Statistical Lies: Unraveling Data Misuse
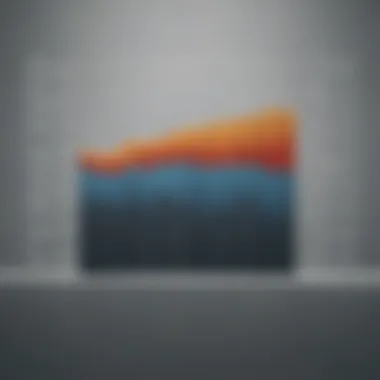
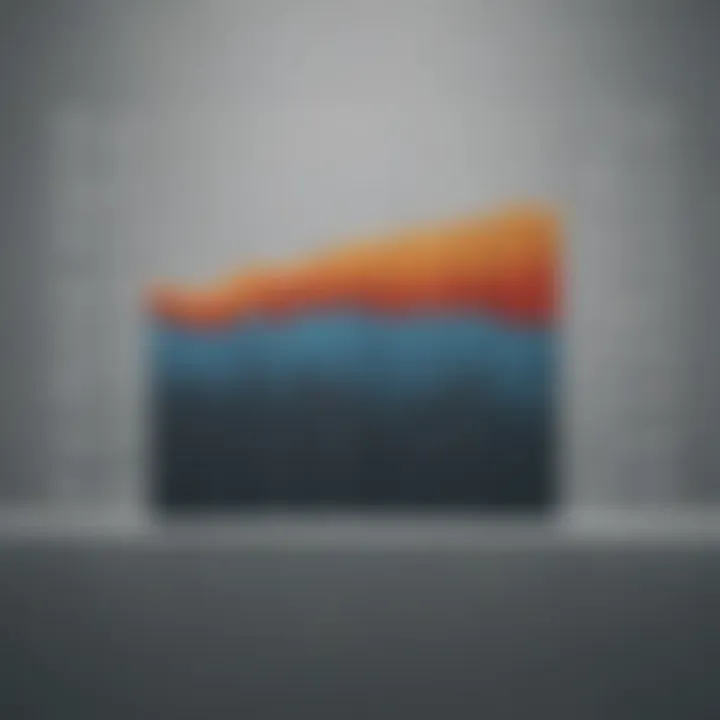
Intro
The contemporary landscape is inundated with data. Numbers and statistics often occupy central positions in discussions across various fields. However, the misuse of this data can lead to a distortion of reality. Recognizing the difference between legitimate data-driven insights and statistical misrepresentation is crucial for any informed citizen. The pervasive nature of statistical lies creates significant challenges not just for individuals, but for entire societies as they navigate complex issues.
In recent years, data manipulation has found its way into policy-making, academic publishing, and even health communication. Understanding these practices is the first step to combating them. This article will delve into numerous examples of statistical deception, outlining the importance of maintaining ethical standards and critical analytical skills.
Research Highlights
Key Findings
Statistical lies can take many forms.
- Selective Reporting: Researchers may cherry-pick data that supports pre-conceived notions, ignoring data that contradicts their findings.
- Misleading Visualizations: Graphs can be designed to exaggerate trends or misinterpret clear outcomes. For example, a truncated vertical axis can make a small difference seem monumental.
- Overgeneralization: Data can be misleading when results are extended beyond their specific context without justification.
- Rounding Errors and P-hacking: Researchers may manipulate data sets to achieve statistically significant results, often leading to false conclusions.
These forms of deception not only obscure the truth but can substantially affect public opinion and scientific integrity.
Implications and Applications
The implications of statistical manipulation are profound. They can:
- Influence public policy based on flawed assumptions.
- Challenge the credibility of scientific claims, making it difficult for practitioners to trust new research.
- Create a society that is skeptical of genuine data points, leading to a more misinformed populace.
To combat these issues, promoting critical thinking and statistical literacy is essential. A populace equipped with these skills is better prepared to evaluate the integrity of data presented to them.
Methodology Overview
Research Design
For this article, an extensive review of literature concerning statistical lies was conducted. Sources included peer-reviewed journals, scholarly articles, reputable news media, and discussions on platforms like Reddit and Facebook. This mixed-methods approach offered a broad perspective on how statistical deceit manifests in various contexts.
