RNA-Seq Data Analysis Course: A Comprehensive Overview
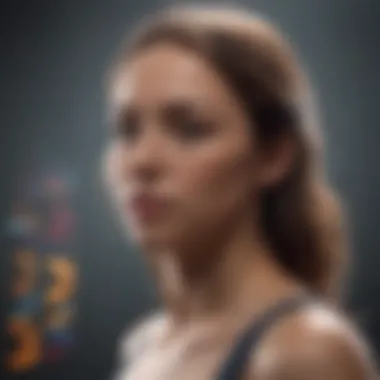
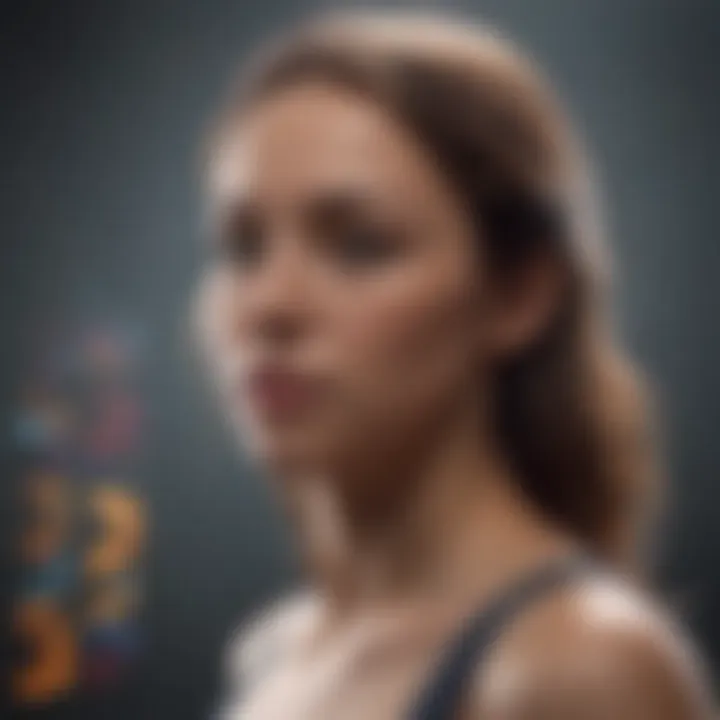
Intro
In an era where genomic technologies advance rapidly, understanding RNA-Seq data analysis is vital for anyone involved in biological research. This comprehensive course aims to equip learners with the necessary tools and methodologies to effectively tackle RNA-Seq data. By focusing on both theoretical foundations and practical skills, the course is designed for a diverse audience, including students, researchers, and professionals.
Through a structured framework, the course addresses key aspects of RNA-Seq data analysis, such as experimental design, data preprocessing, statistical methods, and result interpretation. The aim is to foster a profound understanding that empowers participants to navigate the complex world of genomics confidently.
Research Highlights
Key Findings
The analysis of RNA-Seq data yields numerous insights about gene expression patterns. Learners will discover:
- The ability to identify differentially expressed genes.
- Insights into splicing variations and alternative transcripts.
- The impact of various experimental conditions on RNA profiles.
These findings can significantly influence how researchers approach their hypotheses and experimental designs.
Implications and Applications
Understanding RNA-Seq data extends beyond academic curiosity. It has practical applications in diverse fields:
- Biomedical research: Identifying biomarkers for diseases.
- Pharmacogenomics: Tailoring therapeutic strategies for individuals.
- Agricultural sciences: Improving crop resilience through gene expression studies.
The implications of RNA-Seq extend far and wide, affecting multiple domains where gene expression analysis is critical.
Methodology Overview
Research Design
A robust research design is crucial for the success of RNA-Seq studies. The course emphasizes:
- Clear objectives: Establishing what the analysis aims to achieve.
- Proper controls: Integrating sufficient replicates for statistical relevance.
- Sample selection: Ensuring representative sampling to generalize findings.
These elements form the backbone of effective RNA-Seq analysis, guiding learners in their experimental procedures.
Experimental Procedures
Delving into the technical aspects, the course covers various experimental procedures fundamental to RNA-Seq:
- RNA extraction methods.
- Library preparation techniques.
- Sequencing technologies and platforms, such as Illumina and Oxford Nanopore.
Each of these procedures has specific protocols that impact the quality and interpretability of data. Understanding these can enhance the reliability of subsequent analyses.
"RNA-Seq has revolutionized the way we approach genomic research, allowing for rich insights into gene expression that were once unimaginable."
As learners progress through the course, they will grasp the intricate details of RNA-Seq, enabling them to apply their knowledge effectively in real-world scenarios.
Prologue to RNA Sequencing
RNA sequencing, often referred to as RNA-Seq, has revolutionized the field of genomics, providing insights into the dynamic world of gene expression. Understanding RNA-Seq is essential for those involved in modern biological research, medicine, and biotechnology. This introduction will cover the significance of RNA sequencing and its foundational role in various scientific investigations.
Definition and Importance
RNA sequencing is a next-generation sequencing (NGS) strategy that allows for the quantification of RNA levels in a biological sample. This technique not only provides data on gene expression but also gives insights into alternative splicing, post-transcriptional modifications, and non-coding RNA dynamics. The importance of RNA-Seq lies in its capacity to analyze the transcriptome comprehensively, thereby enabling researchers to dive deeper into the complexities of gene regulation and biological function.
Key benefits of RNA sequencing include:
- High-throughput capability: RNA-Seq can simultaneously analyze thousands of genes, making it efficient for large-scale studies.
- Increased sensitivity: It can detect low-abundance transcripts that are often missed by traditional methods like qPCR.
- Discovery of novel transcripts: RNA-Seq is adept at discovering previously unannotated transcripts, providing a broader scope of investigation.
Historical Context
The journey of RNA sequencing began with the invention of Sanger sequencing in the 1970s, which laid the groundwork for later advancements. However, it was not until the early 2000s that RNA-Seq emerged as a viable method for transcriptomics. The major breakthrough came with the advent of next-generation sequencing technologies, which dramatically reduced the cost and time required for sequencing.
