Deep Data Analytics: Exploring Its Impact and Future
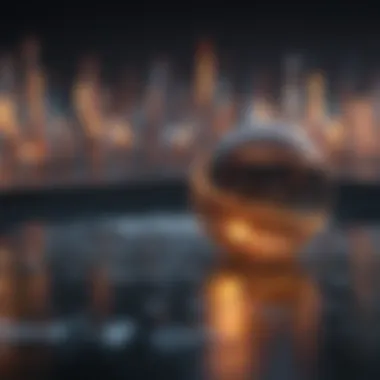
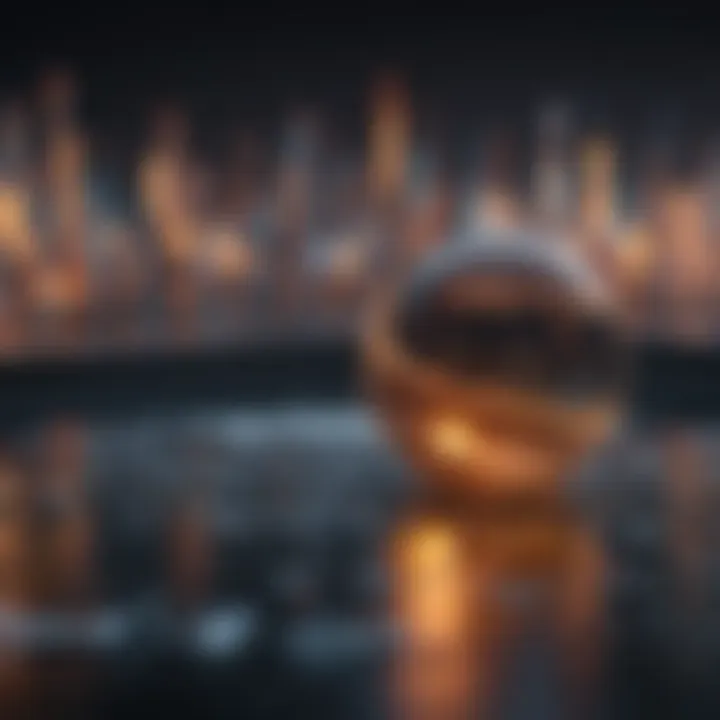
Intro
In the age of information, the ability to harness data effectively has emerged as a pivotal force behind progress in various fields. Deep data analytics stands at the forefront of this evolution, providing a means to draw insights from vast datasets that traditional analysis methods struggle to navigate. The importance of this discipline cannot be overstated; it is reshaping everything from academic research to commercial strategies.
Deep data analytics essentially refers to the techniques and technologies employed to unearth knowledge from complex and voluminous datasets. This encompasses a broad spectrum of methods, including machine learning algorithms, statistical analysis, and data mining processes. The principal aim is to drive informed decision-making by identifying patterns, anomalies, and trends that would otherwise go unnoticed.
With advancements in computational power and data storage, deep data analytics has become more accessible to researchers, educators, and professionals alike. However, as we engage with this powerful tool, it is crucial to consider not only the capabilities of these methods but also their ethical implications and potential societal impact. In the sections that follow, we will delve deeper into various aspects of deep data analytics, underscoring its relevance in contemporary research landscapes.
Understanding Deep Data Analytics
Deep data analytics represents a significant evolution in how we approach data-driven decision-making. Understanding this field not only illuminates its techniques and methods, but also highlights its importance in a rapidly changing world where data has become the new currency. With businesses and institutions generating enormous volumes of data, deep data analytics allows stakeholders to extract meaningful insights and translate them into strategic advantages.
Definition and Core Concepts
Deep data analytics can be broadly defined as the use of advanced computational techniques to analyze large sets of data. This approach goes beyond traditional analytics by leveraging sophisticated algorithms and tools, often integrating machine learning and artificial intelligence to uncover patterns that may not be immediately obvious.
Key concepts include:
- Big Data: Refers to data sets that are so large or complex that traditional data processing applications are inadequate. Deep data analytics thrives on leveraging these vast amounts of information.
- Data Mining: This is the practice of examining large pre-existing databases to generate new information. Through techniques such as clustering and classification, data mining plays a crucial role in enhancing the depth of analysis.