Experimental Procedures
The research followed systematic steps:
- Data Collection: Identified instances of statistical manipulation in recent articles and studies.
- Analysis: Categorized the forms of deception and examined their context and impact.
- Case Studies: Selected specific examples to illustrate the effects of statistical lies.
- Recommendations: Developed strategies to improve statistical literacy among students, researchers, and professionals.
"Statistical deception is not merely an academic issue; it fundamentally undermines informed decision-making in society."
Understanding Statistical Lies
The manipulation of data has become prevalent in modern discourse. Awareness of this issue is crucial for anyone engaged in academic or professional fields where data plays a key role. Understanding statistical lies enables individuals to critically assess the information presented in debates, reports, and studies. This not only assists in identifying misleading tactics but also empowers informed decision-making.
Statistical lies can manifest in various forms, and recognizing these is vital for evaluating claims effectively. Misleading statistics can shape public opinion, influence policy decisions, and alter perceptions in significant ways. Therefore, comprehending how statistical information is misused imparts the ability to challenge erroneous assertions and refine one’s analytical skills.
Definitions and Key Concepts
To grasp statistical lies, it is necessary to define core terms. Statistics refers to the collection, analysis, and interpretation of numerical data. Lies in this context imply intentional misrepresentation or deceptive practices aimed at misleading others. Together, statistical lies involve presenting data in a way that distorts reality, often with the objective of supporting a specific agenda.
Key concepts include:
- Bias: A systematic error that distorts results.
- Correlation vs Causation: Confusing these terms can lead to faulty conclusions.
- Statistical Significance: Misinterpreting this can amplify misleads.
- Sampling Methods: Poor sampling can skew results.
Understanding these distinctions is essential for identifying statistical manipulation.
Historical Context
The misuse of statistics is not a recent phenomenon. Historically, political regimes have employed deceptive statistics to justify policies or mislead the populace. One prominent example is the use of fabricated economic data in the Soviet Union, which created an illusion of growth and prosperity that did not exist.
In contemporary times, statistical manipulation remains prevalent in the media and political landscapes. The evolution of data collection methods, such as surveys and polls, has transformed how information is garnered and interpreted. With the rise of the internet, the accessibility of data has both democratized access to information and introduced new challenges regarding its accuracy. Reports can spread rapidly, and once misinformation circulates, it is often difficult to correct.
By considering this historical backdrop, one can appreciate the enduring relevance of statistical integrity. A clear awareness of past abuses fosters critical engagement with current data, encouraging diligence in evaluation.
Types of Statistical Lies
The types of statistical lies are essential to understand as they form the basis of this article. Recognizing these lies empowers readers to critically assess data presented in various contexts. It reveals how statistics can be warped to mislead the audience, impacting decision-making, policy formation, and public perception. Understanding these types allows for a deeper discussion of their implications and encourages a culture of accountability among data practitioners.
Selective Reporting
Selective reporting is a common technique used to shape narratives around data. When information is cherry-picked, it forms a skewed picture that may align with certain agendas while ignoring the broader context. This method is particularly prevalent in media and political communication, where only favorable statistics are highlighted.
Cherry-Picking Data
Cherry-picking data involves selecting specific pieces of information to support a claim while disregarding contradictory evidence. This tactic is powerful because it allows the presenter to craft a compelling argument. Its key characteristic is the focus on data that aligns with the desired outcome. Cherry-picking is a popular strategy in articles and presentations as it is easy to implement and can produce convincing arguments.
