The Role of Research Data Managers in Science
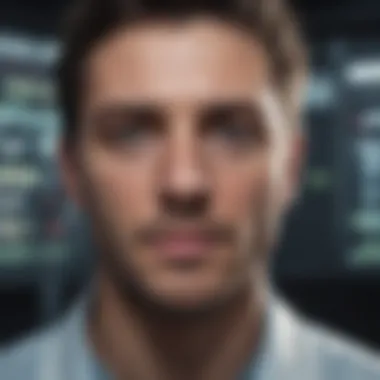
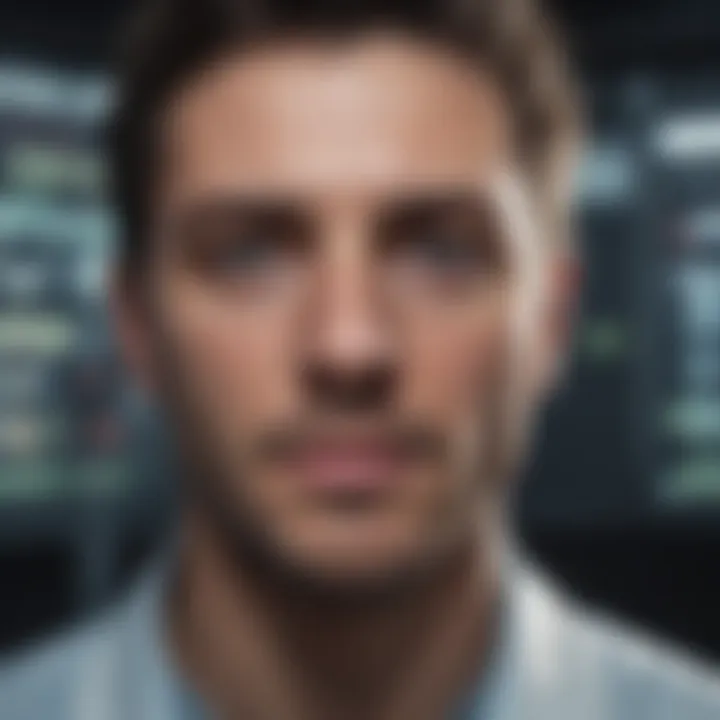
Intro
In the rapidly evolving landscape of scientific inquiry, the role of Research Data Managers (RDMs) is becoming increasingly crucial. As researchers delve deeper into complex projects, often generating terabytes of data, the expertise of RDMs helps navigate the multifaceted challenges presented by data management. From ensuring that data is stored securely and is easily accessible, to fostering collaboration among interdisciplinary teams, RDMs are quietly shaping the future of research.
But what exactly do RDMs do? Their responsibilities extend beyond mere data storage. They are the unsung heroes ensuring data integrity and promoting best practices that ultimately enhance the quality of scientific research. As we uncover the various facets of their roles, it becomes clear how indispensable they are in modern research settings.
Research Highlights
Key Findings
- Data Integrity: RDMs help create and enforce protocols that ensure data accuracy and reliability. This minimizes the risk of errors in data that can jeopardize research outcomes.
- Promoting Collaboration: By facilitating access to research data, RDMs enable teams from different backgrounds to collaborate more easily. Utilizing shared platforms and tools, they create environments ripe for innovation.
- Enhancing Accessibility: RDMs ensure that research data is not just locked away in databases but is accessible to researchers, policymakers, and the public, thus encouraging transparency.
"Data is the new oil, but without proper management, it’s just land with untapped potential."
- Adapting to Technology: With emerging technologies like AI and big data analytics making their mark, RDMs continually adapt practices to leverage these tools in data management, thus enhancing research outcomes.
Implications and Applications
The insights generated from RDMs' work have substantial implications, such as:
- Advanced Data Policies: Institutions can build robust data policies that uphold ethical standards and best practices, in turn enhancing compliance and governance.
- Improving Research Quality: As data becomes more reliable and accessible, the quality of research improves, resulting in findings that researchers and stakeholders can trust.
- Future-Proofing Research: By keeping abreast of technological advancements, RDMs prepare research teams for future challenges, ensuring that they remain at the cutting edge of scientific inquiry.
Methodology Overview
Research Design
The examination of RDMs’ roles requires a mix of qualitative and quantitative approaches. Surveys and interviews can provide valuable insights into their daily tasks, challenges faced, and their contributions to various projects. Moreover, case studies highlighting successful data management initiatives can shed light on best practices.
Experimental Procedures
To observe the impact of RDMs in various settings, consider the following steps:
- Gather Data: Collect information from institutions utilizing RDM services.
- Survey RDMs: Utilize structured surveys to gain their perspectives on challenges and successes.
- Analyze Collaboration: Evaluate how improved access to data has influenced inter-departmental collaborations.
Ultimately, understanding the impact of Research Data Managers in modern scientific research demands a comprehensive approach that encompasses their multifaceted roles and the positive ripples they create across various disciplines. As academic and research institutions embrace these vital figures, they pave the way towards a more robust and efficient research ecosystem.