- Predictive Modeling: This involves using statistics to predict outcomes. By recognizing trends from historical data, organizations can anticipate future events, positioning themselves advantageously.
These concepts are instrumental, enabling organizations not only to analyze what happened but also to use that information to foresee what might happen next.
Distinctions from Traditional Analytics
While both traditional analytics and deep data analytics aim to uncover insights from data, there are key distinctions that set them apart. Traditional analytics mainly involves descriptive and basic upper-level analysis, often focusing on summarizing past performance without diving deeply into underlying patterns or trends.
In contrast:
- Depth of Analysis: Deep data analytics uses advanced methodologies that allow for a multi-dimensional exploration of data, incorporating various data types and sources. This level of detail significantly enhances the possibility of extracting actionable insights.
- Computational Techniques: Traditional methods rely heavily on straightforward calculation techniques like regression analysis or basic statistical tools, whereas deep analytics employs machine learning and neural networks to process data.
- Visualizations and Interpretation: With deep data analytics, results can be visualized through complex data representation methods, enabling better interpretation and understanding of intricate data relationships.
"Data is worth pursuing, not just for what it reveals, but for what it can become."
Understanding these differences is crucial for stakeholders who aim to leverage data more effectively in decision-making processes and strategic planning.
The Framework of Deep Data Analytics
The framework of deep data analytics serves as the backbone for understanding how conversations around data evolve, pushing the boundaries of what is possible within research and industry applications. It is a multifaceted structure encompassing various stages of analytical processes, from gathering data to interpreting results and implementing findings. This framework adds value because it allows researchers and professionals to streamline their approaches, ensuring that insights gleaned are both robust and actionable.
Data Collection Techniques
Data collection forms the first step in the analytic journey. The techniques employed at this stage can significantly influence the quality and reliability of the resultant analysis. Various methods are available, often tailored to the scope and context of the research.
- Surveys and Questionnaires: These are useful for gathering subjective data from a wide audience. They can be easily distributed online, which makes reaching a larger sample size more economical and convenient.
- Sensors and IoT Devices: In fields such as environmental science, data from sensors can capture real-time changes in conditions like temperature, humidity, and air quality. This data is granular and often yields insights that historical data cannot provide.
- Web Scraping: This method allows analysts to harvest data from websites, which can be invaluable for market analysis and sentiment tracking. Extracting data in this way lets researchers gather information at a scale and speed that would be impossible through manual collection.
Using the right collection technique helps in ensuring that the collected data aligns with the objectives of the analysis. Without this alignment, conclusions drawn can be misleading and potentially harmful.
Data Processing Mechanisms
Once data is gathered, it passes through various processing mechanisms. These mechanisms are imperative in transforming raw data into something that makes sense and can be acted upon. The processing stage often involves several distinct steps:
- Data Cleaning: Before analysis can occur, it’s critical to remove inaccuracies or inconsistencies within the dataset. This stage is all about rectifying issues, such as eliminating duplicate entries or correcting formatting errors.
- Data Integration: Here, disparate sources of data are merged to create a cohesive dataset. This might involve aligning data from internal systems with external databases, providing a fuller picture for analysis.
- Data Transformation: This is where data is reshaped into a desired format or structure. For instance, converting all date formats to a standard for consistency.
The importance of robust data processing cannot be overstated. Inaccuracies at this stage can bubble up later in the analysis, leading to faulty decisions based on flawed data.