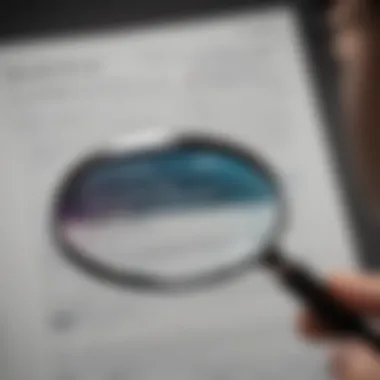
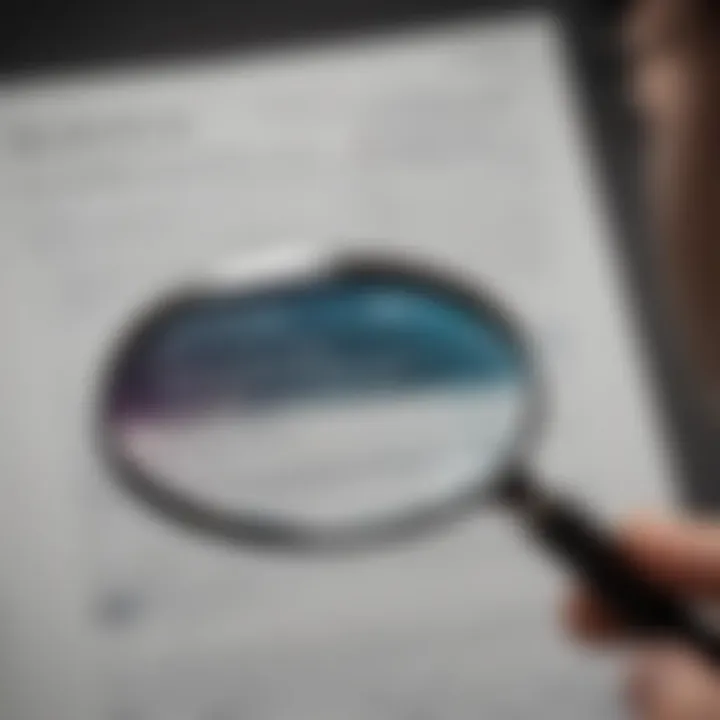
However, this approach has significant disadvantages. It can lead to misleading conclusions and betray the complexity of the bigger picture. The unique feature of cherry-picking data is that it often appeals to emotional responses, making it an effective, albeit flawed, choice for persuasion.
Suppressing Counter-Evidence
Suppressing counter-evidence is another effective form of selective reporting. This entails omitting relevant data that could challenge the narrative being presented. A key characteristic here is the deliberate act of ignoring substantial information, which may not be favorable. It serves as a beneficial tactic for those wishing to uphold a specific viewpoint while undermining credibility.
The unique aspect of suppressing counter-evidence is that it distorts the overall understanding of an issue. On one hand, it can create a compelling narrative; on the other, it can diminish the integrity of the data presented. This tactic ultimately undermines informed decision-making.
Misleading Visualizations
Misleading visualizations directly distort the comprehension of data represented graphically. This manipulation can involve anything from scale adjustments to color choices, making it a crucial topic in discussing types of statistical lies.
Graphical Distortion Techniques
Graphical distortion techniques involve presenting data in a way that misleads the viewer about the actual relationships or magnitudes of the data points. A prevalent characteristic is the manipulation of axes or proportions in charts and graphs. This approach is favorable because it can highlight certain trends while masking others.
The unique feature here is that graphical distortions often go unnoticed by a lay audience, thus making it an effective, if deceptive, method. While it can be engaging, it diminishes the reliability of information and can severely warp public understanding.
Color Usage and Its Impact
Color usage in data visualization can significantly influence how information is perceived. Different colors can evoke emotions, drawing attention to specific data points. A key characteristic of this technique is its psychological impact on interpretation. Using colors effectively can serve to highlight particular trends or urgencies, making it an excellent tool for persuasion.
However, the disadvantage is that it can lead to biased interpretations of the data. Color choices can be manipulated to mislead viewers about the severity or importance of certain data points. Thus, color application requires careful consideration to promote clarity rather than confusion.
Confusing Correlation with Causation
Confusing correlation with causation is a prevalent statistical error that often leads to significant misinterpretations. Recognizing this mistake is vital to evaluating data accurately.
Examples of Common Misinterpretations
Common misinterpretations often arise from observing relationships between two variables and erroneously inferring that one causes the other. A key characteristic here is the oversimplification of complex phenomena. This is popular in casual discussions about data, where anecdotal evidence can reinforce flawed reasoning.
The unique feature of these examples is their tendency to oversimplify reality. Misinterpretations often resonate with people due to their straightforward nature. Yet, they also lead to flawed conclusions and decision-making when critical analysis is lacking.