Understanding the Role of Research Data Managers
Research Data Managers play a crucial role in modern scientific research, acting as the bridge between complex datasets and the researchers who rely on them. As scientific inquiries become more intricate and data-driven, the significance of effective data management has never been more pronounced. This section aims to unpack what these managers actually do, illuminating their essential contributions to the overarching research process.
Defining Research Data Management
At its core, research data management involves the organization, storage, and sharing of data collected throughout a research project. It encompasses a myriad of best practices that help in handling data efficiently and ethically. So, when we talk about Research Data Management, we're not just imagining a librarian with a stack of papers. Rather, it’s an intricate dance of policies, tools, and collaborative duties designed to enhance the quality of research efforts.
The stakes can be quite high. Failing to manage data properly can lead to significant setbacks, including duplicated efforts or irretrievable information. Furthermore, data management practices lay down the framework needed for data sharing, which in our increasingly interconnected world is a vital part of academia.
Importance of Data Management in Research
Navigating the realm of scientific research without robust data management is like trying to sail a ship without a compass. Data management not only boosts the credibility of research findings but also enhances reproducibility. Here are a few key reasons why data management is so essential:
- Enhances Data Integrity: Proper management ensures that data is accurate and trustworthy. Consistency and reliability are the backbone of any research endeavor.
- Facilitates Collaboration: Research often requires interdisciplinary efforts, and having well-managed data makes it easier to collaborate across various fields. Managers synchronize datasets, ensuring all parties are on the same page.
- Promotes Accessibility: Open accessibility to data is becoming a norm in the research environment. Effective data management allows researchers to share information easily, thus broadening the reach of their findings.
- Supports compliance with regulations: By following established data management practices, researchers can ensure they are meeting legal and ethical requirements surrounding data use.
"In a world awash in information, effective data management is less about 'what you have' and more about 'how you use it.'"
In essence, the role of Research Data Managers is integral to the success of modern scientific research as they not only help streamline the data management process but also ensure that researchers can focus on their core objectives—pushing the boundaries of knowledge and innovation.
Key Responsibilities of Research Data Managers
Research Data Managers (RDMs) hold pivotal roles in the intricate tapestry of modern scientific research. Their key responsibilities are centered around ensuring that data is not just stored, but is also organized, secure, and ready for efficient use. In a world where research outputs heavily rely on data integrity and accessibility, understanding these core responsibilities helps to highlight the true impact RDMs have on scientific success.
Data Organization and Storage
At the heart of data management lies the fundamental task of organizing and storing research data. This responsibility goes far beyond merely saving files in designated folders. RDMs develop structured systems to categorize data, ensuring easy retrieval and optimal usability. This involves the creation of data management plans that specify how data will be collected, processed, and archived.
Consider a lab working on genomic research. Instead of dumping enormous data sets into a folder labeled "Genomics," an organized approach entails using specific labels, like "2019-Genome-Study-Results," coupled with relevant metadata descriptors detailing experiment parameters and data collection methods. This not only streamlines access to data but also conserves time – researchers can swiftly locate pertinent information without sifting through heaps of unorganized files.
Furthermore, choosing the right storage solutions is critical. RDMs assess various systems (cloud vs. local storage) based on the research's nature and sensitivity. The right choice often hinges on balancing accessibility with security, a key entrance into the next responsibility.
Ensuring Data Integrity and Security
Data integrity and security are vital in maintaining the trustworthiness of any research output. RDMs are responsible for implementing protocols that safeguard data from corruption, alteration, or unauthorized access. Regular audits of data systems are common practices to ensure that the data remains intact and reliable.
In recent years, there have been numerous incidents in which compromised data led to significant setbacks in research progress. Say a university research project is working with sensitive clinical data. RDMs must navigate the treacherous waters of compliance with laws such as HIPAA while also enforcing stringent security measures to protect participant confidentiality. This could include encryption, access controls, and secure file transfer protocols.
Moreover, RDMs often establish disaster recovery plans. They prepare contingencies for data loss scenarios, ensuring that backup procedures are in place to facilitate quick recovery. Thus, their role in assuring the integrity and security of data is not just about preventing issues – it's about trust that fuels the engine of scientific advancement.