Analytical Methods and Tools
When the data is cleaned and processed, it is ready for analysis. A variety of methods can be applied, each offering different insights based on the nature of the data and the questions being posed. Some key analytical methods include:
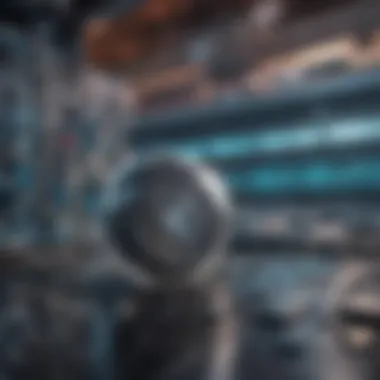
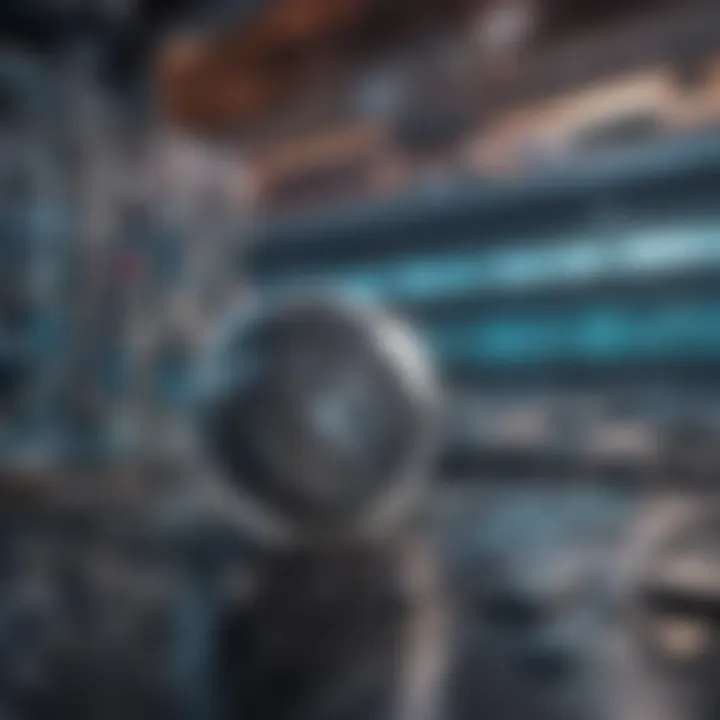
- Descriptive Analytics: This type of analysis provides a straightforward overview of data trends and patterns. It is often the first step in understanding complex datasets.
- Predictive Analytics: Here, past data is used to forecast future trends. Techniques like regression analysis and machine learning are commonly employed, making predictions based on historical patterns.
- Prescriptive Analytics: This advanced method recommends actions based on the analysis. It’s more complex, utilizing simulation and optimization algorithms.
Tools such as Tableau, R, and Python libraries empower analysts to execute these methods effectively. Each tool has its strengths and weaknesses depending on the analytical goals.
In summary, the framework of deep data analytics sets the stage for effective utilization of data through systematic collection, processing, and analysis. It’s an interconnected chain where each link enhances the strength and reliability of the final insights.
Understanding the framework is not just about tools and techniques; it's about recognizing how these elements work together to unlock the latent potential within data.
Technological Innovations Driving Deep Data Analytics
The rapid evolution in technology has fundamentally changed how deep data analytics is approached and executed. Innovations in fields such as artificial intelligence, cloud computing, and advanced visualization techniques have significantly enhanced analytical capabilities, making them more efficient and insightful. These technological advancements not only streamline processes but also enable the extraction of richer information from vast datasets, which is crucial in today’s data-driven world.
Artificial Intelligence and Machine Learning
Artificial intelligence (AI) and machine learning (ML) sit at the heart of deep data analytics. They are game-changers in the realm of data processing and sense-making. By leveraging algorithms that can learn from data, these technologies enable analysts to uncover patterns that may not be immediately obvious.
- Personalization: In industries like marketing and healthcare, AI can tailor recommendations and treatments based on unique user data. For instance, Netflix employs machine learning to suggest shows tailormade for individual preferences, vastly improving user engagement.
- Rapid Data Processing: Traditional data analysis might take days or weeks, but with ML algorithms, processing time can shrink to mere hours. This agility is crucial for applications requiring quick decision-making, such as trading in stock markets where conditions can shift in the blink of an eye.
However, while AI and ML hasten analysis, they also raise questions about transparency and accountability. How decisions made by algorithms affect various stakeholders needs careful scrutiny.
Cloud Computing and Data Storage Solutions
The onset of cloud computing has been another significant leap forward for deep data analytics. With immense storage capabilities and powerful computing resources available on demand, organizations can handle larger datasets and execute complex analyses without the need for dedicated infrastructure.
- Scalability: Cloud platforms, such as Amazon Web Services and Microsoft Azure, provide the flexibility to scale storage and processing power according to current needs, saving costs and minimizing waste.