Statistical Spuriousness
Statistical spuriousness involves situations where a correlation exists due to a third variable rather than a direct relationship. A critical characteristic is the underlying complexity of such relationships, making them easy to overlook. Spuriousness is a beneficial concept to discuss because it reveals the depths of statistical analysis.
The primary disadvantage of spuriousness lies in its subtlety. It often requires sophisticated understanding to identify such inconsistencies. Yet, failing to recognize it can lead to erroneous policies and reactive measures based on false assumptions.
Understanding the various types of statistical lies can empower individuals to decode the complex world of data, ensuring a more informed perspective.
The Role of Bias in Data Interpretation
Bias plays a critical role in how data is interpreted and presented. Understanding biases helps clarify why certain data may be skewed or misrepresented. Bias can infiltrate every stage of the data process, from collection to interpretation and representation, often shaping conclusions and guiding decisions based on tainted evidence. In a world swamped with numbers and statistics, recognizing these biases is crucial for informed decision-making.
Cognitive Biases in Decision Making
Cognitive biases are systematic patterns of deviation from norm or rationality in judgment. They influence how individuals interpret data and make decisions. Two key cognitive biases are confirmation bias and the anchoring effect.
Confirmation Bias
Confirmation bias refers to the tendency to search for, interpret, and remember information in a way that confirms one’s prior beliefs. This bias is prevalent in many domains, particularly around contentious issues like health or politics. One significant aspect of confirmation bias lies in its reinforcement of already held beliefs, which can lead to a narrow perspective. This characteristic makes it a common barrier in discussions about data interpretation.
The unique feature of confirmation bias is that it often results in overlooking data that contradicts one's view. While this may provide short-term reassurance, it poses disadvantages in the long run as it can distort an objective understanding of the reality presented by the data.
"Acknowledging confirmation bias is essential for any critical analysis of data. It fosters an environment where evidence can be surpassed by personal belief."
Anchoring Effect
The anchoring effect occurs when individuals rely too heavily on the first piece of information they receive (the 'anchor') when making decisions. This biased reliance can significantly impact how statistics are perceived. For instance, if a report highlights a shocking statistic, that number can serve as an anchor, influencing how subsequent data is interpreted and valued.
A notable characteristic of the anchoring effect is its ability to skew judgments based on initial impressions. This bias enhances the importance of first encounters with data, which can lead to disproportionate emphasis on specific figures. This can be especially problematic in reports or presentations where the first piece of data presented sets an undesirable precedent for future interpretations.
Institutional and Personal Bias
Bias is not only a matter of individual perception; it can also be embedded in institutions. Institutional bias can arise from funding sources or organizational culture, while personal bias stems from individual beliefs and experiences. Two common forms of institutional and personal biases are funding bias and opinion bias.
Funding Bias
Funding bias refers to the influence that funding sources can have on research outcomes. Often, research funded by organizations has results that favor the interests of the sponsors. This aspect of funding bias highlights the importance of scrutinizing the origins of financial support in research studies.
Unique to funding bias is the challenge it poses to the credibility of research. When a study's funding source is aligned with certain interests, one must question the validity of the findings. The advantage here is that awareness of funding bias encourages transparency and promotes critical examination of research.