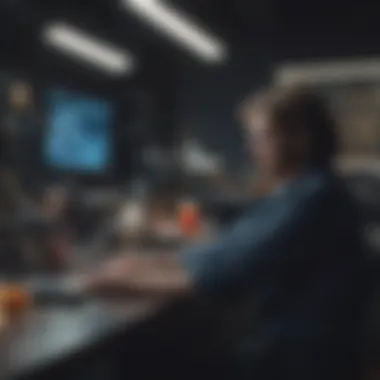
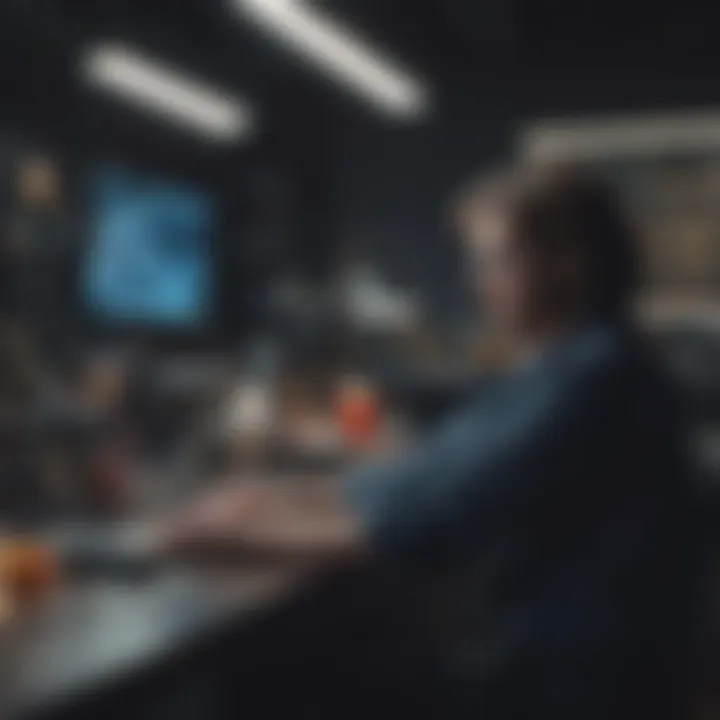
Facilitating Data Sharing and Accessibility
In the realm of research, data should not sit stale on a hard drive, but rather, should be accessible and shareable among researchers, institutions, and, when appropriate, the public. To this end, RDMs play an essential role in facilitating this sharing while maintaining usability.
This can mean developing user-friendly data repositories or platforms where datasets can be easily uploaded and accessed without hurdles. For example, RDMs might help initiate projects sharing findings on platforms like Figshare or Zenodo, enabling broader accessibility of valuable datasets.
It's a fine line, though; protecting sensitive data while still engaging in sharing is no small feat. RDMs often organize workshops and training for researchers on best practices for data sharing while encouraging adherence to licensing agreements, ensuring that the datasets are shared responsibly.
"The true value of research data lies not just in its collection, but in its accessibility to the wider research community."
By ensuring these key responsibilities are effectively executed, Research Data Managers fortify the backbone of scientific research, opening doors to collaboration and advancing the collective understanding of the world.
Technical Skills Required for Research Data Managers
The role of Research Data Managers (RDMs) is complex and multifaceted, requiring a specialized skill set to navigate the intricacies of modern scientific research. A solid foundation in technical skills is paramount for these professionals, as they serve as the linchpin between vast amounts of data and actionable insights. Having the right technical skills not only enhances their effectiveness but also ensures that research teams can rely on accurate data management to support their findings and enforce reproducibility. In this section, we explore the specific technical skills crucial for RDMs to excel in their roles.
Proficiency in Data Management Software
An RDM's ability to wield data management software is indispensable. This proficiency encompasses an array of tools and platforms tailored for research environments, such as REDCap, OpenClinica, or more generic solutions like Microsoft Access and Excel. Familiarity with these systems allows RDMs to organize data effectively, ensuring that researchers can access and manipulate it with ease.
Moreover, this skill aids in automating routine tasks, which in turn enhances efficiency. For instance, automated methods of data entry and retrieval can save countless hours, switching focus from mundane tasks to critical analysis. In essence, being adept with the right software not only enhances productivity but ultimately reinforces quality, as researchers can trust the data they are using.
Understanding of Metadata Standards
Knowing the ropes of metadata standards is another cornerstone of effective data management. Metadata provides the context needed to understand and interpret data accurately. For instance, without proper documentation, a dataset might be as useful as a paper boat in a storm. Established standards like Dublin Core or Data Documentation Initiative (DDI) provide a guide to codifying data elements systematically.
Having a grasp on these standards enables RDMs to ensure consistency across datasets. This is especially important when collaborating in interdisciplinary teams, where the risk of miscommunication or misinterpretation can run high. Moreover, metadata forms the backbone of data discoverability; it allows researchers to search for and access relevant data sets efficiently. A rich understanding of metadata not only improves data usability but enhances the integrity of the research process itself.
Knowledge of Statistical Analysis and Software
Finally, the capability to perform statistical analysis using specialized software is crucial for RDMs. Familiar tools such as R, SPSS, or SAS allow them to assist researchers in interpreting their data accurately. This skill set enables RDMs to bridge the gap between raw data and meaningful conclusions, which is vital in scientific inquiry.
By understanding the principles of statistical analysis, RDMs can provide valuable insights that guide researchers in their decisions based on data trends. Furthermore, this expertise can be beneficial when training researchers on how to analyze their findings critically. Hence, a solid grasp of statistical analysis not only reinforces data's trustworthiness but fosters a culture of meticulousness and precision within research teams.
In summation, the technical skills of Research Data Managers are more than just tools of the trade. They're essential for ensuring that data is effectively managed, preserved, and utilized wisely. By investing time and effort into mastering these specific skills, RDMs can substantially elevate the standard of research data management across diverse scientific fields.