The first applications of RNA-Seq were primarily in model organisms, such as Saccharomyces cerevisiae and Drosophila melanogaster, where it demonstrated superiority over microarray technologies. By providing a more comprehensive view of the transcriptome, it opened doors for investigating complex diseases, evolution, and even developmental biology.
In summary, RNA sequencing is a critical technique in genomics research and offers a myriad of applications across biological disciplines. Understanding its definition, significance, and historical development prepares the foundation for the subsequent sections of this course.
Fundamentals of RNA-Seq Technology
Understanding the basics of RNA-Seq technology is crucial for anyone engaged in genomic research or analysis. This section sets the groundwork for comprehending how RNA sequencing operates and why it is pivotal in modern biology. With the exponential growth of RNA-Seq applications, getting familiar with the fundamental elements can aid researchers in designing experiments and interpreting results accurately.
Overview of Next-Generation Sequencing
In the context of RNA-Seq, next-generation sequencing (NGS) encompasses a group of advanced sequencing technologies that enable rapid and efficient sequencing of RNA. These technologies have revolutionized genomics by allowing researchers to capture the complexity of transcriptomes in a parallel manner. NGS facilitates high-throughput studies which means millions of sequences can be generated in a single run compared to the older Sanger sequencing method. This efficiency significantly reduces costs and speeds up the process of data collection.
Key aspects of NGS relevant to RNA-Seq include:
- High Throughput: The ability to sequence many samples simultaneously, leading to comprehensive data sets.
- Increased Accuracy: Advanced technologies offer improved accuracy in detecting variations in RNA expression levels.
- Cost-Effectiveness: The declining costs of sequencing make RNA-Seq more accessible for various research purposes.
Library Preparation Methods
Library preparation is a critical step in RNA-Seq where RNA is converted into complementary DNA (cDNA) and prepared for sequencing. The quality of the library preparation directly impacts the outcome of the sequencing results. Different methods exist, primarily focusing on various types of RNA such as total RNA or mRNA.
Common library preparation methods include:
- Poly(A) Selection: Enriches mRNA by capturing only polyadenylated transcripts.
- rRNA Depletion: Removes ribosomal RNA, thus enriching the sample for mRNA or other non-coding RNAs.
- Strand-Specific Library Preparation: Retains strand information of the RNA, which is important for certain analyses.
These techniques must be chosen based on the specific goals of the experiment. A well-prepared library enhances the quality of the sequencing data received.
Sequencing Platforms Comparison
There are several sequencing platforms available for RNA-Seq, each with unique features, advantages, and limitations. Choosing the right platform is essential for achieving the desired results.
Some popular platforms used for RNA-Seq include:
- Illumina Technology: Known for its high throughput and relatively low cost. Ideal for large-scale studies.
- Ion Torrent: Offers faster run times and user-friendly workflows, but potentially higher error rates in homopolymeric regions.
- Pacific Biosciences (PacBio): Provides long-read sequencing which is beneficial for resolving complex regions of the transcriptome such as splicing variations.
The choice of sequencing platform should align with research objectives, budget, and the type of data desired. The right selection ensures that the study yields meaningful insights and high-quality data.
In summary, the fundamentals of RNA-Seq technology encompass the foundational knowledge required to navigate the complexities of RNA analysis. A thorough understanding of NGS, library preparation methods, and sequencing platforms provides the necessary context for effective experimental design and data interpretation in RNA-Seq studies.
Experimental Design Considerations
Experimental design is a crucial aspect of RNA-Seq data analysis, significantly influencing the reliability and validity of the findings. This section delves into important factors and methodologies necessary for designing effective RNA-Seq experiments. A well-crafted experimental design maximizes the chances of obtaining high-quality data that answers the posed biological questions. The primary considerations in this context include the choice of design, control of variables, and determination of sample size.
Choosing the Right Design
Selecting the right experimental design is pivotal for successful RNA-Seq analysis. Different design strategies, such as paired designs, crossover studies, or completely randomized designs, serve distinct purposes. Each approach can lead to varying degrees of statistical power and bias reduction.
In RNA-Seq studies, one needs to consider factors like:
- Objective of the study: Whether the aim is to compare gene expression across conditions or time points will influence the chosen design.
- Nature of the samples: Biological variability between samples can dictate the most suitable design. For example, if investigating responses to a treatment, a paired design might be preferred to control for inter-individual variability.
- Budget and resources: Practical constraints may limit the extent of replication or the number of comparisons.
Taking these factors into account helps in optimizing the experimental design, ultimately leading to clearer and more interpretable results.
Controlling Variables and Replication
To derive accurate conclusions from RNA-Seq data, it is vital to control variables that might skew results. These variables can include environmental factors, biological differences between samples, handling procedures, and technology used for sequencing. Any uncontrolled variability can increase noise in data interpretation, undermining the results.
Replication is another core principle in experimental design. Replicating samples enhances reliability and statistical power. There are two main types of replication:
- Technical Replication: Refers to repeating the experiment using the same biological sample to account for technical variability.
- Biological Replication: Involves collecting multiple independent samples to capture biological diversity.