- Collaboration: Teams across different geographical locations can access and analyze the same datasets simultaneously, facilitating collaborative efforts and fostering innovation through shared insights.
Cloud solutions also offer enhanced security features and data backup options, essential for ensuring the integrity of sensitive information.
Advanced Visualization Techniques
In deep data analytics, presenting findings in an easily interpretable format is crucial. Advanced visualization techniques transform complex data into graphics that not only inform but also engage stakeholders. By using tools such as Tableau or Power BI, analysts can create dashboards that provide a snapshot of key performance indicators, making data accessible at a glance.
- Interactivity: Users can manipulate visualizations, drilling down into specific areas of interest. This interactivity leads to deeper insights as users explore data trends dynamically rather than passively consuming static information.
- Storytelling: Good visualizations tell a story. They guide decision-makers through data, highlighting significant trends and correlations. Instead of sifting through rows of numbers, stakeholders can recognize patterns, thus speeding up the decision-making process.
"The goal is to turn data into information, and information into insight." — Carly Fiorina
Applications of Deep Data Analytics
The relevance of deep data analytics extends far beyond what meets the eye. It is the backbone of innovation in various domains. As companies and researchers grapple with vast amounts of data, understanding how to apply deep data analytics has become paramount. From healthcare to environmental studies to finance, its applications present unique benefits and challenges to navigate.
Healthcare and Medical Research
Deep data analytics plays a crucial role in transforming healthcare. It allows for innovative research techniques, improving patient outcomes and streamlining operations. For example, predictive analytics can aid in identifying at-risk populations before symptoms appear. This proactive approach can significantly reduce healthcare costs by facilitating early interventions.
- Patient Data Analysis: Advanced algorithms can sift through electronic health records, uncovering patterns that standard methods miss. This can lead to novel treatment options or more personalized care plans.
- Clinical Trials: Data analytics can improve participant selection for clinical trials, ensuring a more representative sample while accelerating the drug development process.
In essence, the integration of deep data analysis in healthcare not only enhances research but also fundamentally changes the way patient care is delivered. The emerging technologies in this field continue to unveil potential that can reshape medical paradigms.
Environmental Science and Climate Change
Deep data analytics is also pivotal in tackling environmental challenges. With the planet facing pressing issues like climate change, utilizing analytical data helps in understanding complex environmental processes. Such insights can drive effective policy-making and foster sustainable practices.
- Earth Observation: By analyzing satellite data, researchers can monitor deforestation rates or track changes in land use. These insights are essential for crafting strategies to combat climate change.
- Predictive Modeling: It predicts weather patterns, enabling communities to prepare for extreme weather events. Furthermore, it helps in modeling the impact of various factors on biodiversity.
The capacity of deep data analytics in environmental science not only assists in mitigating current problems but also aids in predicting future challenges, painting a clearer picture for forthcoming generations.
Finance and Market Analysis
In the world of finance, data is gold, and the deeper the analysis, the better the insights. Deep data analytics allows financial institutions to make informed decisions that can forecast market movements and mitigate risks.