Collaborative Practices in Research Data Management
In the realm of modern scientific research, collaboration is not just an added convenience; it’s the backbone of effective data management. Research Data Managers play a crucial role in fostering teamwork across disciplines, ensuring that data practices are not siloed but rather integrated and streamlined for everyone involved in the research process. The demand for effective data management arises not just from the data itself, but from the diverse sets of skills, expertise, and perspectives that each collaborator brings to the table. The dynamic nature of scientific research necessitates that Research Data Managers cultivate a collaborative environment where information can flow freely, and decisions can be made efficiently.
Interdisciplinary Team Collaborations
Interdisciplinary collaborations are becoming more commonplace as science recognizes that complex problems often require multiple viewpoints and areas of expertise. Research Data Managers are uniquely positioned to bridge gaps between disciplines. Their understanding of data management tools combined with their insight into various scientific domains enables them to ensure consistency and coherence in data practices.
For example, consider a research project that combines elements from biology, computer science, and social sciences to study the impact of climate change on human health. Each discipline may have its specific data format and analysis approach. Here, a Research Data Manager can facilitate the integration of these distinct data practices, promoting best practices in data collection and storage. This not only enhances the quality of the data but also allows for richer, multi-faceted insights that one discipline alone may not have uncovered.
Engagement with Researchers and Stakeholders
Active engagement with researchers and stakeholders is another vital aspect of the role of Research Data Managers. It's important that they work closely with scientists, IT specialists, and external partners to comprehend their needs fully. Understanding the unique objectives and challenges faced by each party helps in crafting data management strategies that are not only effective but also relevant.
Collaboration doesn’t merely stop at data management; it extends to the communication of data findings. By involving stakeholders early on, Research Data Managers can ensure that the data collected aligns with broader research goals. For instance, if a public health organization is involved in a study, their insights may shape how data is captured, ensuring that it meets public health standards and regulatory requirements. Solid communication nurtures trust and efficacy in partnerships, driving the overall success of the research initiative.
Training and Support for Researchers
Fostering a collaborative atmosphere also necessitates that Research Data Managers take on the responsibility of training and supporting researchers. This involves providing education on best data management practices, guidelines for ethical data usage, and resources on navigating regulatory frameworks. Researchers often juggle numerous responsibilities, and tailored workshops or training sessions can empower them to manage data more effectively.
Regular training sessions may cover pertinent subjects like proper data documentation, metadata standards, and the utilization of specific data management software tools. These educational efforts not only enhance individual researcher skills, but they also contribute to the development of a more competent and capable research environment as a whole. Further, the creation of easy-to-access support resources, such as written guides or instructional videos, can provide ongoing assistance, ensuring researchers can reference best practices as needed.
"Collaboration in data management is akin to jazz - it requires coordination, adaptability, and a willingness to harmonize distinct elements to create something truly impactful."
In summary, collaborative practices in research data management are integral to maximizing the impact of scientific research. By working across disciplinary lines, engaging actively with stakeholders, and providing comprehensive training to researchers, Research Data Managers lay the groundwork for a robust data management system that enhances data quality, integrity, and accessibility.
Challenges Faced by Research Data Managers
Research Data Managers navigate a labyrinth of obstacles that can complicate the effective handling of research data. These challenges not only influence the workflow but also impact the overall quality and trustworthiness of research outcomes. Understanding and addressing these challenges is crucial for optimizing data management practices. In this section, we’ll take a closer look at three significant hurdles: managing diverse data formats, addressing ethical concerns surrounding data usage, and adapting to constantly evolving technologies.
Dealing with Diverse Data Formats
In today’s research landscape, data takes on many shapes and sizes. From numerical spreadsheets to qualitative text and multimedia formats, the variety is truly mind-boggling. This diversity poses a significant challenge for Research Data Managers.
When research projects span multiple disciplines, the tools and methods for collecting data can greatly differ. For instance, a biologist might share genetic sequences in a proprietary format, while a social scientist could be handling survey data in a CSV file. The real conundrum lies in integrating these disparate formats without losing information or context.
Managing this variety calls for a well-thought-out strategy that may include:
- Standardization: Creating common formats or platforms that can accommodate differing types of data.
- Conversion Tools: Implementing software that can convert data into usable formats without compromising data fidelity.
- Training and Guidelines: Educating research teams on data format requirements can streamline future data collection efforts.