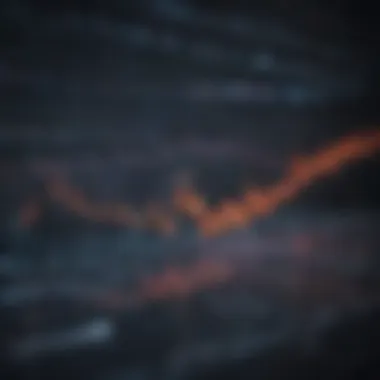
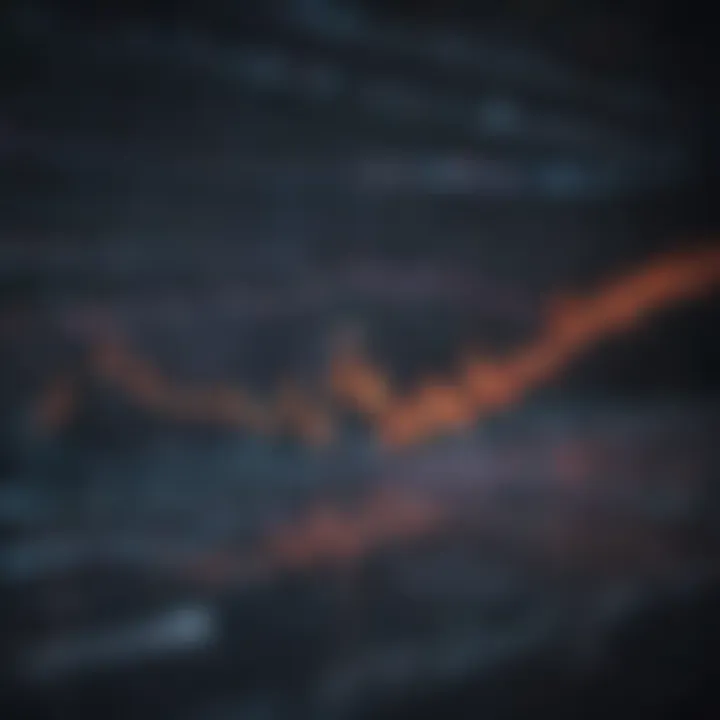
Striking the right balance between these types of replication is essential, as it contributes to the robustness of findings.
Sample Size Determination
Calculating an appropriate sample size is critical for ensuring reliable statistical analysis in RNA-Seq studies. An inadequately small sample size can lead to false negatives, where differences in gene expression might go undetected due to insufficient statistical power. Conversely, a sample size that is excessively large may waste resources and increase experimental costs without substantial benefits.
When determining sample size, several factors should be considered, including:
- Effect Size: The anticipated magnitude of differences in gene expression.
- Variability within samples: Greater variability generally necessitates a larger sample size.
- Desired power of the analysis: Typically, a power of 80% is accepted in many scientific studies, meaning there is an 80% chance of detecting an effect if it exists.
Employing statistical power analysis can provide a framework for calculating the ideal sample size for RNA-Seq experiments.
Important takeaway: Thoughtful experimental design prepares the foundation for successful RNA-Seq data analysis. It ensures that meaningful biological insights are derived from rigorous statistical interpretations. By considering the design, controlling variables, and determining sample sizes carefully, researchers can enhance the quality and reliability of their results.
Data Acquisition and Processing
The steps involved in data acquisition and processing are essential in RNA-Seq data analysis. By understanding these processes, researchers obtain high-quality data that is crucial for reliable results. Each stage influences subsequent analysis, thus comprehension of its intricacies is vital for any RNA-Seq study.
Data Generation from Sequencing
This step refers to the actual production of RNA-Seq data through sequencing technologies. Next-generation sequencing (NGS) platforms, like Illumina and Ion Torrent, convert RNA samples into sequence data.
The generation process begins with the conversion of mRNA into complementary DNA (cDNA) using reverse transcription. Then, sequencing libraries are prepared, often involving fragmentation and adapter ligation. Once libraries are constructed, they are loaded onto the sequencing machines.
The raw data produced consists of numerous sequence reads. These reads, however, are not yet useful without further processing.
Preprocessing Steps
Before any analysis, the preprocessing steps help in cleaning and preparing the data. Preprocessing generally involves several tasks:
- Trimming: This step removes low-quality bases from the ends of reads and cuts adapter sequences.
- Filtering: Reads that do not meet quality standards are discarded.
- Alignment Preparation: The processed reads are organized for alignment against a reference genome or transcriptome.
By conducting these steps carefully, the integrity of the data can be preserved. This is crucial for obtaining valid results later in the analysis.
Quality Control of RNA-Seq Data
Quality control (QC) is a critical phase in RNA-Seq data analysis. It ensures that the data obtained from sequencing is of acceptable quality before progressing to more advanced analyses. The following aspects are often monitored:
- Read Quality Scores: Tools like FastQC can help visualize quality metrics.
- Mapping Rate: The percentage of reads that successfully align to the reference genome is often reviewed.
- Duplication Rates: High duplication rates can indicate potential issues in library preparation.
Maintaining stringent QC standards minimizes erroneous interpretations and increases reproducibility of findings. Researchers should utilize tools such as MultiQC to combine quality reports from various steps and create comprehensive summaries for easier review.
"Proper data acquisition and a rigorous processing framework lay the cornerstone for high-quality RNA-Seq analysis.
Alignment of RNA-Seq Reads
The alignment of RNA-Seq reads is a critical process in the analysis pipeline. This step ensures that the short sequences generated from sequencing are matched accurately to a reference genome or transcriptome. Proper alignment enables researchers to examine gene expression levels, identify novel transcripts, and detect potential genetic variants. The importance of alignment cannot be overstated, as the accuracy of subsequent analyses is highly dependent on how well reads are aligned.
Prelude to Read Alignment
Read alignment involves mapping the sequenced RNA reads back to a reference genome. This is crucial for several reasons. Firstly, it allows researchers to determine where in the genome a particular read originates. Secondly, it helps to reconstruct full-length transcripts. There are multiple tools and algorithms available for this task, including Bowtie, STAR, and HISAT2. Each tool has its strengths and weaknesses, which may influence the choice depending on the specifics of the dataset and the research goals.
Characteristics to consider include speed, memory usage, and the ability to handle unique versus multi-mapping reads. For optimal results, understanding the data and the biological question at hand are essential.
Selecting an Alignment Tool
Choosing the right alignment tool is pivotal to the success of RNA-Seq analysis. The three major factors driving this choice are the type of sequences generated, the computational resources available, and the overall research objectives.
- Speed of Alignment: Tools like Bowtie are known for their fast alignments, suitable for large datasets.
- Sensitivity: STAR and HISAT2 are often favored for their ability to handle spliced alignments, making them ideal for eukaryotic genomes where genes may be interrupted by introns.
- Output Formats: Certain tools produce outputs better suited for downstream analysis, so familiarity with these formats can guide selection.
"Selecting the right tool is more than a technical choice; it reflects an understanding of the research landscape."