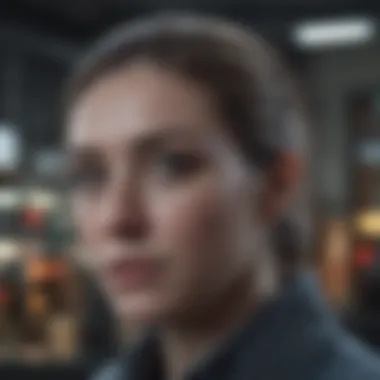
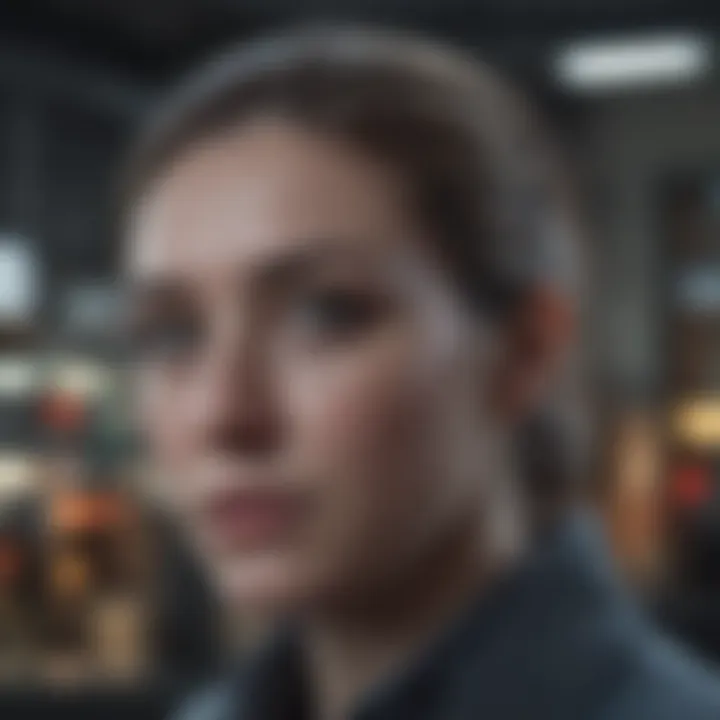
- Risk Management: Using complex algorithms, analysts can assess potential risks by examining historical data. This process enables banks and investment firms to take preemptive measures against market volatility.
- Market Trends: Institutions can analyze consumer behavior through large datasets, discovering trends that dictate buying habits and investing strategies.
In the finance sector, fortune favors the data-savvy. The integration of deep analytics empowers organizations to not only respond to change but to anticipate it, creating a more resilient economic environment.
"The true understanding of deep data analytics is not just in examining the past but in preparing for the future."
As we can see, the application of deep data analytics is diverse and impactful. Each sector benefits uniquely, unlocking doors to innovations that carry the potential to solve complex problems. The interplay of data, technology, and research continually shapes pathways toward a more informed and effective global society.
Exploring Case Studies
In the realm of deep data analytics, exploring case studies serves as a pivotal mechanism for grasping the real-world implications and practical applications of theoretical knowledge. Case studies illuminate how concepts are translated into practice, demonstrating the effectiveness of approaches while fostering an understanding of challenges faced along the journey. By diving deep into specific instances, professionals, researchers and students can comprehend the overarching benefits—but also the pitfalls—that come with implementing deep data analytics.
Successful Implementations in Industry
Examining successful implementations of deep data analytics unravels a wealth of insights across diverse industries. In healthcare, for instance, the integration of data analytics in patient management has not only streamlined operations but has also dramatically improved patient outcomes. Hospitals are leveraging predictive analytics to anticipate patient needs. This was clearly demonstrated by Mount Sinai Health System, which adopted machine learning algorithms to predict patient readmissions. By analyzing historical patient data, they were able to significantly reduce readmission rates.
Additionally, in the finance sector, companies like JPMorgan Chase have adopted deep data analytics to enhance their decision-making processes and risk assessment models. By analyzing numerous data points, they could identify fraud patterns much quicker than traditional methods allowed. These successful case studies not only highlight the tangible benefits of deep data analytics but also serve as templates for best practices that others can adapt.
"In an era where data is the new oil, knowing how to drill for and refine that data is the game changer."
Transitioning from these professional successes, it's evident that case studies shed light on not just strategies, but also on the culture within organizations. When data-driven decision-making becomes part of the organizational fabric, stellar results tend to follow.
Learnings from Failed Initiatives
On the flip side, it’s equally crucial to analyze cases where deep data analytics initiatives fell flat. These instances provide lessons that are just as valuable as successes. For example, a notable failure occurred with Target's predictive analytics model. The retail giant aimed to identify customer shopping habits to enhance marketing strategies. However, the implementation didn’t account for privacy concerns which caused a backlash among customers when specific and sensitive data points were incorrectly assumed to indicate pregnancy.
Such failures illustrate the importance of ethical considerations in data handling. The fallout from these mistakes can obliterate customer trust, ruining reputations that companies have spent decades building. Moreover, these missteps can highlight fundamental misunderstanding or underestimation of data quality issues. For instance, if various data sets aren’t of high caliber or are inconsistent, the insights derived can lead to misguided decisions, culminating in wasted resources and effort.