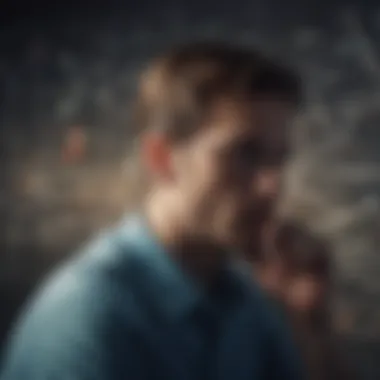
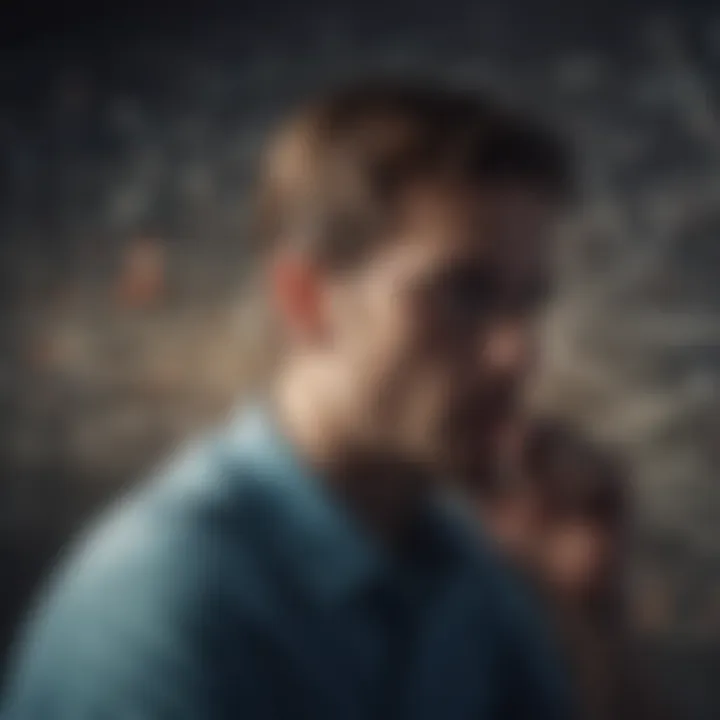
Opinion Bias
Opinion bias relates to the influence of personal views on the interpretation of data. Individuals often let their beliefs interfere with how they assess information, which can lead to skewed conclusions. This facet of bias emphasizes the subjective nature of human reasoning. Personal opinion can shape and distort the acceptance of statistical data and findings.
The unique feature of opinion bias is its capacity to create echo chambers where similar beliefs proliferate. This is beneficial in fostering community discussions, but it can also disadvantage the objective analysis of broader data trends.
Understanding the role of these biases is essential in unraveling the complexities of data interpretation. By being aware of how biases manifest at both the cognitive and institutional levels, individuals can better assess statistical claims and make more informed decisions.
Statistical Lies in Modern Discourse
The current landscape of communication is heavily influenced by data. Statistics are often seen as the backbone of factual claims in many discussions. However, the misuse of data can lead to significant misinformation. Statistical lies shape opinions, influence decision-making, and can alter the course of policies. This section will elaborate on two crucial aspects: media and political manipulation, which are pervasive in modern discourse.
Media Influence
Sensationalism in Reporting
In today's fast-paced world, the media often resorts to sensationalism to capture attention. Sensationalism refers to the use of shocking or exaggerated claims to arouse public interest. This practice has a direct impact on how statistical information is presented. For instance, when news outlets focus on dramatic headlines rather than factual data, they risk misrepresenting the essence of the statistics.
This approach is often popular due to its effectiveness in grabbing headlines and boosting ratings. A key characteristic of sensationalism is its ability to oversimplify complex issues. By making data seem more alarming or significant than it is, sensationalism can lead the public to misconceptions.
However, this can have disadvantages. Such a strategy may create panic or confusion. The audience may take the sensational figures at face value, leading to distorted perceptions of reality. It is crucial for readers to engage critically with these representations to discern what data truly indicates.
Statistical Misrepresentation in News
Statistical misrepresentation occurs when data is presented in a way that misleads or misinforms the audience. News articles sometimes distort statistics to fit a narrative. This can involve selective reporting, where only certain facts are highlighted while others are omitted. It can also involve manipulating visualizations that might confuse the audience.
This practice is concerning because it undermines the credibility of news sources. A primary characteristic is the lack of transparency in how statistics are gathered and presented. It is a common choice for outlets seeking higher viewership or clicks, opting for catchy yet misleading claims.
While this creates engaging content, the disadvantage lies in eroding trust in media. When readers realize that statistics in news are often not what they seem, they become skeptical of all data presented. This skepticism can create a feedback loop of distrust, making it difficult for reputable news sources to convey essential information accurately.
Political Manipulation
Statistical Arguments in Campaigns
In politics, numbers play a critical role. Statistical arguments often become tools for persuasion during campaigns. Politicians may use selective statistics to bolster their positions or undermine their opponents. For instance, citing favorable economic data can create a narrative of success, regardless of the broader context.
A significant characteristic of this approach is the power it wields over public opinion. If voters see favorable statistics, they may be more likely to support a candidate. This makes it a beneficial tactic for those campaigning. However, the reliance on selective statistics can also be a double-edged sword.
