Exploring Digital Twin Images and Their Applications
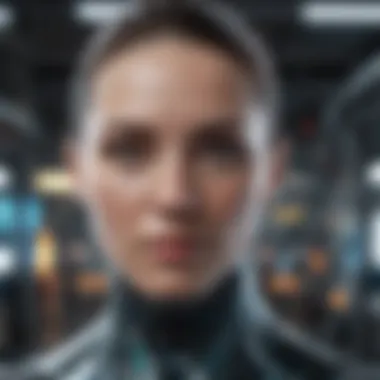
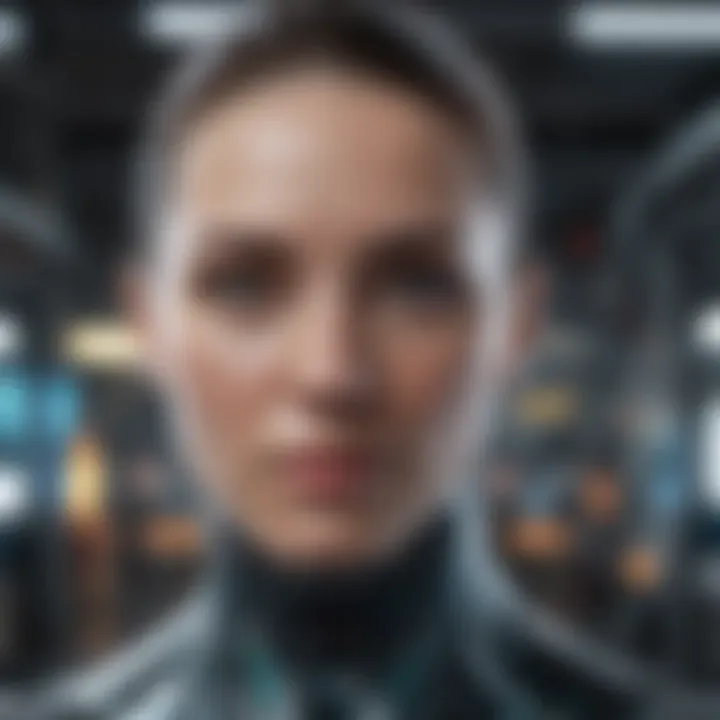
Intro
In the current landscape of advanced technology, the term digital twin images is gaining traction. These images offer a unique lens through which we can analyze the behavior and performance of physical objects or systems by utilizing real-time data. Essentially, a digital twin acts as a mirror, reflecting the physical counterpart's state, processes, and parameters in a virtual environment. This duality paves the way for enhanced decision-making, predictive maintenance, and a deeper understanding of how intricate systems operate.
As industries evolve, the integration of digital twin technology becomes pivotal. In sectors ranging from manufacturing to healthcare, the ability to simulate, track, and optimize processes has profound implications on efficiency and innovation. Yet, piecing together a full picture of digital twin images requires diving into their foundational elements, challenges, and the myriad of possibilities they unlock.
As we embark on this exploration, we will detail the remarkable journey of digital twin images from their theoretical roots to practical applications, with special focus on the intertwining of data, modeling, and predictive analysis. Whether you're a student, researcher, or industry professional, understanding this technology holds significance for grasping its trajectory and potential in the real world.
Foreword to Digital Twins
Digital twins have emerged as a crucial component in the landscape of modern technology, enabling innovative solutions across various sectors. Understanding digital twin images is vital because they serve as virtual replicas of physical entities or systems. This improves decision-making processes, enhances productivity, and aids in predictive maintenance. In the context of rapidly developing technologies, an exploration of digital twins isn’t just informative; it is essential.
Definition of Digital Twin Images
Digital twin images refers to the digital representation of a physical object, process, or system. It captures not only the physical characteristics but also the behavior and performance of the entity in a virtual environment. This concept goes beyond just simple modeling; it embodies the idea of creating a real-time relationship between the digital and physical worlds. For instance, imagine a digital twin of a wind turbine – it reflects real-time data about energy output, structural integrity, and environmental conditions. This information helps in monitoring and optimizing performance effectively.