Through this lens, organizations can glean substantial insights into what not to do, catalyzing more informed decision-making in future projects. The contrast between success and failure, when examined closely, guides teams toward improved methodologies and ethical frameworks.
In sum, exploring case studies not only serves as a platform for showcasing the power of deep data analytics but also compels continuous reflection on practices within this ever-evolving field. With each example—both successful and otherwise—students, researchers, and professionals can elevate their understanding and application of deep data analytics.
Ethical Considerations in Data Analytics
In today's world, where data drives decision-making across various sectors, addressing ethical considerations in data analytics is paramount. This section elucidates the complexities involved in ensuring that data analytics practices are responsible, fair, and transparent. One can hardly underestimate the significance of ethical standards; without them, trust between data providers and users could erode, leading to potential misuse of sensitive information.
Privacy and Data Protection
Privacy stands as a cornerstone of ethical data usage. Individuals often overlook that data isn't just numbers or trends; it's personal stories—biographies captured in bits and bytes. Every time data is collected, there are unseen lives interconnected with that data. It’s necessary to adopt robust measures for privacy and data protection. This not only fulfills legal requirements but also builds trust with data subjects. Privacy-enhancing technologies like anonymization and encryption play crucial roles here. These tools shield personal information while allowing organizations to leverage analytics for insights. Moreover, considering legislation such as the General Data Protection Regulation (GDPR) emphasizes accountability and transparency. Organizations must develop a culture of respect for privacy which transcends mere compliance, ensuring that ethical considerations are woven into the fabric of data handling processes.
Bias and Fairness in Algorithms
Bias in algorithms is another ethical elephant in the room that warrants attention. Algorithms can reflect and magnify societal biases if appropriate measures are not taken. For example, inadequately trained models may discriminate against certain demographic groups—leading to unfair outcomes in areas like hiring or lending. This raises a fundamental question: how can one ensure fairness in algorithms?
One approach is continuous monitoring of algorithms and the development of inclusive datasets used for training. Recognizing that a diverse dataset minimizes bias is crucial. Furthermore, incorporating fairness audits at various stages of the analytical process helps identify and address biases proactively.
A conscious understanding and application of fairness principles can transform data analytics into a force for good, ensuring that technology serves everyone equitably.
"The path of ethical data analytics not only enhances the accuracy of insights obtained but also reinstills faith in the systems designed to help us interpret our complex world.”
Emphasizing these ethical considerations isn't just an option but a necessity in the age of data. As we transition towards more sophisticated analytics, the importance of privacy, protection, algorithmic fairness, and bias cannot be overstated. Engaging with these concepts proactively enhances the integrity of data-driven endeavors and ultimately contributes to a more equitable society.
Challenges in Deep Data Analytics
Navigating the landscape of deep data analytics is no easy feat. It's a wild world filled with both opportunities and obstacles. For professionals, students, and researchers working in this field, understanding these challenges is crucial. Not only do these complications affect the quality of insights derived, but they can also shake the foundation of decision-making processes that heavily rely on data. This section dives into two significant hurdles: data quality and integrity issues, along with the scalability of analytical processes.