Ultimately, the right tool can significantly streamline the RNA-Seq analysis workflow and improve the reliability of results, making this decision paramount.
Post-Alignment Processing
After alignment, post-processing steps are critical to refine the results. This stage involves several key processes that enhance the quality and utility of aligned data:
- Removing Duplicates: PCR duplicates can skew results, thus removing them is essential for accurate quantification of expression levels.
- Quality Filtering: This involves assessing the alignments for errors, ensuring only high-quality reads are considered for analysis.
- Conversion and Normalization: Converting alignment files into formats like BAM or SAM and normalizing data are also important steps.
Each of these processing steps contributes to the integrity of the data. Ignoring them can lead to inaccurate interpretations, which can ultimately compromise the studyโs conclusions.
In summary, the alignment of RNA-Seq reads is a foundational aspect of RNA sequencing analysis. It sets the stage for accurate interpretation of gene expression data, making it essential for any researcher working in genomics.
Quantification of Gene Expression
Quantification of gene expression is a crucial aspect of RNA-Seq data analysis. It involves measuring the amount of RNA produced by genes under specific conditions. By quantifying gene expression, researchers can understand gene activity and its variation in different samples. This information is vital for exploring cellular functions and understanding biological processes.
Understanding Expression Levels
The expression level of a gene refers to the quantity of its RNA transcript present in a sample. This level can offer insights into how genes respond to various stimuli and environmental conditions. For example, a high expression level often correlates with active biological processes related to growth or stress responses.
It is essential to not only quantify the raw read counts but also to interpret what these levels indicate about biological functions. Each gene's expression may vary widely, influenced by several factors, including tissue type and treatment conditions.
Normalization Techniques
Normalization is key in RNA-Seq data analysis. Given that different samples may produce varying numbers of reads due to technical variations, normalization ensures that these differences do not affect the conclusions drawn from the data. There are several normalization techniques:
- Total Count Normalization: This technique scales counts by the total number of reads, providing a simple ratio.
- Quantile Normalization: It adjusts the distribution of gene expression levels across samples to make them comparable.
- TMM (Trimmed Mean of M-values): This method accounts for composition biases in the libraries, enhancing the comparability of samples.
Choosing the right normalization method is critical as it significantly impacts downstream analyses and interpretations.
Common Quantification Methods
Various methods are available for quantifying gene expression from RNA-Seq data. Some common ones include:
- HTSeq: A popular tool that counts reads mapped to each gene, providing precise read counts.
- featureCounts: Similar to HTSeq, it is widely used for counting reads and offers better speed and scalability.
- Salmon: A tool for quantifying transcript expression using a lighter, faster approach.
Each method has its strengths and considerations, and the choice depends on specific research objectives and experimental designs.
"The choice of quantification method can significantly influence the statistical models used and, ultimately, our interpretations of gene functions."
In sum, understanding and quantifying gene expression levels is vital in the RNA-Seq landscape. Proper techniques and methods chosen can lead to robust biological insights.
Statistical Analysis of RNA-Seq Data
Statistical analysis is a fundamental aspect of RNA-Seq data analysis. It allows researchers to derive meaningful insights from raw data generated through sequencing. The large volume of data produced in RNA-Seq experiments presents unique challenges. Appropriate statistical methods are vital for interpreting this data and ensuring findings are reliable and accurate. With suitable statistical approaches, one can differentiate true biological signals from noise, which is crucial in genomics.
Statistical analysis helps in various ways, including:
- Identifying significant gene expression changes: This is key when assessing the effects of different conditions or treatments.
- Controlling for confounding variables: It ensures that the conclusions drawn are valid and not influenced by unintended factors.
- Enhance reproducibility of results: Robust statistical methods contribute to repeatability, reinforcing the credibility of scientific research.
Understanding the statistical framework empowers students and professionals alike. Thus, analyzing RNA-Seq data is not just about retrieving information but understanding the underlying statistics that validate discoveries.
Statistical Methods Overview
The landscape of statistical methods utilized in RNA-Seq analysis is varied. It encompasses techniques designed to handle the specific challenges posed by count data, such as low counts for some genes and high counts for others. Common statistical methods employed include:
- Negative Binomial distribution: This method is often used to model the overdispersed count data typical of RNA-Seq.
- Poisson distribution: An initial approach before acknowledging overdispersion.
- Bayesian methods: Provide flexible frameworks that incorporate prior knowledge, useful for small datasets.
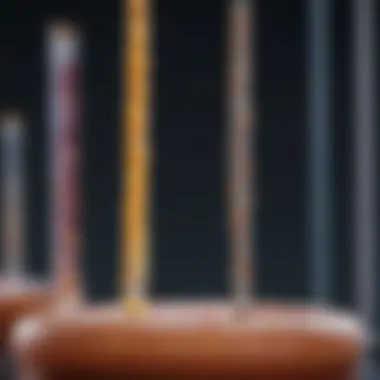
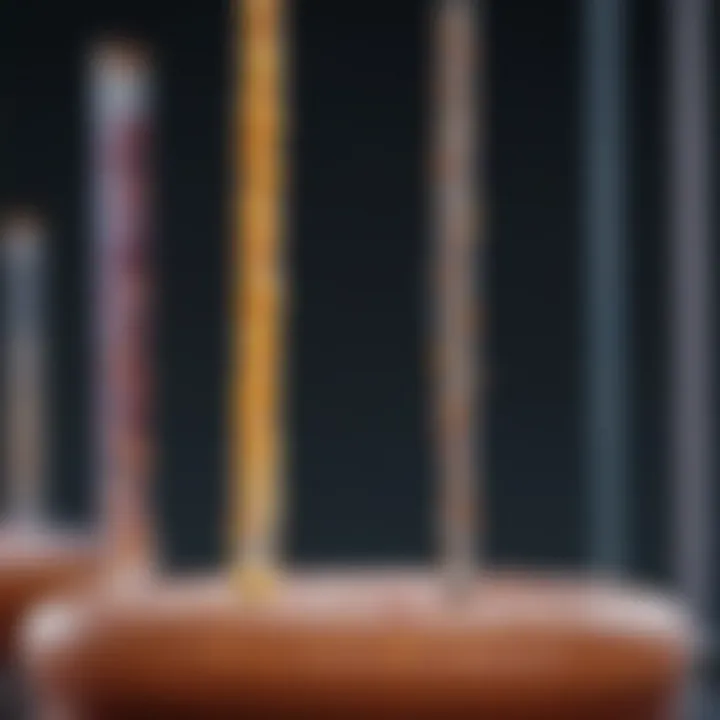
Each of these methods has its strengths and weaknesses. Understanding when and how to apply them is critical for achieving accurate results.