The unique feature here is the potential for backlash. If voters discover that misleading statistics are used, it can damage a political figure's credibility. Rational voters want transparency; therefore, if they feel manipulated, the long-term consequences can be detrimental to a campaign.
Policy Implications of Misleading Data
Misleading data doesn't just affect elections; it influences policy decisions as well. When decision-makers rely on erroneous statistics, the ramifications can ripple through society. It can lead to poor policy choices that impact health care, education, and economic structures.
A key characteristic of this issue is the complexity of data interpretation in policy-making. Misleading data can result in ineffective or damaging policies. This is a widespread choice for stakeholders looking to support their agenda, regardless of the broader repercussions of their claims.
However, the disadvantages are far-reaching. Poorly informed policy decisions can lead to social unrest or increased public dissatisfaction. As citizens become aware of the discrepancies between claims and reality, trust in institutions may diminish, culminating in a skeptical populace that questions data across the board.
In summary, statistical lies in modern discourse pose significant challenges. Understanding how the media and politics manipulate data is essential. Engaging critically with statistical claims can empower individuals to discern truths from lies in an information-saturated environment.
Case Studies of Statistical Lies
Case studies provide tangible examples of how statistical lies manifest in real-world scenarios. They offer insights that help demystify the reasons behind data manipulation. Each case serves as a critical examination of statistical practices and highlights the consequences of misleading information in public discourse. Understanding these examples is essential for fostering a culture of transparency and critical thinking in society.
One must consider various implications when analyzing these case studies, such as the effect on public perception and policy formation. This analysis is beneficial as it strengthens arguments for statistical literacy and education in interpreting data accurately. Moreover, these examples reveal the need for vigilance in data consumption and interpretation, emphasizing the importance of questioning source credibility.
Health Statistics and Public Health
Vaccination Rates and Misleading Trends
Vaccination rates are often represented in a way that can distort public understanding. In some instances, data cherry-picking occurs, where only favorable statistics are highlighted. This affects the overall image of vaccination campaigns and can fuel vaccine hesitancy. The key characteristic of vaccination rate statistics is their direct influence on public health decisions.
By presenting trends without context, it complicates the public’s ability to make informed health choices. The unique feature here lies in how these statistics can vary by demographics, geography, or time frame. Misleading representation can undermine trust in necessary public health interventions, a disadvantage that poses serious outcomes in managing vaccine-preventable diseases.
Healthcare Outcomes and Misrepresentation
Healthcare outcomes are frequently reported in misleading ways, altering public perception of healthcare efficacy. For example, selectively reported data on treatment successes may omit cases where treatments fail or lead to complications. This selective presentation is a critical aspect of the broader narrative surrounding healthcare quality.
The key characteristic of this misrepresentation is that it shapes public expectation and trust in health systems. The unique feature focuses on outcome statistics, which can fluctuate significantly based on reporting criteria. Misrepresentation may result in misguided public policies or healthcare practices, ultimately disadvantaging patients and undermining the integrity of healthcare systems.