In more precise terms, a digital twin acts as a bridge connecting data and application, allowing stakeholders to visualize and interact with system behaviors before implementing changes. Successful companies leverage this technology not just for analysis but also for forecasting outcomes based on varying scenarios.
Historical Context
The roots of digital twins may be traced to the early 2000s, but the concept truly began to gain traction with the evolution of the Internet of Things (IoT) and advanced data analytics. The idea is credited to Michael Grieves, who introduced the concept during a presentation at the University of Michigan in 2002. At that time, the focus was more on product lifecycle management.
As technology advanced, the application and scope of digital twins expanded significantly. In aerospace, Boeing utilized these models for performance analysis of jet engines. Meanwhile, in the manufacturing sector, General Electric adopted digital twins to predict machine failures and optimize factory processes.
Over the years, significant advancements in sensor technology and cloud computing have accelerated the capabilities of digital twin technology. Now, organizations can integrate vast amounts of data seamlessly, updating their digital twins in real time.
Overall, the journey from a theoretical concept to a practical tool in various industries underscores the relevance and potential of digital twin images today. As technology continues to evolve, understanding its historical context is key to appreciating its present applications and future implications.
Technological Foundations
The technological foundations of digital twin images serve as the bedrock upon which these innovative concepts are constructed. Understanding these elements is crucial for grasping how digital twins can facilitate improved decision-making and efficiency across various sectors. The interplay of data acquisition technologies, modeling and simulation techniques, and the inclusion of artificial intelligence cannot be underestimated. Together, these aspects create a comprehensive ecosystem that powers the functionality and applicability of digital twins in real-world situations.
Data Acquisition Technologies
Data acquisition technologies are pivotal in establishing a reliable digital twin. These technologies pull in crucial data from the real world, thereby enabling the creation of an accurate virtual representation. Methods like sensors, IoT devices, and even satellite imagery are commonly employed for this purpose.
Each of these technologies contributes in unique ways:
- Sensors: Devices that collect data regarding physical properties such as temperature, pressure, and humidity. They are often embedded in machinery, allowing for real-time monitoring.
- IoT Devices: These internet-connected devices enhance data collection through continuous tracking. They help gather useful insights from diverse environments—factories, homes, and even smart appliances.
- Satellite Imagery: For broader applications, especially in fields like agriculture or urban planning, satellite imaging collects data across vast geographic areas. This helps in understanding patterns that inform decisions.
The accuracy and timeliness of the data gathered through these methods can make or break the effectiveness of a digital twin. When the input data is of high quality, the resulting digital twin holds greater predictive power and reliability.
Modeling and Simulation Techniques
Once data is collected, modeling and simulation techniques come into play. These practices allow analysts to create a virtual replica that mimics the behavior of its physical counterpart. It's the bridge between raw data and actionable insight.
In this context, a few crucial techniques include:
- Finite Element Analysis (FEA): Commonly used for structural analysis, this technique breaks down complex structures into manageable elements. By analyzing these, engineers can predict how structures respond to various conditions.
- Computational Fluid Dynamics (CFD): This simulation technique addresses fluid movements. It's particularly significant in aerospace and civil engineering, where air or water flow can significantly impact operations.
- Digital Twin Models: Building a digital twin involves integrating modeling tools that simulate the real-world behavior accurately. These models can help visualize potential outcomes before implementing physical changes.