Navigating Ethical Concerns in Data Usage
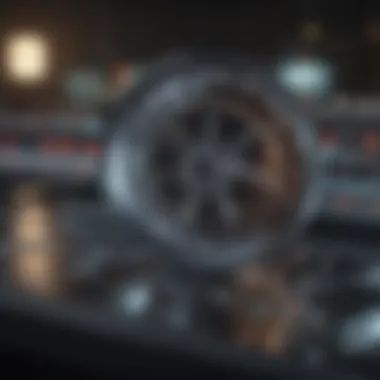
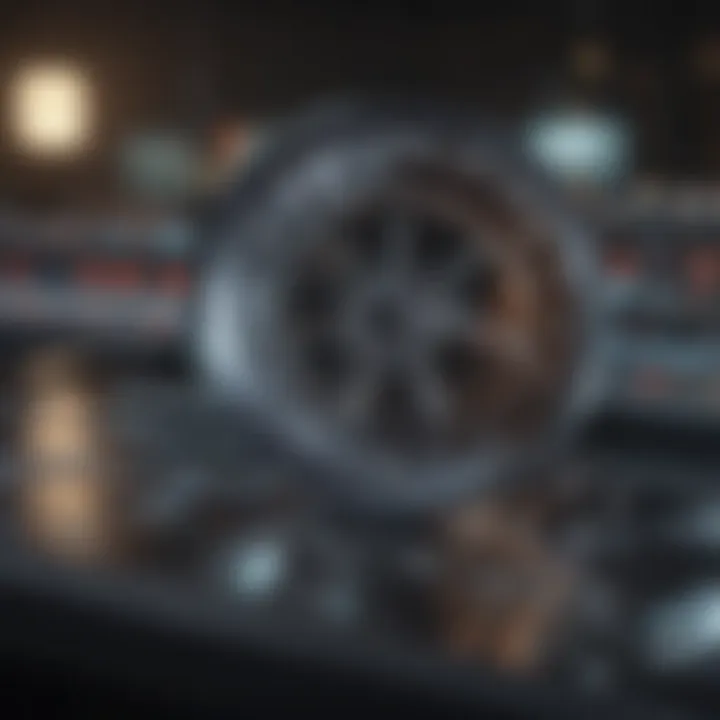
Ethical considerations in research data management have become an increasingly pressing issue. Researchers often face a muddle of regulations governing data privacy, informed consent, and the use of sensitive information. The stakes are high—missteps can lead to legal repercussions or erode public trust in scientific endeavors.
Research Data Managers play a critical role in ensuring that ethical standards are upheld. They must:
- Understand Regulations: Stay informed about national and international data protection laws, such as GDPR in Europe, that place stricter guidelines on data usage.
- Develop Ethical Frameworks: Work with researchers to establish clear protocols for data handling, emphasizing transparency and accountability.
- Conduct Regular Audits: Implement checks to ensure ongoing compliance with ethical guidelines.
Researchers and Data Managers alike must be vigilant in fostering an ethical culture within their institutions.
Keeping Up with Evolving Technologies
Technology is evolving at breakneck speed, and Research Data Managers must keep their finger on the pulse. New software, data storage solutions, and analytic techniques are always coming into play, making it essential to adapt quickly. The challenge is not only to adopt new technology but also to evaluate its relevance and efficacy.
- Continuous Learning: Managers must commit to lifelong learning, attending workshops, webinars, and conferences to stay updated on emerging trends.
- Assessing Impact: Implementing changes in technology should involve assessing how these tools can actually improve data management processes.
- Budgeting for Tools: Upgrading or adopting technology often has financial implications, so managers need to advocate for appropriate budget allocations.
"Only those who will risk going too far can possibly find out how far one can go." - T.S. Eliot
It’s crucial to embrace a mindset of innovation while scrolling through a sea of rapid technological advancements.
In summary, the challenges faced by Research Data Managers are numerous and complex. They must skillfully balance the demands of diverse data formats, ethical considerations, and ongoing technological advancements to ensure that research remains robust, reliable, and ethical.
The Future of Research Data Management
The future of research data management stands as a crucial focal point in the ongoing evolution of scientific inquiry. As the world becomes ever more interconnected and data-driven, the role of Research Data Managers is set to grow in complexity and importance. This article section will explore various elements impacting research data management including technological advancements, emerging open data initiatives, and shifts in policy frameworks. These changes not only promise to enhance the efficiency and integrity of data management but also elevate the overall quality of research output across disciplines.
Impact of Artificial Intelligence and Machine Learning
Artificial Intelligence (AI) and Machine Learning (ML) are reshaping how data is managed, analyzed, and utilized. Gone are the days when manual data entry and management techniques took precedence. Now, machines can assist in sorting through vast datasets, identifying patterns, and even predicting outcomes based on previously collected data.
- Benefits of AI and ML in Data Management:
- Increased efficiency in data processing
- Reduction in human error
- Enhanced capabilities in predictive analytics
- Facilitation of intelligent data retrieval
As researchers look to improve reproducibility, AI can play a significant role in ensuring data is structured and categorized according to best practices. This leads to a more systematic approach that not only increases accessibility but also fosters collaborative efforts among research teams.
Open Data Initiatives and Their Implications
Open data initiatives are crucial to the future landscape of research data management. By making data publicly available, researchers can promote transparency and foster a culture of trust and validation within the scientific community. Open data can lead to:
- Broader Reach and Collaboration:
- Quality-Centric Focus:
- Encourages innovative approaches by diverse research teams.
- Enables smaller institutions to participate in research initiatives.
- Standardizes data formats, making it easier to assess and utilize data.
- Promotes reproducibility in studies due to accessible methodologies.