Economic Data Manipulations
Unemployment Rates and Their Interpretation
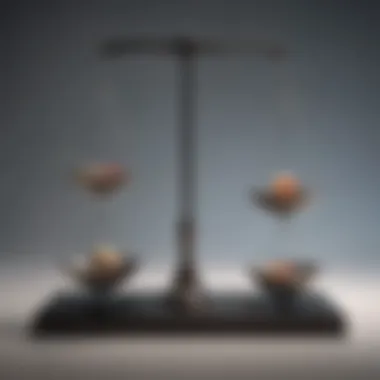
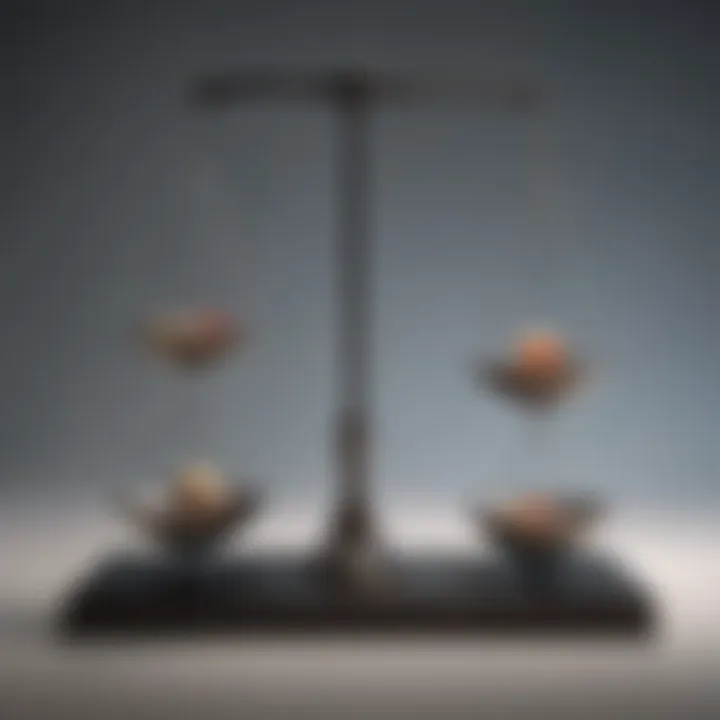
Unemployment rates represent a vital economic indicator but can easily become misinterpreted. Politicians might highlight the drop in numbers without explaining the change in measurement criteria or definitions, leading to public misunderstanding. The key characteristic is the complexity of how unemployment is quantified; raw numbers alone can be misleading.
Often, the unique feature of this statistic may hide underemployment levels or those who leave the labor force entirely. This manipulation could create a false sense of economic security which detracts from addressing real economic issues.
GDP Growth Statistics
Gross Domestic Product (GDP) growth statistics are routinely utilized to demonstrate economic prosperity. However, GDP is often criticized for not accounting for income inequality or environmental degradation. The key characteristic lies in its inability to fully represent the economy's health. This statistic often serves as a popular choice in political messaging, oversimplifying economic realities.
The unique feature of GDP growth statistics is their widespread acceptance in evaluating national prosperity. While these figures can be beneficial for tracking economic trends, the disadvantages include a potential lack of transparency and understanding of what they do not reveal. Public discourse based on GDP figures can subsequently misinform citizen perspectives on economic success or failure.
Combating Statistical Lies
The prevalence of statistical manipulation necessitates a concerted effort to combat these deceptive practices. Combating statistical lies is critical to ensuring that data is presented accurately and interpreted correctly. By addressing the misuse of data, we can protect the integrity of scientific research, sway the discourse towards evidence-based arguments, and ultimately support a knowledgeable public. Awareness of how statistics can be distorted enables individuals and organizations to navigate complex data landscapes more effectively, fostering a more informed society.
Promoting Statistical Literacy
Educational Initiatives
Educational initiatives play a vital role in promoting statistical literacy among various audiences. These programs aim to help individuals understand statistical concepts and methodologies. By integrating statistics into the curriculum, educators can equip students with the tools necessary to analyze and critique data. A key characteristic of educational initiatives is their focus on practical applications. This hands-on approach makes them an effective choice in combatting statistical lies. For example, incorporating case studies that illustrate statistical misrepresentation can enhance students’ critical thinking skills.
Moreover, educational initiatives often include workshops and online courses. These unique features allow for greater accessibility, enabling a wider audience to benefit from such education. However, there can be challenges in reaching individuals who do not see statistics as relevant to their lives.
Public Awareness Campaigns
Public awareness campaigns are essential for informing the general public about the risks of statistical deception. These campaigns highlight the importance of questioning data sources and understanding the context in which statistics are presented. A significant characteristic of public awareness campaigns is their broad outreach, often utilizing social media to disseminate information widely. This makes them effective, as they can engage diverse audiences.
Unique features of these campaigns include infographics and visual tools that simplify complex concepts. Through these means, they create more engaging content that captures attention while educating at the same time. Nonetheless, the effectiveness can vary based on the target demographic and their previous exposure to statistical concepts.
Encouraging Critical Thinking
Encouraging critical thinking is fundamental in equipping individuals to assess data critically. This skill allows people to differentiate between credible statistics and misleading figures. A key aspect of this approach is focusing on the evaluation of sources and data. By understanding how to identify trustworthy sources, individuals can better navigate the often tumultuous sea of information.