Generalized Linear Models
Generalized Linear Models (GLMs) extend traditional linear regression techniques to handle non-normal response variables, which is essential for RNA-Seq data. The structure of GLMs allows for different distributions, including the Negative Binomial and Poisson distributions mentioned earlier. This flexibility enables researchers to model gene count data effectively.
GLMs benefit RNA-Seq analysis in several ways:
- Flexibility: They can accommodate various link functions, permitting the modeling of different types of responses.
- Interpretation of effects: The framework makes it easier to interpret coefficients in terms of relative risk or differences in counts.
- Adjusting for covariates: Researchers can include multiple factors, ensuring a more accurate analysis of gene expression.
Using GLMs enhances the reliability of conclusions drawn from RNA-Seq datasets.
Multiple Testing Corrections
Multiple testing is a major concern when analyzing RNA-Seq data. Given the large number of genes assessed simultaneously, the risk of false positives increases significantly. As a result, applying corrections for multiple comparisons is essential. Common methods for this include:
- Bonferroni correction: A straightforward approach that adjusts the significance threshold based on the number of tests performed.
- False Discovery Rate (FDR): This method balances the identification of true positives while controlling the expected proportion of false discoveries. The Benjamini-Hochberg procedure is often employed for this.
Applying these corrections helps mitigate errors, ensuring that the identified differentially expressed genes are genuinely significant and biologically relevant.
In summary, statistical analysis is instrumental in RNA-Seq research. By employing appropriate methods, researchers can ensure valid inferences and significantly advance our understanding of gene expression.
Differential Gene Expression Analysis
Differential gene expression analysis is a crucial component of RNA-sequencing studies. This analysis allows researchers to identify genes that show significant differences in expression levels between different biological conditions, such as healthy versus diseased tissues. Understanding these differences can offer insights into the underlying biological processes and mechanisms that contribute to disease or other phenotypic variations.
This analysis can be applied in various contexts, which include, but are not limited to, cancer research, developmental biology, and drug response evaluations. By identifying differentially expressed genes (DEGs), researchers can pinpoint potential biomarkers for diseases or targets for therapeutic interventions.
Identifying Differentially Expressed Genes
Identifying DEGs involves several statistical approaches and bioinformatics tools. These tools help compare expression levels across experimental conditions. Commonly used methods include statistical tests such as the t-test, ANOVA, and various modeling approaches like linear models. Notable software includes DESeq2 and edgeR.
- Normalization is a critical first step. It adjusts for factors like library size and composition.
- Following normalization, statistical comparisons between groups are performed.
- The output usually includes a list of genes with associated p-values and fold changes. Selecting appropriate thresholds can enhance the reliability of detected genes.
"Differential expression analysis provides valuable insights into the functional aspects of gene regulation."
Interpreting Results
Properly interpreting the results of differential gene expression requires careful consideration. After obtaining a list of DEGs, it is essential to assess their biological relevance. Researchers often employ gene ontology (GO) enrichment analysis, which helps in understanding the functional implications of these genes. Additionally, pathway analysis can reveal whether the DEGs are tied to specific biological pathways or processes.
Important questions to consider include:
- What are the roles of identified genes in the biological context?
- Are there consistent patterns of expression across different samples or conditions?
- How do these DEGs relate to previous findings in the field?
Visualizing Differential Expression
Visualization plays a significant role in presenting differential expression results effectively. Various plotting techniques can help in conveying complex data in a more digestible form. Common visualizations include:
- Volcano plots: They show significance versus fold change, allowing researchers to easily identify notable genes.
- Heatmaps: They provide a visual summary of expression data across samples, highlighting clusters of co-expressed genes.
- Box plots: Useful for showcasing expression distribution between groups for specific genes.
Using visualization tools like ggplot2 or heatmap.2 can facilitate clear and informative presentations of the data. This approach not only aids in understanding the results but also assists in communicating findings to diverse audiences.
Functional Analysis of Gene Sets
Functional analysis of gene sets serves a crucial purpose in understanding biological mechanisms. This process enhances the interpretation of large-scale RNA-Seq data results. By focusing on groups of genes, it allows researchers to infer biological significance from their expression patterns. This portion of the RNA-Seq data analysis course will detail methodologies and tools necessary for performing effective functional analysis.
Gene Ontology Analysis
Gene ontology analysis provides a framework for describing the roles of genes across various biological contexts. It categorizes genes into three main domains: biological processes, cellular components, and molecular functions. Utilizing databases like the Gene Ontology Consortium, researchers can assign functions to gene lists derived from differential expression analyses. This assists in highlighting overarching biological themes in the data.
Benefits of Gene Ontology Analysis:
- Enhanced understanding of biological functions associated with genes.
- Comparison across studies through standardized classifications.
- Identification of biological pathways potentially affected by experimental conditions.
To perform a gene ontology analysis, tools such as DAVID or GSEA can be employed. These platforms support various input formats and offer detailed result visualization. A typical workflow consists of:
- Collecting the list of differentially expressed genes.
- Uploading the gene list to the analysis tool.
- Selecting the appropriate parameters for background gene sets.
- Interpreting the results focusing on enriched terms and pathways.
Pathway Analysis Techniques
Pathway analysis techniques evaluate the functional interactions among genes and their roles in biological pathways. Understanding these pathways is essential for grasping how gene expression changes may influence cellular functions or disease processes. Pathway databases like KEGG and Reactome provide comprehensive resources for identifying involved pathways based on gene expression data.
Key considerations for pathway analysis include:
- Selection of relevant pathways based on experimental context.