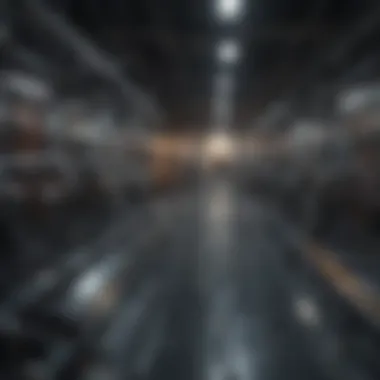
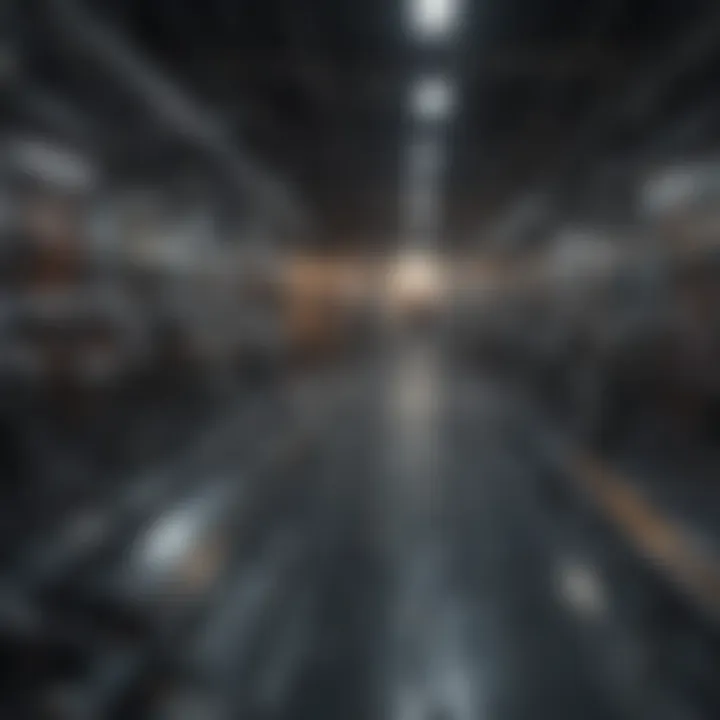
Modeling and simulation techniques enable stakeholders to visualize scenarios and test hypotheses without the risks associated with real-world trials. This capability ultimately drives innovation, reducing costs and enhancing overall productivity.
Artificial Intelligence in Digital Twins
Artificial Intelligence (AI) infuses digital twin technology with the ability to learn and adapt. Integrating AI algorithms into digital twins allows them to analyze data more profoundly, draw insights, and even predict future states of systems and processes.
Here are a few critical applications of AI within digital twins:
- Predictive Maintenance: AI algorithms can analyze real-time data to forecast equipment failures before they occur. This proactive approach minimizes downtime and repair costs.
- Optimization: AI finds patterns in collected data that humans might miss. This capability can lead to improvements in operational efficiency, resource allocation, and performance optimization.
- Scenario Simulation: Utilizing machine learning models, digital twins can simulate various scenarios using historical and real-time data. This allows organizations to run "what if" analyses, testing the impacts of changes in a non-disruptive manner.
In the world of digital twins, the marriage of AI and data acquisition is not just beneficial; it is fundamental. Without it, the potential for optimization and foresight would remain largely untapped.
In summary, the technological foundations of digital twin images encompass data acquisition technologies, modeling and simulation techniques, and the application of artificial intelligence. Together, they form a robust framework that underpins the advantages of digital twins, paving the way for their growing adoption across numerous industries. This synergy not only enhances operational efficiency but also equips organizations to navigate the complexities of modern technological landscapes.
Applications Across Industries
The exploration of digital twin images transcends mere theoretical discussions; it filters into practical realms across various sectors. The ability to create accurate digital representations of physical entities transforms industries by enabling enhanced modeling and simulation. Companies are leveraging digital twins to optimize processes, reduce waste, and innovate solutions, thereby adding significant value. In this section, we will delve into specific applications, examining how different industries harness the power of digital twin technology, the unique benefits they reap, and the implications that come with such advancements.
Manufacturing and Production
In the manufacturing sector, digital twin images play a pivotal role in bridging the gap between the physical and digital worlds. By creating a comprehensive model of production lines, manufacturers can simulate operations without disrupting actual activity. This enables them to assess efficiencies, identify bottlenecks, and predict maintenance needs before they escalate into serious issues. For instance, consider a factory producing automotive parts. By utilizing digital twins, managers can visualize the entire assembly process, enabling real-time adjustments based on simulations of different scenarios.
- Benefits of Digital Twins in Manufacturing:
- Cost Reduction: Minimizing unexpected downtimes reduces costs significantly.
- Optimized Supply Chains: Enhanced insights lead to better inventory management.
- Quality Control: Predictive analytics allow for early detection of defects in manufacturing processes.
Healthcare Innovations
Digital twin technology also arrives powerfully in the healthcare field, where personalized medicine is gaining momentum. By creating digital replicas of patients, healthcare providers can simulate treatment plans and anticipate patient responses to various interventions. For example, a hospital may employ digital twins to model a patient's cardiovascular system to predict the outcomes of a surgical procedure. This approach not only improves patient safety but also fosters tailored treatment plans.