Evaluating Sources and Data
Evaluating sources and data involves analyzing the reliability and validity of statistical information. This practice contributes significantly to the overall goal of combating statistical lies, as it fosters a discerning mindset. A notable characteristic is the emphasis on rigorous criteria for source evaluation. This provides individuals with a beneficial framework for assessing the credibility of the statistics they encounter.
The unique feature of this approach includes training programs that focus on identifying red flags in data reporting. These programs can help raise awareness of common pitfalls in statistical interpretation. However, challenges may arise from pre-existing biases that affect how individuals assess the credibility of various sources.
Understanding Research Methodology
Understanding research methodology is crucial for interpreting statistical findings accurately. This aspect allows individuals to critically assess how data is collected and analyzed. A beneficial characteristic is the clarity with which research designs are explained, making it accessible for non-experts. This fosters a deeper understanding of how conclusions are drawn from data.
Unique features of this understanding include workshops and seminars that emphasize real-world applications. By showing how methodology impacts results, these initiatives encourage a more nuanced view of statistics. Nevertheless, complexities in research methodology can still pose obstacles for laypersons who seek to understand it fully.
"Statistical literacy is like a shield against misinformation in an era dominated by data."
In summary, combating statistical lies requires a multi-faceted approach involving education, awareness, and critical thinking. Each strategy plays a vital role in creating a society that can discern the truth within the statistics presented to it.
The Future of Statistics in Society
The increasing reliance on data in various spheres of life heightens the relevance of statistics. As society progresses, the future of statistics will be shaped significantly by technological advancements, ethical practices, and the pervasive nature of data in discourse. A comprehensive understanding of how these elements intertwine will reveal pathways to enhance data integrity and public trust.
Technological Advancements and Their Impact
Big Data Analytics
Big Data Analytics focuses on analyzing large and complex data sets to uncover patterns, correlations, and insights. This contributes crucially to understanding big phenomena in real-time environments. One of the key characteristics of Big Data Analytics is its ability to manage vast quantities of unstructured data effectively. This capability makes it a popular choice because it empowers organizations to make data-driven decisions at unprecedented speeds.
A unique feature of Big Data Analytics is its application of predictive analytics, which allows entities to anticipate future trends based on historical data. However, it comes with concerns about privacy and data security. As organizations gain significant insights, there is an inherent responsibility to handle that data ethically, balancing benefits with risks.
AI and Machine Learning in Data Interpretation
AI and Machine Learning provide dynamic tools for data interpretation by automating complex analysis processes. These technologies contribute prominently by allowing machines to learn from past data and improve over time. A notable characteristic of AI and Machine Learning is their ability to recognize patterns that are not evident to human analysts. This makes them beneficial in extracting actionable insights and enhancing decision-making frameworks in sectors like healthcare, finance, and marketing.
However, the use of AI and Machine Learning comes with unique challenges. They can perpetuate biases present in the training data, leading to skewed results. Ensuring that these tools are used responsibly is vital to maintaining trust in statistical outputs. As the landscape evolves, experts must prioritize algorithmic fairness and transparency to address these challenges.
The Ethical Responsibility of Data Practitioners
Transparency in Data Reporting
Transparency in Data Reporting is crucial as it fosters trust between data providers and users. This aspect is significant for creating reliability in the statistics presented. The essential characteristic of transparency is openness about data collection methods, analytical approaches, and potential biases. By maintaining this clarity, organizations can promote confidence in their findings, which is particularly vital in public discourse.
A unique feature of transparency is its empowering effect on consumers of data. With accessible information, stakeholders can engage more critically with data interpretations. However, the challenge lies in ensuring the information is adequately detailed without overwhelming non-expert audiences.
Accountability for Misrepresentation
Accountability for Misrepresentation emphasizes the need for responsible data practices. This concept is integral to the ethical framework governing data usage and presentation. The key characteristic of accountability involves holding individuals and organizations responsible for erroneous or misleading data dissemination. This is crucial in maintaining the integrity of statistical information across various sectors.
A distinctive feature is the potential for established guidelines and standards to guide ethical practices in data reporting. Organizations must embody these principles to encourage responsible behaviors. However, enforcing accountability can be complex due to the multifaceted nature of data interpretation and the divergent agendas present in many fields.