- Integration of other omics data, such as proteomics.
- Adjustments for multiple testing to ensure robust conclusions.
Tools like Pathway Studio or Ingenuity Pathway Analysis are frequently used for these analyses. The process typically involves:
- Inputting the gene expression data.
- Linking genes to known pathways within the database.
- Running the analysis to identify significant pathways.
- Visualizing results to interpret biological implications.
Employing functional analysis methods is essential for extracting biological meaning from RNA-Seq data. The insights gained can drive further research and inform on the complexities of biological systems.
Challenges in RNA-Seq Data Analysis
RNA-Seq data analysis is a complex process laden with several challenges that must be understood and mitigated effectively. Identifying these challenges is crucial as they can significantly impact the accuracy of the results obtained from the analysis. These issues stem from various sources and can affect the reliability and validity of the conclusions drawn from RNA-Seq experiments. This section delves into the key challenges faced during RNA-Seq data analysis, including data quality issues, biological variability, and technical limitations. Each of these components plays a vital role in shaping the practices and workflows established in the field of genomic research.
Data Quality Issues
One of the foremost challenges in RNA-Seq data analysis is ensuring high-quality data. RNA extraction and library preparation are sensitive steps that can introduce biases and artifacts. Common data quality issues include:
- Low-quality reads: Reads may contain errors that can mislead downstream analysis.
- Contamination: Samples may become contaminated with foreign RNA, affecting the expression profiles.
- Inconsistent sequencing depth: Variability in sequencing depth can lead to discrepancies in gene expression quantification.
Addressing these data quality issues involves rigorous quality control measures, such as using tools like FastQC. This ensures that the data collected is both reliable and suitable for subsequent analysis.
Biological Variability
Biological variability is another significant and inherent challenge in RNA-Seq data analysis. Living organisms exhibit variations at multiple levels, influenced by several factors such as genotype, environment, and time points during sampling. This variability can obscure the detection of true differences in gene expression. Key factors include:
- Gene expression variability: Even under controlled conditions, gene expression levels can fluctuate.
- Batch effects: Technical variations can lead to differences between samples processed at different times.
- Sample heterogeneity: Mixed cell populations can mask differences in cell-type specific expression.
To tackle biological variability, researchers can employ biological replicates and utilize statistical methods that account for such variability, ensuring a more accurate representation of the underlying biological phenomena.
Technical Limitations
The technological aspects of RNA-Seq data analysis present additional challenges. RNA-Seq technologies have advanced rapidly, but limitations still persist. Some technical limitations include:
- Sequencing platform constraints: Different platforms may produce varying results, leading to comparability issues.
- Library preparation biases: Specific methods can introduce biases in the types of RNA captured, affecting downstream analysis.
- Data interpretation difficulties: The quantitative nature of RNA-Seq data can lead to complexities in biological interpretation, especially in multimodal datasets.
Mitigating these technical challenges involves understanding the limitations of the chosen technology and adopting best practices in experimental design and data processing.
"The challenges in RNA-Seq data analysis underscore the need for meticulous planning and execution throughout the experimental process."
By recognizing and addressing these challenges, researchers can enhance the robustness and reliability of RNA-Seq data analysis, ultimately leading to more credible insights into gene expression and regulation.
Advanced Topics in RNA-Seq Analysis
Advanced topics in RNA-Seq analysis are crucial for those seeking a deeper understanding of this powerful technology. They allow researchers and practitioners to go beyond the basics, exploring new methodologies and applications. As the field of genomics evolves, these topics highlight cutting-edge trends and innovations that significantly enhance data interpretation and utility.
Single-Cell RNA Sequencing
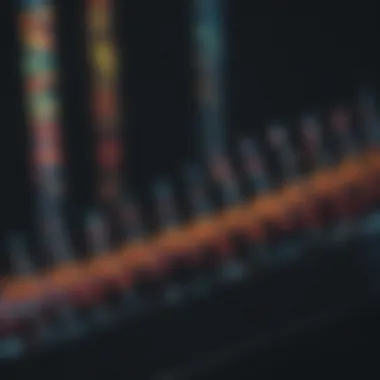
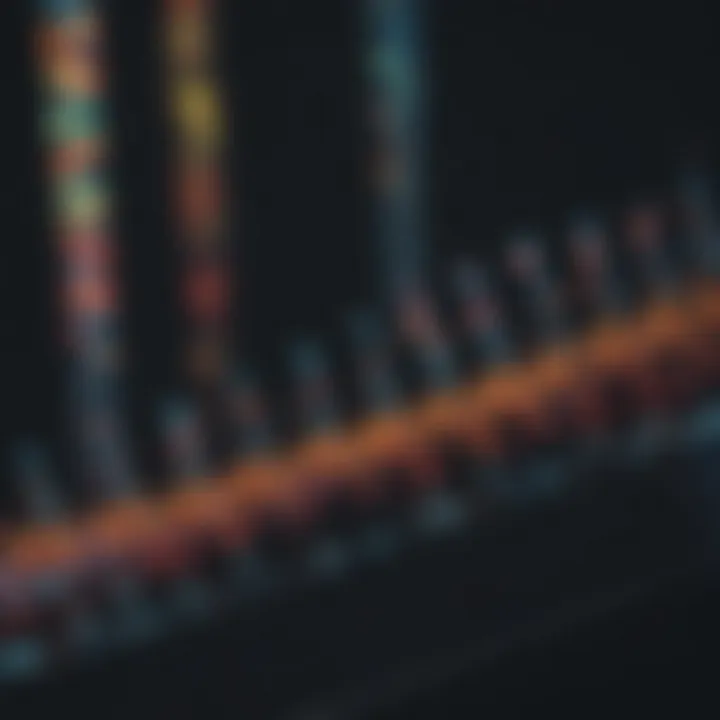
Single-cell RNA sequencing (scRNA-Seq) represents a significant advancement in RNA analysis. Traditional RNA-Seq often analyzes bulk tissue samples, which can mask heterogeneity within tissues. In contrast, scRNA-Seq isolates individual cells, enabling a detailed examination of gene expression at the single-cell level. This method is invaluable for understanding cellular diversity within a population and can reveal distinct transcriptomic profiles across different cell types.