"With digital twins, we are ushering in a new era of personalized patient care, paving the way for tailored solutions that can significantly enhance treatment outcomes."
- Key Aspects to Note:
- Enhanced Medical Training: Medical professionals can practice on virtual models before actual procedures.
- Resource Allocation: Better visibility into patient needs aids in appropriate resource distribution.
- Chronic Disease Management: Continuous monitoring through digital twins helps in real-time adjustments to treatment plans.
Smart Cities and Infrastructure
As urbanization ramps up, cities face increasingly complex challenges in managing infrastructure and services. Digital twin images offer an innovative solution to visualize city dynamics and optimize resource allocation. For instance, by simulating traffic patterns using a digital twin of a city, planners can identify congestion points and improve transportation networks efficiently. The models help in predicting the impact of new projects, allowing for data-driven decisions that minimize disruption.
- Importance for Smart Cities:
- Sustainability Initiatives: Monitoring emissions and resource use in real-time encourages sustainable practices.
- Emergency Preparedness: Predictive modeling assists in planning for disaster responses.
- Public Services Optimization: Enhances delivery effectiveness through accurate modeling of needs.
Aerospace and Defense Applications
In the aerospace and defense realm, the implementation of digital twin images proves invaluable for both safety and efficiency. Aircraft manufacturers utilize digital twins for testing and monitoring, significantly reducing costs associated with physical testing. In a military context, tracking the performance of equipment through digital twins allows for proactive maintenance strategies that minimize the risk of failures during operations.
- Notable Benefits:
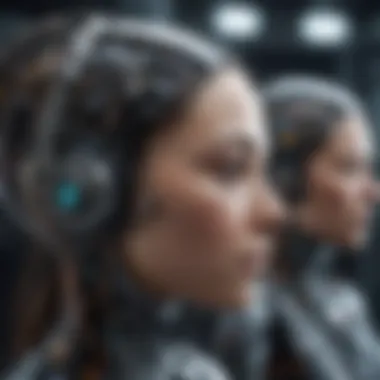
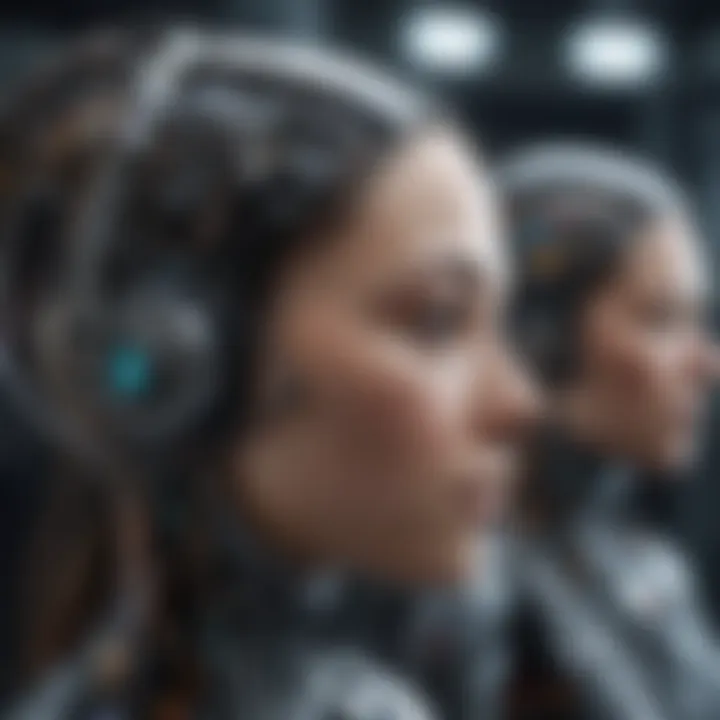
- Lifecycle Management: Monitoring systems throughout their lifecycle leads to informed upgrades.
- Simulation of Mission Scenarios: Training simulators enhanced by digital twin technology offer realistic environments for personnel preparation.
- Cost Efficiency: Reduces the necessity for physical prototypes and extensive testing.