Data Quality and Integrity Issues
When it comes to data, quality is king. The integrity of data refers to the accuracy and consistency of information over its entire lifecycle. Poor data quality can result in screwy analytics, leading to misinformed decisions that might send strategies down the wrong path. Let's dig into a few common pitfalls that arise from inadequate data quality:
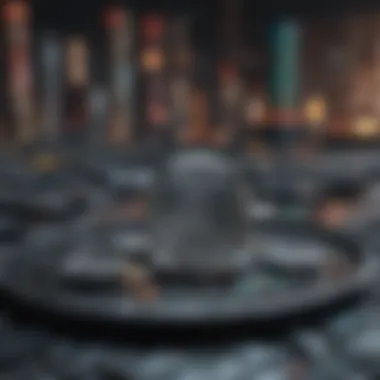
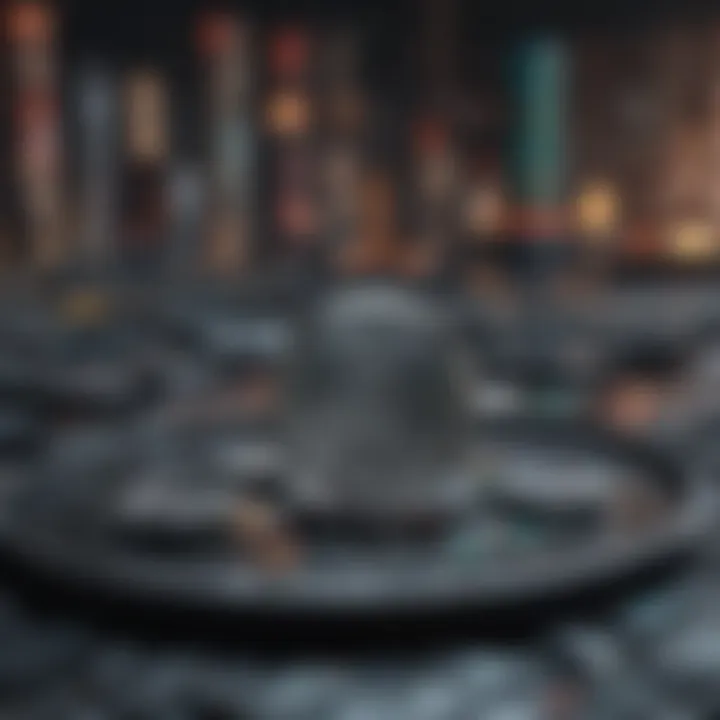
- Inaccurate Data Entry: Mistakes during manual entry can lead to erroneous datasets, which then impact analytical results.
- Outdated Information: Data that has not been updated can misrepresent current conditions, particularly in fast-paced industries like finance or tech. Relying on stale data is like trying to drive a car by looking in the rearview mirror.
Furthermore, there’s the question of source credibility. Not every dataset harvested from the internet holds water. Understanding the origin of the data can prevent surprises down the line. Considerations such as:
- Where did the data come from?
- Is it compliant with regulations?
- How was the data collected?
These aspects are crucial for ensuring that any conclusions drawn later on are trustworthy and actionable. In short, maintaining high standards for data quality not only enhances analytical outcomes but also reinforces the overall efficacy of deep data analytics initiatives.
Scalability of Analytical Processes
As data grows at an exponential rate, scaling analytical processes becomes necessary. A one-size-fits-all approach simply doesn’t cut it anymore. When we talk about scalability, we address how analytics efforts can adapt to handle increasing amounts of data efficiently. Here are highlighted points of concern in this regard:
- Infrastructure Capacity: Most organizations struggle with outdated hardware that simply can’t handle the massive influx of data. Investing in cloud computing solutions, like Amazon Web Services or Google Cloud, can make a world of difference.
- Tool Adaptability: The software being used must keep pace with growing data demands. Tools that can enhance speed and efficiency are vital. Otherwise, analytics efforts may stall on the launch pad, leading to missed opportunities.
- Team Expertise: It's not just about having fancy tools. If the team lacks relevant skills or training, problems can crop up fast. Continuous education and stating current with trends can prevent analytics teams from becoming stale themselves.
The struggle for scalability isn't just an operational dilemma; it's an existential one for data-driven organizations. Companies that gear up for scalability find themselves ready to harness advanced analytics more effectively, giving them a leg up in their respective fields.
"In the world of data, quality and scalability are not optional; they are essential for thriving in chaos."
In summation, the challenges of data quality and scalability in analytical processes must be recognized and addressed. Only by focusing on these areas can individuals and organizations unlock the full potential that deep data analytics offers.
Future Trends in Deep Data Analytics
The landscape of deep data analytics is constantly evolving, shaped by technological advancements and shifting societal needs. Not only does this area drive the future of research, but it also influences numerous sectors, making its trends pivotal for anyone involved in data-driven decision making. Understanding these trends means grasping the nuances of how data will be generated, processed, and analyzed going forward. This section unpacks significant elements that will play a key role in the future trajectory of deep data analytics and discusses the implications for various stakeholders.
Emerging Technologies and Methodologies
In the realm of deep data analytics, emerging technologies are the spearhead of innovation. We are standing on the brink of transformative changes that will redefine data interaction. Some of the most promising technologies include:
- Quantum Computing: The potential to process complex datasets at lightning speed could revolutionize analysis capabilities. Quantum systems can handle calculations that are unfathomable for traditional computers, enabling deeper insights into vast datasets.