The benefits of scRNA-Seq include:
- Resolution: Researchers can observe the expression variations between individual cells, leading to insights into rare cell types.
- Heterogeneity: This technology provides understanding of cellular responses to perturbations, which can be essential for studies in cancer, development, and immunology.
- Functional Insights: Analyzing single-cell transcriptomes can uncover regulatory networks that govern cellular behavior.
However, challenges remain with scRNA-Seq, such as:
- Data Complexity: The data generated is vast and complex, requiring sophisticated analysis tools.
- Cost: Single-cell experiments can be significantly more expensive than bulk RNA-Seq.
Institutions and researchers must adeptly navigate these challenges to fully leverage scRNA-Seq in their studies.
Integrative Genomics
Integrative genomics involves combining data from various genomic technologies to achieve a comprehensive understanding of biological systems. This approach can bring insights from RNA-Seq together with DNA sequencing, proteomics, and epigenomics. By analyzing these layers of data, researchers can better understand the regulation of gene expression and identify pathways that are altered in disease states.
Key considerations in integrative genomics include:
- Multimodal Data: The integration of diverse datasets can enrich findings but may also complicate analyses due to differences in data structure.
- Statistical Methods: Employing robust statistical methods is critical for interpreting integrated results effectively. Techniques like multi-omics analysis facilitate the identification of correlations across different levels of biological information.
- Biological Relevance: Findings must be contextualized within biological frameworks to ensure they are meaningful.
Integrative genomics opens new pathways for understanding complex biological phenomena, making it a vital area of focus for those involved in RNA-Seq data analysis.
Software Tools for RNA-Seq Analysis
RNA-Seq analysis entails several technical steps that require precision and the use of appropriate software tools. The importance of software tools in this domain cannot be overstated; they facilitate data management, processing, and the analysis of vast amounts of sequencing data. An efficient RNA-Seq workflow is often contingent upon selecting the right software that aligns with specific research goals. Students and researchers alike need to understand the nuances of these tools, not only to manage their data effectively but also to draw meaningful conclusions from their analyses.
Choosing the right software tools can enhance the sensitivity and specificity of the analysis, reduce computational time, and improve the reproducibility of results. Therefore, a comprehensive understanding of bioinformatics tools, their functions, and limitations is imperative.
Prelude to Bioinformatics Tools
Bioinformatics tools are a crucial element in RNA-Seq data analysis. These are specialized software solutions designed to handle tasks ranging from data preprocessing to statistical analysis. They allow researchers to handle the complexities associated with RNA sequencing data. Tools such as TopHat, HISAT2, and STAR are primarily used for read alignment, while DESeq2 and edgeR are popular for differential expression analysis.
Benefits of Bioinformatics Tools
- Data Handling: They provide infrastructures for managing large datasets, which is fundamental for RNA-Seq.
- Operational Efficiency: Automated pipelines can streamline workflow, allowing researchers to focus on interpretation rather than data processing.
- Enhanced Accuracy: Tools often come equipped with algorithms that enhance the precision of analyses, reducing the occurrence of false positives and negatives.
- User Support: Many tools offer extensive documentation and community forums to assist users in resolving issues.
Use of these tools requires familiarity with programming languages, especially R or Python, as these languages are commonly used to extend the functionalities of basic bioinformatics tools.
Comparative Analysis of Software Options
With a myriad of software tools available, a comparative analysis can help in selecting the best-fit options for specific RNA-Seq projects. It is important to weigh factors such as functionality, ease of use, performance, and cost when choosing software tools.
- Functionality: Some tools may focus primarily on alignment, while others may integrate features for visualization and statistical analysis.
- Ease of Use: Graphical user interfaces (GUIs) may ease the learning curve for beginners, whereas command-line tools may provide more flexibility and efficient performance for experienced users.
- Performance: Evaluating speed and accuracy can be critical, especially in large-scale projects.
- Cost: While many tools are open-source and free, some proprietary software may offer advanced functions for a price.
Here are some commonly used tools along with their features:
- HISAT2: Fast and sensitive alignment. Suitable for large datasets.
- DESeq2: Excellent for differential expression analysis with robust statistical methods.
- Cufflinks: Primarily for transcript assembly and quantification.
By evaluating these factors, researchers can make informed decisions that serve their specific analytical needs effectively.
"Selecting appropriate software tools is crucial for efficient data analysis, ultimately impacting the validity of the research outcomes."
Understanding software tools allows students and professionals to leverage the full potential of RNA-Seq data, establishing a solid foundation for accurate and reliable results.
Case Studies in RNA-Seq Data Analysis
Case studies play a crucial role in RNA-Seq data analysis by providing real-world examples that bridge the gap between theory and practical application. They illustrate how RNA-Seq technologies can be implemented in diverse biological contexts and which outcomes can be expected. The significance of case studies lies in their ability to showcase the methodology, challenges, and effective strategies for tackling RNA-Seq data analysis. They serve not only as a learning tool but also as a source of inspiration for future research.
Notably, these examples offer insights into the design of experiments, data processing techniques, and statistical analysis approaches used in actual research scenarios. Furthermore, they help in understanding the practical nuances of interpreting results in the context of biological systems. The benefits of incorporating case studies into an RNA-Seq data analysis course include enhancing comprehension and fostering critical thinking skills in students and professionals alike.
Exploration of Real-World Applications
Real-world applications of RNA-Seq are numerous and varied. One prominent case involves cancer research, where RNA-Seq has uncovered the expression profiles of tumor cells compared to normal cells. Researchers have utilized this data to identify potential biomarkers for diagnosis and prognosis. This specific application demonstrates how RNA-Seq lends itself to cancer genomics by revealing gene expression changes that correlate with disease progression.
Similarly, RNA-Seq has been used in the study of developmental biology. For instance, researchers investigating the differentiation of stem cells employ RNA-Seq to track changes in gene expression over time. This aids in understanding the complex processes involved in cell fate decisions.