Through these varied applications across industries, it's clear that digital twin technology is not merely a theoretical concept but rather a transformative force shaping operational strategies and outcomes. The prospects it holds are expansive, revealing the powerful interplay between the digital and physical worlds.
Data Integration and Interoperability
In the rapidly evolving landscape of digital twin technology, the concepts of data integration and interoperability emerge as crucial cornerstones. Data integration refers to the process of consolidating data from different sources into a unified view, while interoperability emphasizes the ability of various systems and devices to work together seamlessly. Understanding these elements not only enhances the functionality of digital twin images but also amplifies their application across multiple domains.
When a digital twin is created, it integrates real-time data from its physical counterpart, making it vital to ensure that data from various sources can communicate effectively. This means having standardized data formats and protocols, which contribute to consistent interpretation across platforms.
Importance of Data Standards
Clearly defined data standards play a pivotal role in the realm of digital twins. These standards facilitate compatibility between disparate systems, allowing for smoother data flows and interaction. Without a common language, the real potential of digital twin technology can’t be realized. For instance, consider the automotive industry where manufacturers use digital twins to simulate vehicle performance. If each manufacturer used different specifications or measurement units, the integration of these digital representations would be cumbersome, if not impossible. Thus, adopting universal data standards fosters uniformity, ensuring that all data is comprehensible by all devices involved.
Moreover, data standards not only improve integration but also have implications for governance and compliance. Standards help in identifying best practices, establishing protocols for data access, and ensuring privacy and security measures are met. This is especially significant in regulated industries like healthcare where sensitive data needs stringent handling.
Challenges of Data Silos
While the importance of data integration and standards is evident, the reality is often riddled with challenges, including the ubiquitous presence of data silos. A data silo occurs when information is trapped within a single department or system, unable to connect to other segments of an organization. This segregation can be detrimental for digital twins, which thrive on comprehensive data streams from various sources.
- Communication Breakdown: If only certain systems can provide or interpret specific data, the overall effectiveness of the digital twin can be severely hindered. For instance, in smart city applications, traffic management systems must communicate with public transport systems to provide accurate predictions and autonomous adjustments based on real-time conditions.
- Inefficiencies in Management: Organizations with data silos risk suboptimal decision-making. If insights are limited to fragmented data, the resulting strategies will likely lack the depth necessary for effective responses to challenges.
- Increased Costs: Maintaining multiple, isolated systems can lead to increased operational costs. Streamlining data sources and ensuring interoperability would not only reduce these costs but also enhance operational efficiency.
"For any digital twin initiative to succeed, breaking down the barriers of data silos is imperative. Only then can a seamless flow of information empower predictive models and adaptive systems."
Challenges in Implementing Digital Twin Technology
Implementing digital twin technology is no walk in the park. While the concept promises a wealth of benefits across various sectors, it also presents a myriad of challenges that organizations must navigate. In this section, we’ll break down the technical limitations, cost factors, and data privacy concerns that come with leveraging digital twin images, helping the reader grasp the complexity and considerations at play.
Technical Limitations
At its core, the technical challenges associated with digital twins primarily stem from the sophistication needed to create and maintain accurate models. Not every organization has access to the level of data integration and computational resources required to run effective digital twins.
One major hurdle is the disparity in data quality and resolution. Many organizations struggle with obtaining real-time data that can fuel these models effectively. For instance, a manufacturing plant may have sensors that gather plenty of data, but if that data is inconsistent or incomplete, the digital twin will produce flawed outputs. It becomes a case of garbage in, garbage out. As George Santayana famously said, “Those who cannot remember the past are condemned to repeat it,” so too can an organization find itself mired in inefficient processes if it does not first resolve its data integrity issues.
There’s also the question of interoperability. Many businesses utilize legacy systems that don’t easily connect with newer technologies. Without seamless communication between systems, creating a comprehensive digital twin that accurately reflects the physical counterpart can be a daunting task. The technical limitations may not only inhibit functionality but can also limit the insights organizations hope to gain from these digital representations.
Cost Factor and Resource Allocation
Cost is another significant consideration when thinking about digital twin implementation. The investment can be steep, and organizations must weigh the short-term outlay against long-term value. Building a digital twin framework can require high initial capital expenses, from purchasing advanced sensors and computational resources to hiring experts who understand the intricacies of modeling.