- Augmented Analytics: This methodology uses machine learning to assist data preparation, which not only saves time but also enhances accuracy. By allowing automated insights generation, professionals can focus on strategic decision-making rather than mundane tasks.
- Edge Computing: As more devices get connected, processing data closer to its source becomes essential. Edge computing can reduce latency and bandwidth usage, making real-time analytics more feasible across various applications.
Each of these technologies invites a rethink of current practices and could help address inefficiencies in the existing data analytics landscape.
Predictive Analytics and Forecasting
Predictive analytics is not new, but its continued evolution is crucial. Through leveraging historical data, it helps organizations forecast future trends and behaviors. Considerations for the evolution of predictive analytics are manifold:
- Integration of AI: By embedding artificial intelligence into predictive models, firms can enhance predictive accuracy. AI allows for the processing of unprecedented amounts of data, leading to more actionable insights.
- Focus on Real-time Data: Traditional predictive analytics often relied on static datasets. The future sees a shift towards using real-time data, making forecasts far more relevant as they evolve alongside incoming information.
- Human-Centric Design: There's a rising recognition of the importance of human factors in data interpretation. Models must not just be algorithmically sound but also understandable and usable for decision-makers.
Predictive analytics isn't merely about crunching numbers anymore; it's about engaging multifaceted variables that breathe life into the data. As a result, organizations not only gain foresight but also the ability to adapt swiftly to changing conditions.
"Deep data analytics is becoming the bedrock on which future innovations stand. By aligning technological advancements with analytical methodologies, we pave the way for uncharted territories in research and application."
The End and Reflection
In the final stretch of our exploration into deep data analytics, it becomes crystal clear how vital this field is for modern research and innovation. The discussions presented have illuminated the multifaceted nature of data and its profound implications across various disciplines. As we sift through heaps of information, it's essential to distill what truly matters and reflect on the pivotal insights that emerge.
Summation of Key Insights
As we wrap things up, several themes resonate throughout our journey. Here are some key takeaways:
- Analytical Depth: Deep data analytics elevates the analysis process from surface-level interpretations to in-depth understanding. Through advanced methods like machine learning and AI, patterns hidden neck-deep in data can be uncovered.
- Impact Across Fields: Whether in healthcare, finance, or environmental science, applying deep data analytics can reshape industries, leading to improved outcomes and smarter decision-making.
- Ethical Awareness: One cannot overlook the ethical considerations entwined with data use. Issues such as privacy concerns and algorithmic biases have gained traction, highlighting the need for responsible practices and developments.
- Future Readiness: Embracing emerging technologies and continuously adapting methodologies positions researchers and practitioners to stay ahead of the curve. A proactive stance on future trends paves the way for innovative breakthroughs.
In essence, the study of deep data analytics is not merely academic but a bottom-line necessity in navigating the complexities of the information age.
Encouragement for Continued Exploration
Let's not put the brakes on our curiosity just yet. The landscape of deep data analytics is ever-evolving, and the future holds even greater possibilities. Here’s why further exploration is crucial:
- Lifelong Learning: The field is rapidly changing, and staying informed about new tools and methodologies can significantly enhance one’s analytical capabilities. Enrolling in courses or attending workshops could be a valuable step.
- Networking Opportunities: Engaging with like-minded professionals and experts can lead to fruitful collaborations. Online platforms such as Reddit or LinkedIn abound with forums to discuss and share insights about data analytics.
- Real-World Application: As we dive deeper into emerging technologies, applying learned concepts to real-world problems nurtures ingenuity. Take the initiative to explore case studies, volunteer for projects, or engage in community-driven data initiatives.
"In every problem, there is a realm of knowledge waiting to be uncovered; it’s only by continuously seeking that we truly advance."
Last but not least, always keep an eye out for fresh literature. Read pieces from reputable sources like Britannica or Wikipedia to remain updated on the ongoing advancements in deep data analytics.
In summary, deep data analytics stands as a cornerstone of modern scientific inquiry. Engage with curiosity, reflect critically, and continually explore the vast landscape it encompasses.