Other fields also benefit from RNA-Seq, such as environmental biology. Researchers can assess how organisms adapt to changing environmental conditions by comparing RNA expression levels under various stressors.
These examples show the versatility of RNA-Seq technology and its relevance across different areas of research, solidifying its place as a vital tool in modern molecular biology.
Lessons Learned from Case Studies
The lessons derived from case studies in RNA-Seq data analysis are impactful. Many studies reveal the necessity of meticulous experimental design to ensure data quality and reproducibility. Attention to variables, sample sizes, and proper controls has demonstrated to be critical for valid outcomes.
Moreover, case studies often highlight the complexity of data interpretation. Often, results are not straightforward, as biological systems can be influenced by numerous factors. This emphasizes the importance of using robust statistical methods and tools to draw reliable conclusions from RNA-Seq data.
Additionally, the integration of findings from various studies broadens the perspective on gene function and regulatory mechanisms. By examining diverse case studies, researchers can recognize patterns that lead to novel hypotheses and experimental directions.
In summary, case studies in RNA-Seq data analysis are instrumental in informing, guiding, and enhancing the understanding of RNA-Seq applications. They provide invaluable lessons that contribute to both academic learning and practical research.
Future Directions in RNA-Seq Research
The field of RNA-Seq research is constantly evolving, driven by technological advances and an enhanced understanding of genomic complexities. Exploring future directions in this area is vital, as it reflects the potential to transform our understanding of genetics, biology, and medicine. This section outlines the emerging trends and technological innovations that will shape RNA-Seq research in the years to come.
Emerging Trends
Recent years have seen significant developments in RNA-Seq methodologies, with trends pointing towards greater accessibility, efficiency, and resolution. One such trend is the increase in single-cell RNA sequencing (scRNA-Seq), allowing researchers to examine the transcriptomic landscape at an unprecedented resolution. This approach facilitates the study of heterogeneity within tissues and provides insights into cellular pathways.
Moreover, there is a growing focus on the integration of multi-omics data. By combining RNA-Seq data with other omics technologies such as genomics, proteomics, or metabolomics, researchers can gain a comprehensive understanding of biological systems. This holistic view helps in elucidating complex molecular interactions, paving the way for more personalized medicine strategies.
Other prominent trends include the development of simplified workflow platforms tailored for educational purposes. As RNA-Seq becomes integrated into standard research practice, educational tools must evolve correspondingly to equip the upcoming generation with essential skills.
Technological Innovations
Technological innovations play a core role in shaping the future of RNA-Seq research. Improvements in sequencing technologies are leading to higher throughput and lower costs, making RNA-Seq more accessible. For example, long-read sequencing technologies, such as those developed by Pacific Biosciences and Oxford Nanopore, enable researchers to capture entire transcripts, providing a richer context for gene expression analysis.
Additionally, advancements in computational tools are essential for handling the vast amounts of data generated. For instance, bioinformatics tools that harness artificial intelligence are emerging, offering more efficient ways of analyzing complex RNA-Seq datasets. The implementation of machine learning algorithms helps in identifying patterns that may otherwise go unnoticed, enhancing our understanding of gene regulations and interactions.
"The rapid evolution of RNA-Seq technologies marks a significant shift in genomics, encouraging innovation and discovery across multiple biological disciplines."
Finally, collaborative research efforts are becoming increasingly common. Global partnerships enable the sharing of knowledge, datasets, and resources, accelerating advancements in RNA-Seq research. With a focus on open science, researchers can foster a more inclusive and productive environment, further pushing the boundaries of what RNA-Seq can achieve.
Epilogue and Summary
In concluding this article, it is important to recognize the significant role that the conclusion and summary play in consolidating the extensive insights shared throughout the course outline on RNA-Seq data analysis. This section serves as a critical synthesis of the information presented, emphasizing the importance of a structured approach to RNA-Seq analysis. By summarizing the key points discussed, readers can better grasp the flow and interconnections among various topics such as experimental design, data acquisition, and statistical methods.
One essential element of this conclusion is that it highlights the growing relevance of RNA-Seq in genomics and biotechnology. As technologies continue to evolve, RNA-Seq remains at the forefront, providing crucial insights into gene expression and regulatory mechanisms. Understanding these aspects helps researchers to design better experiments and interpret their findings more accurately.
In addition, this summary reflects the benefits of mastering RNA-Seq data analysis. Equipped with this knowledge, learners can tackle real-world problems in their respective fields. Data-driven decisions can lead to advancements in personalized medicine, agriculture, and ecological studies, among other areas.
Consider the following key aspects:
- Holistic Understanding: The course aims to foster a comprehensive grasp of RNA-Seq processes, allowing students and professionals to navigate challenges in data analysis.
- Practical Applications: Insights gained from RNA-Seq can be applied to a variety of research settings, enhancing the impact of genomic data.
- Continuous Learning: As the field advances, keeping abreast of new methodologies and technologies is vital. The summary encourages ongoing education in RNA-Seq.
This overview acts as a reminder of how diverse elements contribute to an effective RNA-Seq data analysis framework. As learners move forward in their academic or professional pursuits, the importance of these interconnected concepts cannot be understated.
Key Takeaways
- RNA-Seq is a powerful tool that offers insights into gene expression and regulation.
- A structured approach to experimental design leads to more reliable data.
- Understanding statistical methods is essential for accurate interpretation of results.
- Continuous advances in technology necessitate ongoing education and flexibility in methodologies.
- Practical applications of RNA-Seq data analysis span many fields, promoting innovation.
Final Thoughts on RNA-Seq Data Analysis
The journey through RNA-Seq data analysis shown in this article is essential for students, researchers, and professionals aiming to enhance their understanding of genomics. With its depth of knowledge, this course outline encourages a thoughtful exploration of the RNA-Seq landscape.
Ultimately, embracing the challenges and complexities of RNA-Seq analysis will yield fruitful results in research outcomes and practical applications. The importance of staying updated with emerging trends and innovations in RNA-Seq cannot be emphasized enough. As the field progresses, so too must the knowledge and skills of its practitioners. This is not merely a sign of academic diligence but an essential aspect of advancing science as a whole.