However, challenges exist in navigating ethical issues around data ownership and privacy concerns. Managers will need to find a delicate balance between openness and ethical considerations as they guide their teams toward these initiatives.
Policy Developments in Research Data Management
As research continues to grow in scale and complexity, the attention on policies governing data management is more pressing than ever. Emerging regulations aim to standardize practices, ensuring data is handled in accordance with legal and ethical guidelines. Key policies that research data managers will need to watch include:
- Data Privacy Regulations:
- Funding Agency Mandates:
- Compliance with laws like GDPR to protect personal information.
- Creation of frameworks for responsibly managing sensitive data.
- Requirements for open-access data sharing to receive funding.
- Documentation and reporting practices that ensure accountability.
Given these trends, it’s crucial for research managers to stay abreast of policy changes and actively engage in conversations around best practices. The future of effective research data management, therefore, is an intricate interplay of technology, transparency, collaboration, and compliance.
"The evolution of research data management is not just a trend, but a deepened understanding of the value of information in our quest for scientific discovery."
As we move forward, embracing the shifts in technology, initiatives, and policies, Research Data Managers will play a pivotal role in guiding the field toward successful, accountable, and impactful research practices.
Research Data Management in Different Scientific Disciplines
Research data management is not a one-size-fits-all endeavor; rather, it adapts to the nuances of the specific scientific disciplines involved. Each field carries its own practices, challenges, and norms when it comes to data management. This section will delve into how various disciplines address research data management, outlining the significance of tailored strategies to maximize data integrity, accessibility, and utility.
Life Sciences Data Management Practices
In life sciences, data management practices are typically robust, reflecting the high stakes associated with biological research. Researchers often deal with complex datasets, stemming from experiments and clinical trials, which require systematic organization. The following elements characterize effective management of life sciences data:
- Standardized Protocols: Guidelines are essential to ensure that data is consistent across studies. Protocols help in maintaining reproducibility, which is vital for validating findings.
- Data Repositories: Life sciences often leverage specialized repositories such as GenBank or dbSNP that facilitate data sharing within the community. This sharing promotes collaboration and helps in building a collective knowledge base.
- Ethical Compliance: Managing sensitive data, especially human subjects’ data, demands adherence to ethical guidelines like the General Data Protection Regulation (GDPR). Ensuring confidentiality is paramount to preserve trust in research.
Life sciences data management thus requires not just technical skills but a deep understanding of ethical considerations and compliance frameworks, paving the way for groundbreaking advances in medical research and beyond.
Data Management in Environmental Sciences
Environmental sciences showcase a unique tapestry of data management challenges. Researchers in this field often gather disparate types of data that span various scales and sources, from satellite imagery to local field observations. Emphasis in data management here includes:
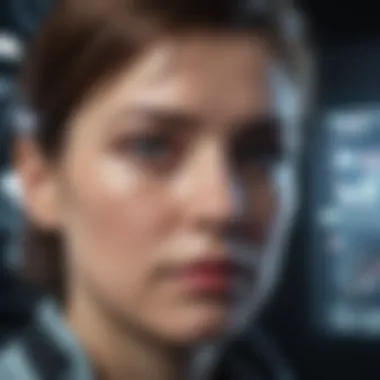
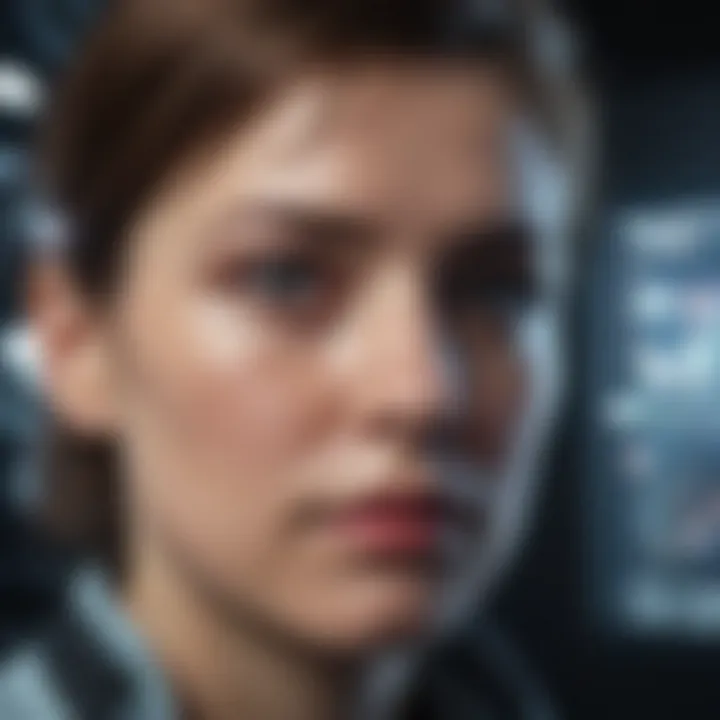
- Integrative Approaches: It's crucial to create frameworks that integrate data from different sources while keeping context intact. Several interdisciplinary collaborations attempt this, enriching the datasets with multiple perspectives.
