essential focus has been on the diverse components and functionalities of DSS, including data management, inference engines, and user interfaces. Each of these elements plays a unique role in ensuring that healthcare providers can access accurate information and utilize it effectively during patient care. Furthermore, DSS are producing measurable benefits by reducing medical errors, fostering patient safety, and informing clinical decisions.
The considerations surrounding DSS implementation, such as user training, data quality, and regulatory compliance, are also crucial. Recognizing these aspects ensures that healthcare providers can effectively utilize DSS while adhering to industry standards and protecting patient data. The relevance of these systems is underscored by their ability to adapt to future advancements in technology, such as artificial intelligence and predictive analytics.
In summary, DSS represent a significant step forward in healthcare decision-making processes. Their integration into clinical settings not only aids practitioners but ultimately leads to better patient care.
Summary of Key Points
- DSS are essential for informed decision-making in healthcare. They leverage data and technology to support clinicians and administrators.
- Components include data management, inference engines, and user interfaces. Each of these contributes to the effectiveness of the system.
- Impact on patient safety and reduction of medical errors highlights the importance of utilizing DSS.
- Implementation challenges must be addressed. Issues like data quality and integration with existing systems are critical for success.
- Future trends in technology can further optimize DSS. Emphasis on artificial intelligence and telemedicine will continue to shape these systems.
Future Directions for Research and Development
Looking ahead, the future of Decision Support Systems in healthcare is promising yet complex. Continued research is essential to harness the full potential of DSS. Areas warranting special attention include:
- Integration with Emerging Technologies: The fusion of DSS with artificial intelligence can lead to smarter systems that learn from data, improving recommendations and efficiencies. Research can explore algorithms that enhance predictive capabilities.
- Patient-Centric Systems: Developing DSS that incorporate patient feedback can lead to more personalized care. Future studies should investigate how patient engagement can be improved to make systems more effective.
- Data Privacy Enhancements: As DSS use sensitive data, there is a need for ongoing research into privacy and security measures, ensuring compliance with healthcare regulations.
- Evaluation Frameworks: Exploring frameworks to measure the effectiveness of DSS is crucial. Metrics that capture improvements in patient outcomes can provide insights necessary for continuous improvement.
- Training Programs: The need for ongoing training for healthcare providers on evolving DSS technologies will remain significant. Researching best practices for training can enhance overall system utilization.
These focus areas provide groundwork for advancing decision-making technologies in healthcare settings, ensuring that DSS can meet the challenges of tomorrow.