A business must plan accordingly and allocate resources wisely. It’s important to assess whether the potential return on investment justifies the expenditures involved.
“A penny saved is a penny earned.” This old adage rings particularly true in the realm of digital twins, where understanding where to cut costs without compromising quality can be a game changer.
Additionally, proper resource allocation extends beyond financial considerations. Organizations often find themselves juggling various projects, and digital twin initiatives can slip through the cracks if not prioritized appropriately. This can lead to subpar implementation and, ultimately, failure to harness the benefits that digital twins can offer.
Data Privacy Concerns
As organizations dive into the realm of digital twins, data privacy emerges as a crucial issue. Digital twin technology involves the continuous gathering and processing of vast amounts of data, often including sensitive information.
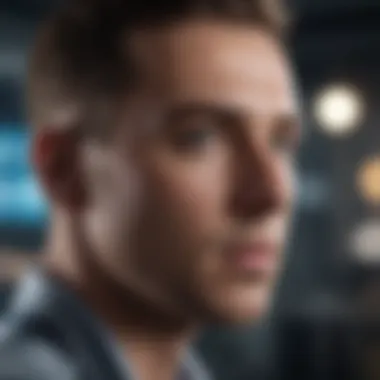
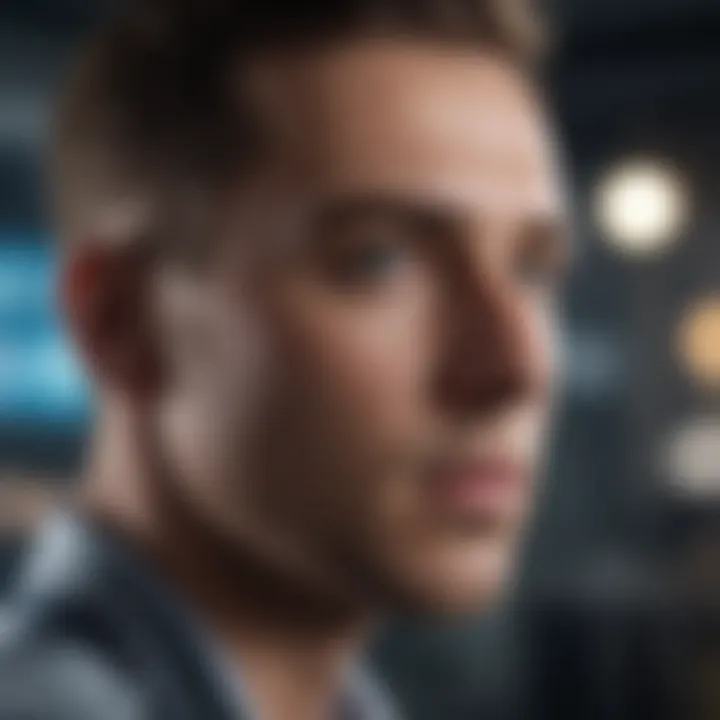
With the rise of regulations such as GDPR and CCPA, organizations must tread carefully in preserving user privacy while still leveraging data to enhance their operations. The fine line between useful insights and potential breaches can lead to major liabilities if organizations are not extemely vigilant.
Thus, it becomes paramount for businesses to establish robust data governance strategies, ensuring both compliance and security. Regular audits of data collection practices are essential to avoid falling victim to breaches or misuse of information. Organizations must also be open about their data usage policies with customers and stakeholders, maintaining transparency in how digital twin images are utilized.
In summary, while digital twin technology holds incredible promise, the challenges surrounding its implementation cannot be underestimated. From technical issues to cost allocations and data privacy, understanding these obstacles is key to navigating this complex landscape successfully.
Future Directions of Digital Twin Technology
As we peer into the horizon of digital twin technology, the future looks bright and bit complicated. The evolving landscape presents diverse opportunities alongside a raft of challenges. As this technology grows, understanding its future directions becomes crucial for stakeholders across various fields. We shall dive deep into not only the potential but also the implications of these advancements, ensuring that the significance of digital twin images is not merely theoretical but practically applicable.