- Tools for Visualization and Analysis: Environmental scientists often rely on Geographic Information Systems (GIS) and other tools for analyzing spatial data. Effective data management ensures that these tools are compatible with the datasets being handled.
- Long-term Monitoring: The environmental field heavily depends on longitudinal studies that track changes over time. Thus, creating effective data pipelines for maintaining historical data is critical to understanding trends and forming predictions.
Better data management practices in environmental sciences not only enhance scientific outcomes but also support informed decisions on policy and conservation efforts, illustrating the degree to which data influences real-world applications.
Challenges in Social Sciences Data Management
The social sciences present an array of distinct challenges when it comes to data management. Social scientists often deal with qualitative data, survey responses, and complex societal variables, each carrying idiosyncratic issues that affect data handling:
- Diverse Data Types: Unlike physical sciences, datasets can include interviews, open-ended surveys, and observational notes. The variability makes standardization complex, often requiring customized management approaches.
- Subjectivity in Data Interpretation: Qualitative data carries inherent biases and varied interpretations. Research Data Managers must focus on transparency in methodologies and coding schemes to ensure replicability of qualitative studies.
- Dynamic Data Environments: Social phenomena are often changeable, necessitating that data management strategies remain flexible and responsive to new findings and societal changes.
In sum, while challenges abound, they also reflect the rich, multifaceted nature of human interactions and behaviors. Social sciences data management, therefore, plays a crucial role in shaping impactful research and societal insights.
"Effective data management is the backbone of credible research, ensuring that findings are reproducible, reliable, and ultimately useful for advancing knowledge across disciplines."
In summarizing the nuances of research data management in different scientific domains, it is clear that understanding the context and specific requirements of each discipline is paramount. Addressing these unique needs results in more effective research, fostering environments where knowledge can thrive and impact can be maximized.
Evaluating Success in Research Data Management
Evaluating success in research data management is crucial for understanding the effectiveness of the tools, practices, and strategies employed by Research Data Managers (RDMs). Without measurable assurance, it’s tough to navigate how well data management contributes to the broader goals of research. This section outlines the significance of assessment and reveals how key metrics can inspire improvements and stimulate best practices in the realm of data management.
A multitude of elements come into play when we discuss evaluating success. RDMs focus on various components such as data integrity, sharing practices, and accessibility. The ultimate goal is to foster an environment where accessible and reliable data serves as the backbone of scientific discovery. Regular evaluations lead to identifying strengths and weaknesses, allowing teams to make data-driven decisions that enhance their work.
Metrics for Assessing Data Management Effectiveness
To effectively assess data management practices, RDMs employ several metrics that yield insights into various aspects of performance. These metrics can broadly include:
- Data Quality Indicators: Assessing the completeness, consistency, and accuracy of data is fundamental. Metrics might include percentage of missing data or the frequency of data errors found during audits.
- User Engagement Levels: Measuring how often data sets are accessed or downloaded gives insight into how researchers value the available data. High engagement can translate to successful data practices.
- Compliance with Standards: Assessing how well the data management practices align with established protocols, like FAIR (Findable, Accessible, Interoperable, and Reusable) principles, can indicate effectiveness in managing research outputs.
- Feedback Mechanisms: Gathering feedback from researchers utilizing the data helps in understanding areas that demand attention. Surveys and forms can be useful here.
"We can't improve what we do not measure. Metrics provide the light that guides us in the right direction."
Using these metrics, RDMs can hone their practices and foster a better research environment that prioritizes integrity and collaboration.
Case Studies of Successful Data Management
Real-world examples often speak louder than theories when it comes to demonstrating success. Looking into specific case studies sheds light on how effective data management transforms research practices and fosters collaboration.
One noteworthy case is that of the Global Biodiversity Information Facility. This organization emphasizes data accessibility and interoperability. Through their structured data management approaches, biodiversity datasets from around the world can be easily accessed by researchers, resulting in increased cooperation across various ecological disciplines. Their successes are measured through metrics indicating increased downloads and collaborations, bolstered by formal feedback from the research community.
Another notable example involves NASA. They launched a successful data management initiative involving remote sensing data. By employing advanced tools and metrics for data sharing, they ensured that datasets were compliant with international data sharing agreements. As an outcome, this initiative boosted the overall transparency and reproducibility of scientific studies conducted on climate change.
These case studies not only illustrate the positive impacts of sound research data management but also provide context on how evaluations can help in refining these practices. As students, researchers, and educators take note, they will realize that assessing and showcasing success in research data management is not just an academic exercise, but a vital part of advancing science as a whole.
The Role of Research Data Management in Enhancing Reproducibility
Reproducibility is often hailed as a cornerstone of scientific research. When researchers can replicate findings using the same data and methods, it reinforces the validity of an experiment and solidifies its conclusions. The role of Research Data Managers (RDMs) in promoting reproducibility is increasingly critical as studies grow in complexity and data volumes skyrocket. Effective data management practices not only aid researchers in organizing their datasets but also ensure that their work can stand the test of replication.
RDMs contribute significantly to minimizing discrepancies in research outcomes. They accomplish this through robust data management strategies that promote a culture of transparency and trust within the scientific community. Enhancing reproducibility isn’t merely about generating the same results; it’s about creating a reliable framework through which data can be managed, shared, and utilized effectively in future studies.
Importance of Transparency in Data Practices
Transparency in data practices means that researchers openly share their methodologies, datasets, and any modifications. This openness enables other scientists to scrutinize their work, test the results, and build upon existing research. RDMs play a pivotal role in fostering this transparency. They help in documenting data management processes and creating user-friendly data repositories.
- Maintaining clear documentation aids in understanding data provenance.
- Ensuring datasets are accurately described using standardized metadata increases their discoverability.
- Organizing data in a way that is comprehensive and intuitive facilitates easy access for others who wish to replicate or validate findings.
By prioritizing transparency, RDMs help to create an environment where researchers can engage in constructive dialogues about methodologies, thus elevating the overall scientific discourse.
"Transparency serves as the bedrock for reproducibility. Without it, trust in scientific findings is compromised."
Strategies for Increasing Reproducibility through Data Management
RDMs employ various strategies to bolster the reproducibility of scientific findings. These strategies are designed to streamline data processes and ensure that all necessary information is readily available for those looking to replicate results.
- Centralized Data Repositories: Establishing centralized repositories allows for uniform data access. When all research data is stored in one place, researchers can easily obtain the information they need.
- Version Control Systems: Implementing version control ensures that all changes to datasets are tracked. This not only helps in preserving the integrity of the data but also allows researchers to revert back to previous iterations if necessary.
- Standardized Protocols: Developing and promoting the use of standardized protocols for data collection and analysis can reduce variability in methodologies among researchers. Such consistency is crucial for replicating studies accurately.
- Training Workshops: Offering training for researchers on best practices in data management can cultivate a shared understanding of the importance of reproducibility. This builds a more conscientious approach to data handling across disciplines.
- Regular Audits: Conducting regular audits of data management practices allows institutions to identify areas for improvement and ensure adherence to established guidelines.
Through these strategies, RDMs not only facilitate easier access to data but also nurture a scientific culture that values reproducibility and transparency. This ultimately enhances the credibility of research outcomes and the scientific enterprise as a whole.
End and Future Directions
The evolution of scientific research increasingly hinges on effective research data management. In this landscape, Research Data Managers stand as key players, ensuring that the massive influx of data is organized, accessible, and secure. Their role cannot be overstated; they not only facilitate the practical aspects of data handling but also play a crucial part in enhancing the credibility and transparency of research outcomes. As we delve into the concluding thoughts of this article, several critical elements emerge that exemplify the significance of effective data management practices in a modern research environment.
In summary, the contributions of Research Data Managers stretch far beyond mere organization of data. They actively foster a culture of collaboration among researchers, streamline communication, and ensure compliance with ethical standards. This article highlighted how their responsibilities intertwine to support the overarching goals of reproducibility and transparency in scientific inquiry. Additionally, the future directions for these roles seem promising and challenging at the same time. Emerging technologies and shifting policies will continuously reshape their landscape, demanding adaptability and foresight.
Summarizing Key Takeaways
- Crucial Role in Research Quality: Research Data Managers directly contribute to improving data integrity, thereby elevating the quality and reliability of research outcomes.
- Promoting Interdisciplinary Collaboration: By managing data across various scientific disciplines, they encourage researchers to work together, enriching the scope and applicability of research.
- Navigating Ethical Concerns: These managers play a pivotal role in addressing ethical concerns surrounding data usage, ensuring that research complies with prevailing standards.
- Adaptability to Technology Trends: Staying abreast of evolving technologies enables them to implement the best tools for data management, preparing for future challenges in the field.
- Focus on Open Data Initiatives: By promoting open data practices, they enhance the accessibility of datasets, thereby accelerating scientific discovery and innovation.
Future Trends in Research Data Management
The journey ahead for Research Data Managers is poised for transformation, driven by several key trends:
- Artificial Intelligence Integration: The incorporation of AI tools into data management practices is making it easier to sort, analyze, and manage data. This trend not only streamlines workflows but also enhances decision-making processes.
- Emphasis on Open Science: The push for open science will continue, making it imperative for Research Data Managers to adapt to policies and technologies encouraging data sharing and accessibility.
- Policy Evolution: As the landscape of research changes, relevant policies must also evolve to govern data management practices. This warrants that Research Data Managers stay informed and actively participate in shaping these policies.
- Cloud Technologies: The rise of cloud computing transforms data storage and processing capabilities, offering flexibility and efficiency in data management that was previously unattainable.
- Data Stewardship Roles: As institutions recognize the value of effective data management, there will be a growing demand for specialized roles within research teams dedicated solely to data stewardship.
Through these considerations, the role of Research Data Managers not only remains essential but is also likely to expand, becoming increasingly integral to the research process itself. The integration of evolving technologies, coupled with a growing emphasis on ethical practices, positions these professionals to significantly enhance the quality of scientific research in the years to come.