Potential for Enhanced Predictive Analytics
Predictive analytics stands out as one of the most compelling prospects within the realm of digital twin technology. By harnessing the advanced computing power and algorithms, organizations can utilize digital twin images to predict future outcomes and behaviors more accurately.
These digital replicas simulate real assets and processes, allowing for data-driven decision-making that was unimaginable just a few years back. Imagine a factory equipped with a digital twin: operators can analyze machine usage patterns, foresee maintenance needs, and minimize downtime. This could result in significant cost reductions and improved efficiency.
- Benefits of Enhanced Predictive Analytics include:
- Reduction in unexpected failures by addressing potential issues before they arise.
- Increased operational efficiency through optimized resource allocation.
- Improved customer satisfaction as services and products are refined based on real-time insights.
However, adopting predictive analytics isn't without its caveats. Organizations need to grapple with issues such as data quality, integration challenges, and security concerns regarding sensitive information. As the saying goes, "You can't manage what you don’t measure;" therefore, ensuring that data is accurate and reliable is absolutely crucial in this context.
Integration with Emerging Technologies
The interplay between digital twin technology and emerging technologies shapes a new paradigm for innovation. One of the most striking opportunities lies in the integration with Internet of Things (IoT) devices. As devices become smarter and more interconnected, the ability to create more precise and real-time digital twins grows exponentially.
For instance, consider a smart city that utilizes a digital twin to monitor traffic flow, energy consumption, and public safety. By linking real-time data from thousands of IoT sensors to the digital twin, city planners and managers can proactively address issues like congestion and resource allocation.
- Key Emerging Technologies That Complement Digital Twins:
- Artificial Intelligence (AI): Enhances the analytics capabilities of digital twins, making predictions more precise.
- Blockchain: Adds a layer of security and transparency to the data being shared across various stakeholders.
- Augmented Reality (AR): Can provide an immersive experience for users to interact with the digital twin in a more engaging manner.
Although the prospects of this integration are enticing, stakeholders must also be aware of the complications that might arise. Issues such as interoperability among different systems need to be addressed, and data governance must be prioritized to ensure that ethical considerations remain at the forefront of these developments.
The convergence of digital twins with emerging technologies may rewrite the rules of engagement in numerous sectors, offering both significant benefits and challenges to overcome.
In sum, the future directions of digital twin technology signify a rich tapestry of possibilities replete with potential for enhanced predictive analytics and seamless integration with emerging technologies. The journey ahead is as promising as it is demanding, and it beckons organizations to both adapt and innovate. Every step taken, whether big or small, can lead to profound implications not just for individual entities, but also for entire industries.
Finale
As we draw the curtains on our exploration of digital twin images, it's essential to grasp why this topic holds such significant weight in today's digital age. The burgeoning field of digital twins is not merely a technical fad but a substantial leap towards enhanced efficiency and insight across various sectors.
Recap of Key Takeaways
Digital twin technology stands out for its ability to create a seamless replica of physical objects or processes, allowing for real-time monitoring, predictive analysis, and improved decision-making.
- Definition: Digital twins are sophisticated digital representations crucial for analysis and improvement in diverse fields ranging from manufacturing to healthcare.
- Tech Foundations: The technology relies on robust data acquisition and insightful modeling techniques. Best practices in simulation empower these images to evolve and adapt.
- Applications: Industries are leveraging digital twins to optimize operations, anticipate maintenance, and improve customer experiences.
- Future Prospects: With the integration of emerging technologies, the future of digital twins appears bright, especially concerning analytics.
"Digital twins bridge the gap between the physical and the digital worlds, offering unprecedented insights into operational performance."
The Significance of Digital Twin Images
The weight of digital twin images extends beyond mere representation. They embody a transformative approach to handling complex systems and processes. Here’s why they matter:
- Real-time Data Integration: Digital twin images facilitate a continuous flow of data, enhancing situational awareness and operational efficiency.
- Predictive Insights: By harnessing predictive analytics, companies can foresee issues before they arise, thereby saving time and resources.
- Enhanced Collaboration: A clear digital representation fosters better communication among stakeholders, allowing for collaborative problem-solving.
- Informed Decision-Making: Decision-makers can visualize scenarios and outcomes, leading to more informed choices that are backed by solid data.